node_filefd_allocated{job="node-exporter"} * 100 / node_filefd_maximum{job="node-exporter"}
时间: 2024-10-15 13:27:20 浏览: 35
该表达式`node_filefd_allocated{job="node-exporter"} * 100 / node_filefd_maximum{job="node-exporter"}`是一个Prometheus监控指标的计算方式,用于计算名为"node-exporter"的节点上已分配文件描述符占最大可用文件描述符的比例。
在Node.js中,每个进程(如由Node.js运行的服务)都有一个最大文件描述符限制(通过`/proc/sys/fs/file-max`文件查看)。`node_filefd_allocated`度量表示当前进程已经使用的文件描述符数量,而`node_filefd_maximum`则代表进程的最大允许文件描述符数量。
这个比率可以用来评估系统的资源利用率,如果值接近100%,可能意味着系统文件描述符管理已经达到极限,可能会对性能产生影响,因为过多的文件描述符可能会导致系统效率下降或资源耗尽。
在Prometheus查询工具(如PromQL)中,你可以直接输入上述表达式来获取实时结果:
```promql
(node_filefd_allocated{job="node-exporter"} * 100 / node_filefd_maximum{job="node-exporter"})
```
请注意,实际操作时,可能需要访问Prometheus服务器或配置正确的标签筛选以匹配特定的"node-exporter"实例。
相关问题
ulint* rec_get_offsets_func( /*=================*/ const rec_t* rec, /*!< in: physical record */ const dict_index_t* index, /*!< in: record descriptor */ ulint* offsets,/*!< in/out: array consisting of offsets[0] allocated elements, or an array from rec_get_offsets(), or NULL */ ulint n_fields,/*!< in: maximum number of initialized fields (ULINT_UNDEFINED if all fields) */ #ifdef UNIV_DEBUG const char* file, /*!< in: file name where called */ ulint line, /*!< in: line number where called */ #endif /* UNIV_DEBUG */ mem_heap_t** heap) /*!< in/out: memory heap */ { ulint n; ulint size; ut_ad(rec); ut_ad(index); ut_ad(heap); if (dict_table_is_comp(index->table)) { switch (UNIV_EXPECT(rec_get_status(rec), REC_STATUS_ORDINARY)) { case REC_STATUS_ORDINARY: n = dict_index_get_n_fields(index); break; case REC_STATUS_NODE_PTR: /* Node pointer records consist of the uniquely identifying fields of the record followed by a child page number field. */ n = dict_index_get_n_unique_in_tree_nonleaf(index) + 1; break; case REC_STATUS_INFIMUM: case REC_STATUS_SUPREMUM: /* infimum or supremum record */ n = 1; break; default: ut_error; return(NULL); } } else { n = rec_get_n_fields_old(rec); } if (UNIV_UNLIKELY(n_fields < n)) { n = n_fields; } /* The offsets header consists of the allocation size at offsets[0] and the REC_OFFS_HEADER_SIZE bytes. */ size = n + (1 + REC_OFFS_HEADER_SIZE); if (UNIV_UNLIKELY(!offsets) || UNIV_UNLIKELY(rec_offs_get_n_alloc(offsets) < size)) { if (UNIV_UNLIKELY(!*heap)) { *heap = mem_heap_create_at(size * sizeof(ulint), file, line); } offsets = static_cast<ulint*>( mem_heap_alloc(*heap, size * sizeof(ulint))); rec_offs_set_n_alloc(offsets, size); } rec_offs_set_n_fields(offsets, n); rec_init_offsets(rec, index, offsets); return(offsets); }帮我以注释的形式解释下这段代码吧
下面是对这段代码的注释解释:
```
/**
* rec_get_offsets_func函数的作用是获取给定记录的字段偏移量数组。
*
* @param rec 指向记录的指针
* @param index 指向记录描述符的指针
* @param offsets 存储字段偏移量数组的指针。如果为NULL,则需要分配一个新的。
* @param n_fields 初始化字段的最大数目。如果为ULINT_UNDEFINED,则初始化所有字段。
* @param file 调用该函数的文件名
* @param line 调用该函数的行号
* @param heap 记录偏移量数组的内存堆
* @return 如果成功,则返回字段偏移量数组的指针;否则返回NULL。
*/
ulint* rec_get_offsets_func(
const rec_t* rec,
const dict_index_t* index,
ulint* offsets,
ulint n_fields,
#ifdef UNIV_DEBUG
const char* file,
ulint line,
#endif /* UNIV_DEBUG */
mem_heap_t** heap)
{
ulint n;
ulint size;
ut_ad(rec);
ut_ad(index);
ut_ad(heap);
// 如果表是COMP压缩的,则根据记录状态判断记录中的字段数目
if (dict_table_is_comp(index->table)) {
switch (UNIV_EXPECT(rec_get_status(rec), REC_STATUS_ORDINARY)) {
case REC_STATUS_ORDINARY:
n = dict_index_get_n_fields(index);
break;
case REC_STATUS_NODE_PTR:
// 节点指针记录由唯一标识字段和子页号字段组成
n = dict_index_get_n_unique_in_tree_nonleaf(index) + 1;
break;
case REC_STATUS_INFIMUM:
case REC_STATUS_SUPREMUM:
// infimum或supremum记录只有一个字段
n = 1;
break;
default:
// 不应该出现的情况
ut_error;
return NULL;
}
} else {
// 如果表没有压缩,则返回记录中的所有字段
n = rec_get_n_fields_old(rec);
}
// 如果要初始化的字段数目比记录中的字段数目少,则只初始化前面的字段
if (UNIV_UNLIKELY(n_fields < n)) {
n = n_fields;
}
// 计算字段偏移量数组的大小
// 头部包括一个ulint类型的分配大小和REC_OFFS_HEADER_SIZE个字节
size = n + (1 + REC_OFFS_HEADER_SIZE);
// 如果没有给定存储字段偏移量数组的指针,或者这个指针指向的空间不足,则需要分配一个新的
if (UNIV_UNLIKELY(!offsets) || UNIV_UNLIKELY(rec_offs_get_n_alloc(offsets) < size)) {
if (UNIV_UNLIKELY(!*heap)) {
*heap = mem_heap_create_at(size * sizeof(ulint), file, line);
}
offsets = static_cast<ulint*>(mem_heap_alloc(*heap, size * sizeof(ulint)));
rec_offs_set_n_alloc(offsets, size);
}
// 设置字段偏移量数组的头部信息
rec_offs_set_n_fields(offsets, n);
// 初始化字段偏移量数组
rec_init_offsets(rec, index, offsets);
return offsets;
}
```
Traceback (most recent call last): File "/root/autodl-tmp/ultralytics-main/run.py", line 7, in <module> model.train(data='/root/autodl-tmp/ultralytics-main/traindata3/data.yaml') File "/root/autodl-tmp/ultralytics-main/ultralytics/yolo/engine/model.py", line 371, in train self.trainer.train() File "/root/autodl-tmp/ultralytics-main/ultralytics/yolo/engine/trainer.py", line 192, in train self._do_train(world_size) File "/root/autodl-tmp/ultralytics-main/ultralytics/yolo/engine/trainer.py", line 328, in _do_train preds = self.model(batch['img']) File "/root/miniconda3/lib/python3.8/site-packages/torch/nn/modules/module.py", line 1501, in _call_impl return forward_call(*args, **kwargs) File "/root/autodl-tmp/ultralytics-main/ultralytics/nn/tasks.py", line 219, in forward return self._forward_once(x, profile, visualize) # single-scale inference, train File "/root/autodl-tmp/ultralytics-main/ultralytics/nn/tasks.py", line 70, in _forward_once x = m(x) # run File "/root/miniconda3/lib/python3.8/site-packages/torch/nn/modules/module.py", line 1501, in _call_impl return forward_call(*args, **kwargs) File "/root/autodl-tmp/ultralytics-main/ultralytics/nn/modules/block.py", line 183, in forward return self.cv2(torch.cat(y, 1)) torch.cuda.OutOfMemoryError: CUDA out of memory. Tried to allocate 100.00 MiB (GPU 0; 23.65 GiB total capacity; 6.18 GiB already allocated; 98.56 MiB free; 6.21 GiB reserved in total by PyTorch) If reserved memory is >> allocated memory try setting max_split_size_mb to avoid fragmentation. See documentation for Memory Management and PYTORCH_CUDA_ALLOC_CONF terminate called without an active exception Aborted (core dumped)
这是一个CUDA out of memory错误,说明你的GPU显存已经不足以支持你的模型在训练时的计算了。你可以尝试减小batch size或者使用更高显存的GPU来解决这个问题。另外,你也可以尝试使用PyTorch提供的一些内存管理的工具,比如设置max_split_size_mb参数来避免内存碎片化。
阅读全文
相关推荐
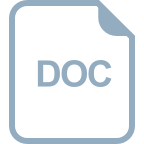
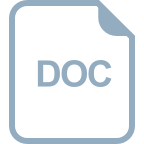
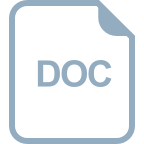













