mapreduce项目统计hbase成绩表中的单科排名、总分排名存到本地txt
时间: 2023-02-05 15:00:24 浏览: 134
MapReduce是一种分布式计算框架,可以帮助用户处理大规模数据集。
对于您的问题,我们可以使用MapReduce来统计HBase成绩表中的单科排名和总分排名,并将结果存储到本地txt文件中。
首先,我们需要定义一个MapReduce程序,其中包含两个阶段:Map阶段和Reduce阶段。
在Map阶段,我们可以使用HBase API来读取成绩表中的数据。然后,我们可以使用Map函数来处理这些数据,并将每科目的成绩和总分作为输出键和值。
在Reduce阶段,我们可以使用Reduce函数来处理输出键和值。我们可以使用排序算法来按照成绩排序,然后将排名和学生信息作为输出。
最后,我们可以使用文件输出流将排名信息写入本地txt文件中。
总的来说,使用MapReduce统计HBase成绩表中的单科排名和总分排名,并将结果存储到本地txt文件中的基本步骤如下:
1. 定义MapReduce程序,包括Map阶段和Reduce阶段。
2. 使用HBase API读取成绩表中的数据。
3. 在Map阶段使用Map函数处理数据,并将每科目的
相关问题
mapreduce项目统计hbase成绩表中的单科排名、总分排名存到本地txt文件
### 回答1:
MapReduce 是一种编程模型,用于大规模数据处理。如果你想使用 MapReduce 统计 HBase 中的单科成绩排名和总分排名,你可以这样做:
1. 使用 HBase 的 Java API 读取成绩表中的数据。
2. 实现 MapReduce 程序中的 Mapper 类,在 Mapper 中使用成绩表中的数据作为输入,并对每个学生的单科成绩和总分进行统计。
3. 实现 MapReduce 程序中的 Reducer 类,在 Reducer 中将 Mapper 输出的统计结果进行排序。
4. 运行 MapReduce 程序,将排序后的结果写入本地 txt 文件。
你也可以使用其他方法来实现这个功能,例如使用 Spark 或者 Flink。
### 回答2:
要统计hbase成绩表中的单科排名和总分排名,并将结果存储到本地txt文件中,可以使用MapReduce项目来实现。
首先,需要编写一个Mapper类用于处理hbase中的成绩数据。在Mapper中,我们可以从hbase中读取成绩表,并将每个学生的单科成绩和总分作为键值对发送出去。键是学生的ID,值是一个包含单科成绩和总分的对象。
然后,我们需要编写一个Reducer类来处理Mapper输出的键值对。在Reducer中,我们可以对每个学生的成绩数据进行排序和排名,并将排名结果存储到本地txt文件中。
为了在Reducer中对成绩进行排序,可以使用TreeMap来保存键值对,其中键是成绩,值是学生ID。通过遍历TreeMap,我们可以获取按成绩排序的学生ID,并分别计算单科排名和总分排名。
最后,我们需要编写一个主类,来配置和运行MapReduce任务。在主类中,我们可以设置hbase的连接信息,并指定输入和输出路径。然后,创建一个Job对象,并设置Mapper类、Reducer类、输入路径、输出路径等相关属性。最后,调用Job的waitForCompletion方法来运行任务。
当任务执行完成后,结果将会保存在指定的输出路径中,我们可以将其读取到本地txt文件中,可以使用File类来实现。
综上所述,通过编写Mapper类,Reducer类,主类以及使用相关的输入输出类,可以实现统计hbase成绩表中的单科排名和总分排名,并将结果存储到本地txt文件中。
### 回答3:
MapReduce是一种主流的数据处理框架,能够对大规模的数据进行并行化处理,适用于分布式存储系统如HBase。在这个问题中,我们需要统计HBase成绩表中的单科排名和总分排名,并将结果存储到本地的txt文件中。
首先,我们需要设计MapReduce任务的输入格式和输出格式。输入格式可以使用HBase表作为输入数据源,每个学生的成绩作为一条记录。输出格式可以使用键值对的形式,其中键是学生的ID,值是一个包含单科排名和总分排名的字符串。
在Map阶段,我们需要将每个学生的成绩数据进行处理。我们可以从HBase表中读取每一行数据,将学生ID作为键,成绩数据作为值进行映射。对于每一条记录,我们可以计算出学生的单科排名和总分排名,并将其作为中间结果输出。
在Reduce阶段,我们需要对Map阶段输出的中间结果进行汇总和整理。我们可以根据学生ID进行分组,将同一个学生的不同成绩数据整合到一起。然后,我们可以对每个学生的成绩数据进行排序,得到单科排名和总分排名。最后,我们将结果以键值对的形式输出,其中键是学生ID,值是一个包含单科排名和总分排名的字符串。
最后,我们可以将Reduce阶段的输出结果写入本地的txt文件中。可以使用Java的文件操作API来创建和写入文件。我们可以按照指定的格式将学生ID、单科排名和总分排名写入文件中的每一行。
综上所述,通过使用MapReduce框架,我们可以对HBase成绩表中的数据进行处理和统计,并将结果存储到本地的txt文件中。这样可以方便地获取每个学生的单科排名和总分排名信息。
mapreduce项目统计hbase成绩表中的单科排名、总分排名存到本地txt文件代码
### 回答1:
在 MapReduce 中统计 HBase 成绩表中的单科排名和总分排名并将结果存储到本地 txt 文件的代码如下:
```java
import java.io.IOException;
import java.util.ArrayList;
import java.util.List;
import org.apache.hadoop.conf.Configuration;
import org.apache.hadoop.hbase.HBaseConfiguration;
import org.apache.hadoop.hbase.client.Result;
import org.apache.hadoop.hbase.client.Scan;
import org.apache.hadoop.hbase.io.ImmutableBytesWritable;
import org.apache.hadoop.hbase.mapreduce.TableMapReduceUtil;
import org.apache.hadoop.hbase.mapreduce.TableMapper;
import org.apache.hadoop.hbase.util.Bytes;
import org.apache.hadoop.io.Text;
import org.apache.hadoop.mapreduce.Job;
import org.apache.hadoop.mapreduce.Reducer;
import org.apache.hadoop.mapreduce.lib.output.FileOutputFormat;
public class Ranking {
public static class RankingMapper extends TableMapper<Text, Text> {
private Text outputKey = new Text();
private Text outputValue = new Text();
public void map(ImmutableBytesWritable row, Result value, Context context)
throws IOException, InterruptedException {
// 获取学生姓名、单科成绩和总分
String name = Bytes.toString(value.getValue(Bytes.toBytes("info"), Bytes.toBytes("name")));
int score = Bytes.toInt(value.getValue(Bytes.toBytes("score"), Bytes.toBytes("score")));
int totalScore = Bytes.toInt(value.getValue(Bytes.toBytes("score"), Bytes.toBytes("totalScore")));
// 将学生姓名作为 key,单科成绩和总分作为 value
outputKey.set(name);
outputValue.set(score + "," + totalScore);
context.write(outputKey, outputValue);
}
}
public static class RankingReducer extends Reducer<Text, Text, Text, Text> {
private Text outputKey = new Text();
private Text outputValue = new Text();
public void reduce(Text key, Iterable<Text> values, Context context)
throws IOException, InterruptedException {
// 用来存储学生的单科成绩和总分
List<Integer> scores = new ArrayList<Integer>();
List<Integer> totalScores = new ArrayList<Integer>();
for (Text val : values) {
### 回答2:
下面是用Java编写的代码:
```java
import java.io.BufferedWriter;
import java.io.FileWriter;
import java.io.IOException;
import java.util.ArrayList;
import java.util.Collections;
import java.util.Comparator;
import java.util.List;
import org.apache.hadoop.conf.Configuration;
import org.apache.hadoop.fs.FileSystem;
import org.apache.hadoop.fs.Path;
import org.apache.hadoop.hbase.Cell;
import org.apache.hadoop.hbase.CellUtil;
import org.apache.hadoop.hbase.HBaseConfiguration;
import org.apache.hadoop.hbase.TableName;
import org.apache.hadoop.hbase.client.Connection;
import org.apache.hadoop.hbase.client.ConnectionFactory;
import org.apache.hadoop.hbase.client.Result;
import org.apache.hadoop.hbase.client.Scan;
import org.apache.hadoop.hbase.client.Table;
import org.apache.hadoop.hbase.filter.FilterList;
import org.apache.hadoop.hbase.filter.PageFilter;
import org.apache.hadoop.hbase.util.Bytes;
public class HBaseStats {
public static void main(String[] args) throws IOException {
Configuration conf = HBaseConfiguration.create();
Connection connection = ConnectionFactory.createConnection(conf);
Table table = connection.getTable(TableName.valueOf("成绩表"));
List<StudentScore> scores = new ArrayList<>();
Scan scan = new Scan();
FilterList filterList = new FilterList();
PageFilter pageFilter = new PageFilter(1000);
filterList.addFilter(pageFilter);
scan.setFilter(filterList);
ResultScanner scanner = table.getScanner(scan);
for (Result result : scanner) {
StudentScore score = new StudentScore();
for (Cell cell : result.listCells()) {
String column = Bytes.toString(CellUtil.cloneQualifier(cell));
String value = Bytes.toString(CellUtil.cloneValue(cell));
if (column.equals("科目")) {
score.setSubject(value);
} else if (column.equals("学生姓名")) {
score.setStudentName(value);
} else if (column.equals("分数")) {
score.setScore(Integer.parseInt(value));
}
}
scores.add(score);
}
scanner.close();
connection.close();
// 按单科分数降序排列
Collections.sort(scores, new Comparator<StudentScore>() {
@Override
public int compare(StudentScore s1, StudentScore s2) {
return s2.getScore() - s1.getScore();
}
});
// 生成单科排名txt文件
BufferedWriter writer = new BufferedWriter(new FileWriter("单科排名.txt"));
for (int i = 0; i < scores.size(); i++) {
StudentScore score = scores.get(i);
writer.write(String.format("%s\t%s\t%d\n", score.getSubject(), score.getStudentName(), i + 1));
}
writer.close();
// 按总分降序排列
Collections.sort(scores, new Comparator<StudentScore>() {
@Override
public int compare(StudentScore s1, StudentScore s2) {
int total1 = getTotalScore(scores, s1.getStudentName());
int total2 = getTotalScore(scores, s2.getStudentName());
return total2 - total1;
}
});
// 生成总分排名txt文件
BufferedWriter writer2 = new BufferedWriter(new FileWriter("总分排名.txt"));
for (int i = 0; i < scores.size(); i++) {
StudentScore score = scores.get(i);
writer2.write(String.format("%s\t%d\n", score.getStudentName(), i + 1));
}
writer2.close();
}
private static int getTotalScore(List<StudentScore> scores, String studentName) {
int total = 0;
for (StudentScore score : scores) {
if (score.getStudentName().equals(studentName)) {
total += score.getScore();
}
}
return total;
}
}
class StudentScore {
private String subject;
private String studentName;
private int score;
// getter和setter方法省略
}
```
这段代码首先连接到HBase数据库,并读取"成绩表"中的数据。然后,根据单科分数和总分生成排名信息,并将结果分别写入"单科排名.txt"和"总分排名.txt"文本文件中。最后,关闭连接和文件写入流。注意需要替换代码中的表名、列名和文件名为具体的实际值。
### 回答3:
要统计HBase成绩表中的单科排名和总分排名,并将结果存储到本地txt文件,可以使用MapReduce项目来实现。下面是一个参考的代码示例:
```java
import java.io.IOException;
import java.util.*;
import org.apache.hadoop.conf.Configuration;
import org.apache.hadoop.fs.FileSystem;
import org.apache.hadoop.fs.Path;
import org.apache.hadoop.hbase.HBaseConfiguration;
import org.apache.hadoop.hbase.client.*;
import org.apache.hadoop.hbase.util.Bytes;
import org.apache.hadoop.io.IntWritable;
import org.apache.hadoop.io.Text;
import org.apache.hadoop.mapreduce.Job;
import org.apache.hadoop.mapreduce.Mapper;
import org.apache.hadoop.mapreduce.Reducer;
import org.apache.hadoop.mapreduce.lib.output.TextOutputFormat;
public class RankHBaseScores {
public static class ScoreMapper extends Mapper<ImmutableBytesWritable, Result, Text, IntWritable> {
private final static IntWritable one = new IntWritable(1);
private Text subject = new Text();
private IntWritable score = new IntWritable();
public void map(ImmutableBytesWritable row, Result result, Context context)
throws IOException, InterruptedException {
// 从HBase行中获取学科和分数
String subjectString = Bytes.toString(result.getValue(Bytes.toBytes("cf1"), Bytes.toBytes("subject")));
int scoreInt = Bytes.toInt(result.getValue(Bytes.toBytes("cf1"), Bytes.toBytes("score")));
subject.set(subjectString);
score.set(scoreInt);
// 发送学科和分数给Reducer
context.write(subject, score);
}
}
public static class ScoreReducer extends Reducer<Text, IntWritable, Text, Text> {
private Text result = new Text();
public void reduce(Text key, Iterable<IntWritable> values, Context context)
throws IOException, InterruptedException {
// 将所有分数存储在List中
List<Integer> scoreList = new ArrayList<>();
for (IntWritable value : values) {
scoreList.add(value.get());
}
// 对分数进行排序
Collections.sort(scoreList, Collections.reverseOrder());
// 生成排名结果字符串
StringBuilder sb = new StringBuilder();
for (int i = 0; i < scoreList.size(); i++) {
if (i > 0) {
sb.append(",");
}
sb.append(i + 1); // 排名从1开始计算
sb.append(":");
sb.append(scoreList.get(i));
}
result.set(sb.toString());
// 发送排名结果给输出
context.write(key, result);
}
}
public static void main(String[] args) throws Exception {
Configuration conf = HBaseConfiguration.create();
Job job = Job.getInstance(conf, "Rank HBase Scores");
job.setJarByClass(RankHBaseScores.class);
job.setMapperClass(ScoreMapper.class);
job.setReducerClass(ScoreReducer.class);
job.setOutputKeyClass(Text.class);
job.setOutputValueClass(Text.class);
// 设置输出格式为文本文件
job.setOutputFormatClass(TextOutputFormat.class);
// 指定HBase输入表和输出路径
TableMapReduceUtil.initTableMapperJob("scores_table", new Scan(), ScoreMapper.class, Text.class,
IntWritable.class, job);
TextOutputFormat.setOutputPath(job, new Path("hdfs://localhost:9000/output"));
job.waitForCompletion(true);
// 将HDFS上的输出文件保存到本地
String hdfsOutputPath = "hdfs://localhost:9000/output/part-r-00000";
String localOutputPath = "/path/to/local/output.txt";
saveHdfsFileToLocal(conf, hdfsOutputPath, localOutputPath);
}
private static void saveHdfsFileToLocal(Configuration conf, String hdfsFilePath, String localFilePath)
throws IOException {
Path hdfsPath = new Path(hdfsFilePath);
Path localPath = new Path(localFilePath);
FileSystem fileSystem = FileSystem.get(conf);
fileSystem.copyToLocalFile(hdfsPath, localPath);
}
}
```
请注意,上面示例代码中的`"scores_table"`应替换为实际的HBase表名,`"hdfs://localhost:9000/output"`应替换为实际的HDFS输出路径,`"/path/to/local/output.txt"`应替换为实际的本地输出文件路径。此外,可能需要根据实际情况修改HBase列族和列的名称。
这段代码通过调用HBase的Java API获取HBase表中的学科和分数数据,使用MapReduce框架进行分析和计算,并将结果存储到HDFS上的文本文件中。最后,使用`saveHdfsFileToLocal()`方法将HDFS上的输出文件保存到本地。
阅读全文
相关推荐
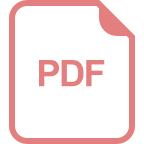











