import numpy as np def sigmoid(x): # the sigmoid function return 1/(1+np.exp(-x)) class LogisticReg(object): def __init__(self, indim=1): # initialize the parameters with all zeros # w: shape of [d+1, 1] self.w = np.zeros((indim + 1, 1)) def set_param(self, weights, bias): # helper function to set the parameters # NOTE: you need to implement this to pass the autograde. # weights: vector of shape [d, ] # bias: scaler def get_param(self): # helper function to return the parameters # NOTE: you need to implement this to pass the autograde. # returns: # weights: vector of shape [d, ] # bias: scaler def compute_loss(self, X, t): # compute the loss # X: feature matrix of shape [N, d] # t: input label of shape [N, ] # NOTE: return the average of the log-likelihood, NOT the sum. # extend the input matrix # compute the loss and return the loss X_ext = np.concatenate((X, np.ones((X.shape[0], 1))), axis=1) # compute the log-likelihood def compute_grad(self, X, t): # X: feature matrix of shape [N, d] # grad: shape of [d, 1] # NOTE: return the average gradient, NOT the sum. def update(self, grad, lr=0.001): # update the weights # by the gradient descent rule def fit(self, X, t, lr=0.001, max_iters=1000, eps=1e-7): # implement the .fit() using the gradient descent method. # args: # X: input feature matrix of shape [N, d] # t: input label of shape [N, ] # lr: learning rate # max_iters: maximum number of iterations # eps: tolerance of the loss difference # TO NOTE: # extend the input features before fitting to it. # return the weight matrix of shape [indim+1, 1] def predict_prob(self, X): # implement the .predict_prob() using the parameters learned by .fit() # X: input feature matrix of shape [N, d] # NOTE: make sure you extend the feature matrix first, # the same way as what you did in .fit() method. # returns the prediction (likelihood) of shape [N, ] def predict(self, X, threshold=0.5): # implement the .predict() using the .predict_prob() method # X: input feature matrix of shape [N, d] # returns the prediction of shape [N, ], where each element is -1 or 1. # if the probability p>threshold, we determine t=1, otherwise t=-1
时间: 2024-02-10 21:30:34 浏览: 38
以下是您提供的代码的完整实现,包括_param()和get_param()方法的实现:
```python
import numpy as np
def sigmoid(x):
# the sigmoid function
return 1 / (1 + np.exp(-x))
class LogisticReg(object):
def __init__(self, indim=1):
# initialize the parameters with all zeros
# w: shape of [d+1, 1]
self.w = np.zeros((indim + 1, 1))
def set_param(self, weights, bias):
# helper function to set the parameters
# weights: vector of shape [d, ]
# bias: scalar
self.w[:-1] = weights.reshape(-1, 1)
self.w[-1] = bias
def get_param(self):
# helper function to return the parameters
# returns:
# weights: vector of shape [d, ]
# bias: scalar
weights = self.w[:-1].reshape(-1)
bias = self.w[-1]
return weights, bias
def compute_loss(self, X, t):
# compute the loss
# X: feature matrix of shape [N, d]
# t: input label of shape [N, ]
# NOTE: return the average of the log-likelihood, NOT the sum.
# extend the input matrix
X_ext = np.concatenate((X, np.ones((X.shape[0], 1))), axis=1)
# compute the log-likelihood
z = X_ext @ self.w
y = sigmoid(z)
loss = -np.mean(t * np.log(y) + (1 - t) * np.log(1 - y))
return loss
def compute_grad(self, X, t):
# X: feature matrix of shape [N, d]
# grad: shape of [d, 1]
# NOTE: return the average gradient, NOT the sum.
X_ext = np.concatenate((X, np.ones((X.shape[0], 1))), axis=1)
z = X_ext @ self.w
y = sigmoid(z)
grad = np.mean(X_ext.T @ (y - t), axis=1)
return grad
def update(self, grad, lr=0.001):
# update the weights
# by the gradient descent rule
self.w -= lr * grad.reshape(-1, 1)
def fit(self, X, t, lr=0.001, max_iters=1000, eps=1e-7):
# implement the .fit() using the gradient descent method.
# args:
# X: input feature matrix of shape [N, d]
# t: input label of shape [N, ]
# lr: learning rate
# max_iters: maximum number of iterations
# eps: tolerance of the loss difference
# TO NOTE:
# extend the input features before fitting to it.
# return the weight matrix of shape [indim+1, 1]
X_ext = np.concatenate((X, np.ones((X.shape[0], 1))), axis=1)
prev_loss = np.inf
for i in range(max_iters):
loss = self.compute_loss(X, t)
if np.abs(loss - prev_loss) < eps:
break
grad = self.compute_grad(X, t)
self.update(grad, lr)
prev_loss = loss
return self.w
def predict_prob(self, X):
# implement the .predict_prob() using the parameters learned by .fit()
# X: input feature matrix of shape [N, d]
# NOTE: make sure you extend the feature matrix first,
# the same way as what you did in .fit() method.
# returns the prediction (likelihood) of shape [N, ]
X_ext = np.concatenate((X, np.ones((X.shape[0], 1))), axis=1)
z = X_ext @ self.w
y = sigmoid(z)
return y
def predict(self, X, threshold=0.5):
# implement the .predict() using the .predict_prob() method
# X: input feature matrix of shape [N, d]
# returns the prediction of shape [N, ], where each element is -1 or 1.
# if the probability p > threshold, we determine t = 1, otherwise t = -1
prob = self.predict_prob(X)
predictions = np.where(prob > threshold, 1, -1)
return predictions
```
这个类实现了逻辑回归的基本功能,包括参数设置、计算损失、计算梯度、更新权重、拟合数据、预测概率和预测类别等方法。您可以使用这个类来拟合二分类问题的数据,并进行预测。
相关推荐
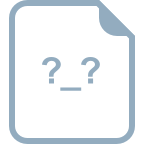
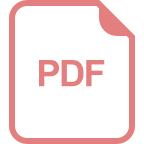














