def forward(self, x, depth): b, c, h, w = x.size() #位置编码 输入x是一个大小为[b, c, h, w]的四维张量 b_d, c_d, h_d, w_d = depth.size() assert b == b_d and c_d == 1 and h == h_d and w == w_d if self.num_pos_feats_x != 0 and self.num_pos_feats_y != 0: y_embed = torch.arange(h, dtype=torch.float32, device=x.device).unsqueeze(1).repeat(b, 1, w) x_embed = torch.arange(w, dtype=torch.float32, device=x.device).repeat(b, h, 1) z_embed = depth.squeeze().to(dtype=torch.float32, device=x.device)
时间: 2024-01-07 15:03:58 浏览: 25
这段代码是用于生成位置编码的,其中x是输入的四维张量,depth是深度信息的一维张量。首先,代码会判断输入x的大小与depth的大小是否匹配。如果num_pos_feats_x和num_pos_feats_y不为0,则会生成y_embed和x_embed分别表示y和x轴上的位置编码。最后,将depth的张量形状变为与x相同,并转换为float32类型。
相关问题
def forward(self, x): """Forward function.""" depth_map = self.DarkChannel(x) x = self.patch_embed(x)
这段代码是在一个神经网络模型的 forward 函数中,对输入的 x 进行了处理。具体来说,它先使用 DarkChannel 函数对输入进行处理得到深度图 depth_map,然后使用 patch_embed 函数对输入进行 patch embedding,生成一个新的表示 x。这个新的表示可以被输入到模型的后续层中进行处理。
class BasicBlock(nn.Module): def __init__(self, net_depth, dim, kernel_size=3, conv_layer=ConvLayer, norm_layer=nn.BatchNorm2d, gate_act=nn.Sigmoid): super().__init__() self.norm = norm_layer(dim) self.conv = conv_layer(net_depth, dim, kernel_size, gate_act) def forward(self, x): identity = x x = self.norm(x) x = self.conv(x) x = identity + x return x转化为Paddle框架写法
class BasicBlock(fluid.dygraph.Layer):
def __init__(self, net_depth, dim, kernel_size=3, conv_layer=ConvLayer, norm_layer=nn.BatchNorm2d, gate_act=fluid.dygraph.nn.functional.sigmoid):
super(BasicBlock, self).__init__()
self.norm = norm_layer(dim)
self.conv = conv_layer(net_depth, dim, kernel_size, gate_act)
def forward(self, x):
identity = x
x = self.norm(x)
x = self.conv(x)
x = identity + x
return x
相关推荐
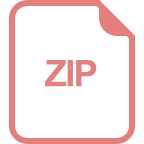
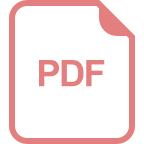
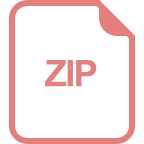







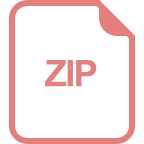
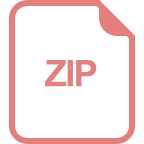