{test_loss:>8f} \n"
时间: 2024-04-28 15:20:20 浏览: 18
这似乎是一个 Python 的格式化输出语句,其中 test_loss 是一个变量,:>8f 表示将 test_loss 格式化为浮点数,且总共占据 8 个字符的宽度,右对齐输出。输出结果可能类似于 " 1.234567"。不过,我需要提醒您的是,这段代码缺少上下文,无法确定 test_loss 的值,因此无法准确回答您的问题。
相关问题
print(f'test error: \n accuracy:{(100*correct):>0.1f}%, ave loss: {test_loss:>8f}')
这是一个使用 f-string 格式化输出的例子。在这个例子中,我们使用了两个变量 correct 和 test_loss。correct 表示正确的数量,test_loss 表示测试损失。这两个变量被插入到字符串中的占位符中。
在占位符中,我们使用了 :>0.1f 和 :>8f。这些是格式化选项,用于指定输出的格式。其中,:>0.1f 表示右对齐,至少占用 0 个字符,并保留一位小数。而 :>8f 表示右对齐,至少占用 8 个字符,并保留八位小数。
最终的输出结果将是类似于下面的形式:
test error:
accuracy: 99.9%, ave loss: 0.12345678
为以下代码添加注释def test(dataloader, model, loss_fn): size = len(dataloader.dataset) num_batches = len(dataloader) model.eval() test_loss, correct = 0, 0 with torch.no_grad(): for X, y in dataloader: X, y = X.to(device), y.to(device) pred = model(X) test_loss += loss_fn(pred, y).item() correct += (pred.argmax(1) == y).type(torch.float).sum().item() test_loss /= num_batches correct /= size print(f"Test Error: \n Accuracy: {(100*correct):>0.1f}%, Avg loss: {test_loss:>8f} \n")
# 定义一个测试函数,用于测试模型
def test(dataloader, model, loss_fn):
# 获取数据集大小和批次数
size = len(dataloader.dataset)
num_batches = len(dataloader)
# 将模型设为评估模式
model.eval()
# 初始化测试损失和正确率
test_loss, correct = 0, 0
# 关闭梯度计算
with torch.no_grad():
# 遍历数据集
for X, y in dataloader:
# 将数据和标签移动到设备上
X, y = X.to(device), y.to(device)
# 前向传播计算预测值
pred = model(X)
# 计算损失
test_loss += loss_fn(pred, y).item()
# 统计正确率
correct += (pred.argmax(1) == y).type(torch.float).sum().item()
# 计算平均测试损失和正确率
test_loss /= num_batches
correct /= size
# 输出测试结果
print(f"Test Error: \n Accuracy: {(100*correct):>0.1f}%, Avg loss: {test_loss:>8f} \n")
相关推荐
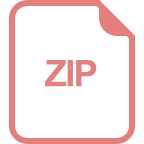
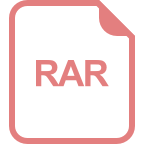
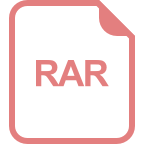













