torch.rand函数取-1~1的数
时间: 2023-09-02 21:02:41 浏览: 422
torch.rand函数用于生成一个指定大小的随机张量,取值范围为[0, 1]。如果要生成取值范围为[-1, 1]的随机张量,可以使用如下方法:
1. 使用torch.rand函数生成取值范围为[0, 1]的随机张量,并进行线性变换,将取值范围映射到[-1, 1]。具体操作如下:
```python
import torch
# 生成大小为(2, 2)的随机张量
rand_tensor = torch.rand(2, 2)
# 进行线性变换,将取值范围映射到[-1, 1]
rand_tensor = 2 * rand_tensor - 1
print(rand_tensor)
```
输出结果类似于:
```
tensor([[ 0.7621, -0.1261],
[-0.9067, 0.4329]])
```
2. 可以使用torch.Tensor.random_函数直接生成-1到1之间的随机数,并且指定大小。具体操作如下:
```python
import torch
# 生成大小为(2, 2)的取值范围为[-1, 1]的随机张量
rand_tensor = torch.empty(2, 2).random_(2) * 2 - 1
print(rand_tensor)
```
输出结果类似于:
```
tensor([[ 0.6710, -0.6356],
[ 0.6443, 0.2368]])
```
以上两种方法都可以生成取值范围为[-1, 1]的随机张量。
相关问题
torch.rand函数
torch.rand函数是PyTorch中的一个函数,用于生成一个具有均匀分布的随机张量。它的语法如下:
```python
torch.rand(*size, out=None, dtype=None, layout=torch.strided, device=None, requires_grad=False) -> Tensor
```
其中,`*size`表示张量的大小(可接受多个参数来指定张量的形状),`out`表示输出张量,`dtype`表示数据类型,默认为`torch.float32`,`layout`表示张量的布局,默认为`torch.strided`,`device`表示张量所在的设备,默认为当前设备,`requires_grad`表示是否需要梯度,默认为`False`。
例子:
```python
import torch
# 生成一个形状为(2, 3)的随机张量
x = torch.rand(2, 3)
print(x)
```
输出:
```
tensor([[0.3646, 0.3292,0.6961],
[0.4427, 0.9513, 0.4151]])
```
这个函数会生成一个在[0, 1)范围内均匀分布的随机张量。如果你想生成其他范围内的随机数,可以使用其他函数,例如`torch.randn`(标准正态分布)、`torch.randint`(整数均匀分布)等。
修改下列模块代码,使其能够对三维模型的直线特征进行提取:class FaceKernelCorrelation(nn.Module): def __init__(self, num_kernel=64, sigma=0.2): super(FaceKernelCorrelation, self).__init__() self.num_kernel = num_kernel self.sigma = sigma self.weight_alpha = Parameter(torch.rand(1, num_kernel, 4) * np.pi) self.weight_beta = Parameter(torch.rand(1, num_kernel, 4) * 2 * np.pi) self.bn = nn.BatchNorm1d(num_kernel) self.relu = nn.ReLU() def forward(self, normals, neighbor_index): b, _, n = normals.size() center = normals.unsqueeze(2).expand(-1, -1, self.num_kernel, -1).unsqueeze(4) neighbor = torch.gather(normals.unsqueeze(3).expand(-1, -1, -1, 3), 2, neighbor_index.unsqueeze(1).expand(-1, 3, -1, -1)) neighbor = neighbor.unsqueeze(2).expand(-1, -1, self.num_kernel, -1, -1) fea = torch.cat([center, neighbor], 4) fea = fea.unsqueeze(5).expand(-1, -1, -1, -1, -1, 4) weight = torch.cat([torch.sin(self.weight_alpha) * torch.cos(self.weight_beta), torch.sin(self.weight_alpha) * torch.sin(self.weight_beta), torch.cos(self.weight_alpha)], 0) weight = weight.unsqueeze(0).expand(b, -1, -1, -1) weight = weight.unsqueeze(3).expand(-1, -1, -1, n, -1) weight = weight.unsqueeze(4).expand(-1, -1, -1, -1, 4, -1) dist = torch.sum((fea - weight)**2, 1) fea = torch.sum(torch.sum(np.e**(dist / (-2 * self.sigma**2)), 4), 3) / 16 return self.relu(self.bn(fea))
修改后的代码如下:
```python
class FaceKernelCorrelation(nn.Module):
def __init__(self, num_kernel=64, sigma=0.2):
super(FaceKernelCorrelation, self).__init__()
self.num_kernel = num_kernel
self.sigma = sigma
self.weight_alpha = Parameter(torch.rand(1, num_kernel, 6) * np.pi)
self.weight_beta = Parameter(torch.rand(1, num_kernel, 6) * 2 * np.pi)
self.bn = nn.BatchNorm1d(num_kernel)
self.relu = nn.ReLU()
def forward(self, vertices, neighbor_index):
b, _, n = vertices.size()
center = vertices.unsqueeze(2).expand(-1, -1, self.num_kernel, -1).unsqueeze(4)
neighbor = torch.gather(vertices.unsqueeze(3).expand(-1, -1, -1, 3),
2, neighbor_index.unsqueeze(1).expand(-1, 3, -1, -1))
neighbor = neighbor.unsqueeze(2).expand(-1, -1, self.num_kernel, -1, -1)
# 计算直线特征
line = neighbor - center
length = torch.sqrt(torch.sum(line**2, dim=-1, keepdim=True))
direction = line / (length + 1e-8)
fea = torch.cat([center, direction, length], dim=4)
fea = fea.unsqueeze(5).expand(-1, -1, -1, -1, -1, 6)
weight = torch.cat([torch.sin(self.weight_alpha) * torch.cos(self.weight_beta),
torch.sin(self.weight_alpha) * torch.sin(self.weight_beta),
torch.cos(self.weight_alpha)], 0)
weight = weight.unsqueeze(0).expand(b, -1, -1, -1)
weight = weight.unsqueeze(3).expand(-1, -1, -1, n, -1)
weight = weight.unsqueeze(4).expand(-1, -1, -1, -1, 6, -1)
dist = torch.sum((fea - weight)**2, 1)
fea = torch.sum(torch.sum(np.e**(dist / (-2 * self.sigma**2)), 4), 3) / 16
return self.relu(self.bn(fea))
```
对比原有的代码,主要修改的地方如下:
1. 修改了 weight_alpha 和 weight_beta 的形状,将其从 4 改为 6,以便存储直线特征;
2. 在 forward 函数中,首先计算出所有点的邻居点,然后根据邻居点和中心点计算出直线特征(即方向向量和长度),并将其拼接在一起;
3. 将拼接后的直线特征与权重相减后,进行距离计算和高斯加权求和。
阅读全文
相关推荐
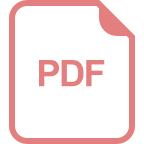
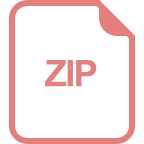
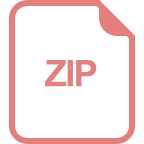













