tree_model.score
时间: 2024-06-01 17:10:42 浏览: 19
The function `score` for a decision tree model in machine learning is used to calculate the accuracy of the model. The accuracy is the ratio of the number of correct predictions made by the model to the total number of predictions made.
Syntax: `tree_model.score(X_test, y_test)`
where:
- `tree_model`: Decision tree model object
- `X_test`: Test data features
- `y_test`: Test data target variable
The `score` function returns the accuracy of the model on the test data. The accuracy score ranges from 0 to 1, where 1 represents a perfect prediction.
相关问题
我已经用数据训练好决策树和随机森林,现在需要用输入数据,用数据预测这组数据描述的病人是否患有心脏病,请给我测试的代码。下面是我的训练过程import pandas as pd from sklearn import tree from sklearn.ensemble import RandomForestClassifier import matplotlib.pyplot as plt import numpy as np heart=pd.read_csv("D:\Anaconda1\heart.csv") heart.head() heart.info() clf_tree = tree.DecisionTreeClassifier() from sklearn.model_selection import train_test_split x = heart.drop('target',axis = 1) y = heart['target'] x_train,x_test,y_train,y_test = train_test_split(x,y,random_state=0) clf_tree.fit(x_train,y_train) print(clf_tree.score(x_train,y_train)) print(clf_tree.score(x_test,y_test)) tree.export_graphviz(clf, out_file='tree.dot') score_list=[] for i in range(10,100,10): clf_forest = RandomForestClassifier(n_estimators = i, random_state = 0) clf_forest.fit(x_train,y_train) score_list.append(clf_forest.score(x_test,y_test)) plt.plot(range(10,100,10), score_list) plt.show() print(np.argmax(score_list)) print(np.max(score_list))
好的,给您提供一份用训练好的决策树和随机森林模型进行预测的代码:
```
import pandas as pd
from sklearn import tree
from sklearn.ensemble import RandomForestClassifier
# 读入测试数据
test_data = pd.read_csv("test_data.csv")
# 加载训练好的决策树模型
clf_tree = tree.DecisionTreeClassifier()
clf_tree.load("tree_model.pkl")
# 加载训练好的随机森林模型
clf_forest = RandomForestClassifier()
clf_forest.load("forest_model.pkl")
# 预测决策树模型结果
tree_result = clf_tree.predict(test_data)
# 预测随机森林模型结果
forest_result = clf_forest.predict(test_data)
# 输出预测结果
print("决策树模型预测结果:", tree_result)
print("随机森林模型预测结果:", forest_result)
```
需要将上面的代码中的 `test_data.csv` 替换为你要预测的测试数据的文件路径,并且需要将 `tree_model.pkl` 和 `forest_model.pkl` 替换为训练好的决策树模型和随机森林模型的文件路径。
import pandas as pd from sklearn.model_selection import train_test_split from sklearn.tree import DecisionTreeClassifier, plot_tree from sklearn.preprocessing import LabelEncoder import matplotlib.pyplot as plt # 加载csv文件 data = pd.read_csv("data填补.csv") # 将标签进行编码 le = LabelEncoder() data['label'] = le.fit_transform(data['label']) # 划分自变量和因变量 X = data.drop(columns=["label"]) y = data["label"] # 将数据集划分为训练集和测试集 X_train, X_test, y_train, y_test = train_test_split(X, y, test_size=0.2, random_state=42) # 定义决策树模型 dt_model = DecisionTreeClassifier() # 训练决策树模型 dt_model.fit(X_train, y_train) # 计算测试集的准确率 accuracy = dt_model.score(X_test, y_test) print("测试集准确率:", accuracy) # 可视化决策树 plt.figure(figsize=(30, 30)) plot_tree(dt_model, filled=True, feature_names=X.columns, class_names=le.classes_) plt.show()我想使这段代码生成的决策图只显示置信度大于0.95的区间应该如何更改
你可以在 `plot_tree()` 函数中添加 `precision` 参数,来控制节点中显示的类别置信度的精度。默认情况下,`precision` 参数的值为 3,可以将其设置为 2 或更高的值,以显示更高精度的置信度。然后,在生成决策树图后,可以使用 matplotlib 库的相关函数来调整节点的可视化效果,只显示置信度大于 0.95 的节点。
修改后的代码如下:
```python
import pandas as pd
from sklearn.model_selection import train_test_split
from sklearn.tree import DecisionTreeClassifier, plot_tree
from sklearn.preprocessing import LabelEncoder
import matplotlib.pyplot as plt
# 加载csv文件
data = pd.read_csv("data填补.csv")
# 将标签进行编码
le = LabelEncoder()
data['label'] = le.fit_transform(data['label'])
# 划分自变量和因变量
X = data.drop(columns=["label"])
y = data["label"]
# 将数据集划分为训练集和测试集
X_train, X_test, y_train, y_test = train_test_split(X, y, test_size=0.2, random_state=42)
# 定义决策树模型
dt_model = DecisionTreeClassifier()
# 训练决策树模型
dt_model.fit(X_train, y_train)
# 计算测试集的准确率
accuracy = dt_model.score(X_test, y_test)
print("测试集准确率:", accuracy)
# 可视化决策树
plt.figure(figsize=(30, 30))
plot_tree(dt_model, filled=True, feature_names=X.columns, class_names=le.classes_, precision=2)
# 调整节点的可视化效果,只显示置信度大于0.95的节点
for node in dt_model.tree_.children_left:
confidence = dt_model.tree_.value[node][0][y_train.mode()[0]]
if confidence >= 0.95:
plt.gca().texts[node].set_text(f'Confidence: {confidence:.2f}')
else:
plt.gca().texts[node].set_text('')
for node in dt_model.tree_.children_right:
confidence = dt_model.tree_.value[node][0][y_train.mode()[0]]
if confidence >= 0.95:
plt.gca().texts[node].set_text(f'Confidence: {confidence:.2f}')
else:
plt.gca().texts[node].set_text('')
plt.show()
```
这样修改后,生成的决策树图中,只有置信度大于 0.95 的节点会显示置信度值,其余节点将不显示。
相关推荐
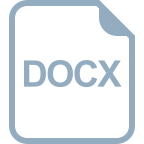
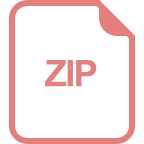
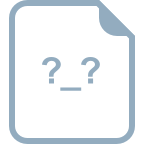












