import cv2 # 加载图片列表 img_list = ['打印1.png', '打印2.png', 'img111.png'] img_index = 0 # 创建窗口并显示第一张图片 cv2.namedWindow('image') cv2.imshow('image', cv2.imread(img_list[img_index])) # 定义按钮回调函数 def next_image_callback(event, x, y, flags, param): global img_index img_index = (img_index + 1) % len(img_list) cv2.imshow('image', cv2.imread(img_list[img_index])) # 创建按钮并绑定回调函数 cv2.createButton('Next', next_image_callback) # 进入主循环 while True: key = cv2.waitKey() if key == 27: # 按下ESC键退出 break cv2.destroyAllWindows()显示找不到图片
时间: 2024-03-11 19:50:38 浏览: 136
这个问题通常是因为程序找不到指定的图片文件导致的。
请确保你的图片文件确实存在,且路径正确。你可以尝试使用绝对路径或者相对路径来指定图片文件的路径。例如,如果你的图片文件位于当前工作目录下的 `images` 子目录中,那么可以使用如下代码来加载图片:
```python
img_list = ['./images/打印1.png', './images/打印2.png', './images/img111.png']
```
如果你不确定图片文件的路径是否正确,可以使用 `os.path.exists` 函数来检查文件是否存在。例如:
```python
import os
for img_path in img_list:
if not os.path.exists(img_path):
print(f'File not found: {img_path}')
```
这段代码会逐一检查图片文件是否存在,如果某个文件不存在,则输出错误信息。你可以根据输出的错误信息来找出问题所在,并进行修复。
相关问题
import cv2 import numpy as np def retinex(img, sigma_list): retinex = np.zeros_like(img) for sigma in sigma_list: img_blur = cv2.GaussianBlur(img, (0, 0), sigma) img_blur = np.where(img_blur == 0, 0.1, img_blur) retinex += np.log10(img) - np.log10(img_blur) retinex = retinex / len(sigma_list) retinex = np.where(retinex < 0, 0, retinex) retinex = np.where(retinex > 1, 1, retinex) return retinex def enhance_image(img): hsi = cv2.cvtColor(img, cv2.COLOR_BGR2HSV) h, s, i = cv2.split(hsi) i_enhanced = retinex(i.astype(np.float64), [15, 80, 250]) i_enhanced = (i_enhanced * 255).astype(np.uint8) s_enhanced = cv2.equalizeHist(s) hsi_enhanced = cv2.merge([h, s_enhanced, i_enhanced]) enhanced_img = cv2.cvtColor(hsi_enhanced, cv2.COLOR_HSV2BGR) return enhanced_img img = cv2.imread('low_illumination_1.png') enhanced_img = enhance_image(img) cv2.imshow('Enhanced Image', enhanced_img) cv2.waitKey(0) cv2.destroyAllWindows()
这是一段基于Retinex算法对图像进行增强的Python代码。Retinex算法是一种用于图像增强的经典算法,它可以增强图像的对比度和颜色鲜艳度,同时可以保留图像的细节信息。该算法的基本思想是将图像分解为多个尺度的高斯模糊图像,然后通过计算不同尺度的高斯模糊图像与原始图像之间的差异来获得图像的反射性和色彩性信息。在这段代码中,首先将输入图像转换为HSI颜色空间,然后对亮度通道进行Retinex增强处理,对饱和度通道进行直方图均衡化处理,最后将三个通道重新组合成BGR颜色空间的图像输出。
import os import random import numpy as np import cv2 import keras from create_unet import create_model img_path = 'data_enh/img' mask_path = 'data_enh/mask' # 训练集与测试集的切分 img_files = np.array(os.listdir(img_path)) data_num = len(img_files) train_num = int(data_num * 0.8) train_ind = random.sample(range(data_num), train_num) test_ind = list(set(range(data_num)) - set(train_ind)) train_ind = np.array(train_ind) test_ind = np.array(test_ind) train_img = img_files[train_ind] # 训练的数据 test_img = img_files[test_ind] # 测试的数据 def get_mask_name(img_name): mask = [] for i in img_name: mask_name = i.replace('.jpg', '.png') mask.append(mask_name) return np.array(mask) train_mask = get_mask_name(train_img) test_msak = get_mask_name(test_img) def generator(img, mask, batch_size): num = len(img) while True: IMG = [] MASK = [] for i in range(batch_size): index = np.random.choice(num) img_name = img[index] mask_name = mask[index] img_temp = os.path.join(img_path, img_name) mask_temp = os.path.join(mask_path, mask_name) temp_img = cv2.imread(img_temp) temp_mask = cv2.imread(mask_temp, 0)/255 temp_mask = np.reshape(temp_mask, [256, 256, 1]) IMG.append(temp_img) MASK.append(temp_mask) IMG = np.array(IMG) MASK = np.array(MASK) yield IMG, MASK # train_data = generator(train_img, train_mask, 32) # temp_data = train_data.__next__() # 计算dice系数 def dice_coef(y_true, y_pred): y_true_f = keras.backend.flatten(y_true) y_pred_f = keras.backend.flatten(y_pred) intersection = keras.backend.sum(y_true_f * y_pred_f) area_true = keras.backend.sum(y_true_f * y_true_f) area_pred = keras.backend.sum(y_pred_f * y_pred_f) dice = (2 * intersection + 1)/(area_true + area_pred + 1) return dice # 自定义损失函数,dice_loss def dice_coef_loss(y_true, y_pred): return 1 - dice_coef(y_true, y_pred) # 模型的创建 model = create_model() # 模型的编译 model.compile(optimizer='Adam', loss=dice_coef_loss, metrics=[dice_coef]) # 模型的训练 history = model.fit_generator(generator(train_img, train_mask, 4), steps_per_epoch=100, epochs=10, validation_data=generator(test_img, test_msak, 4), validation_steps=4 ) # 模型的保存 model.save('unet_model.h5') # 模型的读取 model = keras.models.load_model('unet_model.h5', custom_objects={'dice_coef_loss': dice_coef_loss, 'dice_coef': dice_coef}) # 获取测试数据 test_generator = generator(test_img, test_msak, 32) img, mask = test_generator.__next__() # 模型的测试 model.evaluate(img, mask) # [0.11458712816238403, 0.885412871837616] 94%
上面这段代码是在导入一些库。它导入了 OS 库,Random 库,NumPy 库,CV2 库,Keras 库,以及一个叫做 Create_unet 的自定义模块。它还定义了两个字符串变量:img_path 和 mask_path,分别存储了图像数据和掩码数据的路径。
阅读全文
相关推荐
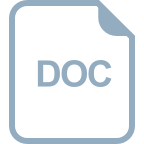
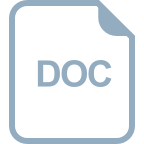
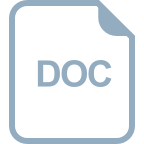










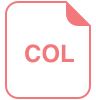
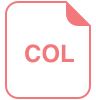
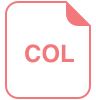
