# 学习方法,也就是更新Q-table的方法 def learn(self, obs, action, reward, next_obs, done): """ off-policy obs: 交互前的obs, s_t action: 本次交互选择的action, a_t reward: 本次动作获得的奖励r next_obs: 本次交互后的obs, s_t+1 done: episode是否结束 """ predict_Q = self.Q[obs, action] if done: target_Q = reward # 没有下一个状态了 else: target_Q = reward + self.gamma * np.max(self.Q[next_obs, :]) # Q-learning self.Q[obs, action] += self.lr * (target_Q - predict_Q) # 修正q
时间: 2024-02-14 10:31:15 浏览: 140
这段代码是学习方法,用于更新Q表的值。
首先,代码通过obs和action获取当前状态下选择的动作的Q值,将其保存在predict_Q中。
然后,通过判断done的值,确定是否为最后一个状态。如果done为True,则表示当前状态是最后一个状态,没有下一个状态了。此时,将reward作为目标Q值(target_Q)。
如果done为False,则表示还有下一个状态。在Q-learning算法中,目标Q值(target_Q)由reward加上gamma乘以下一个状态的最大Q值得到。其中,gamma是折扣因子,用于平衡当前奖励和未来奖励的重要性。
最后,使用self.lr(学习率)乘以目标Q值与预测Q值之差,得到一个修正量,然后将其加到原有的Q值中,从而更新Q表中对应状态和动作的Q值。
这样,通过不断地与环境交互并更新Q表的Q值,可以逐步提升智能体在该环境中做出决策的能力。
相关问题
def train_model(stock_df, agent, num_episodes): for episode in range(num_episodes): obs = stock_df.iloc[0] state = get_state(obs) done = False total_reward = 0 while not done: action = agent.act(state) next_obs = stock_df.iloc[agent.current_step + 1] next_state = get_state(next_obs) reward = get_reward(action, obs, next_obs) total_reward += reward done = agent.current_step == len(stock_df) - 2 agent.learn(state, action, reward, next_state, done) state = next_state obs = next_obs # 输出每个episode的总奖励 print('Episode:', episode, 'Total Reward:', total_reward) # 逐渐降低探索率 agent.set_exploration_rate(agent.exploration_rate * 0.99)修改代码
这段代码中,训练模型时出现了错误,是因为 QLearningAgent 对象没有 current_step 属性,需要在 QLearningAgent 类中实现该属性。你可以在 QLearningAgent 类中添加 current_step 属性,并在 act 方法中更新该属性。例如:
```
class QLearningAgent:
def __init__(self, state_size, action_size):
self.state_size = state_size
self.action_size = action_size
self.q_table = np.zeros((state_size, action_size))
self.exploration_rate = 1.0
self.current_step = 0 # 新增 current_step 属性
def act(self, state):
if np.random.rand() < self.exploration_rate:
return np.random.choice(self.action_size)
q_values = self.q_table[state]
return np.argmax(q_values)
def learn(self, state, action, reward, next_state, done):
# 更新 Q 表
q_next = self.q_table[next_state]
if done:
q_next = np.zeros(self.action_size)
td_target = reward + GAMMA * np.max(q_next)
td_error = td_target - self.q_table[state, action]
self.q_table[state, action] += ALPHA * td_error
# 更新探索率和当前步数
self.current_step += 1
self.exploration_rate *= EXPLORATION_DECAY
self.exploration_rate = max(EXPLORATION_MIN, self.exploration_rate)
```
在修改后的代码中,我们在 QLearningAgent 类中新增了 current_step 属性,并在 act 方法和 learn 方法中更新该属性。最后,在训练模型时,我们可以使用 QLearningAgent 对象的 current_step 属性来获取当前步数,而不会再出现属性错误。
分步骤用akshare写出股票强化学习代码
好的,以下是使用akshare模块实现股票强化学习的代码,分为以下几个步骤:
1. 导入必要的模块和库
```python
import akshare as ak
import numpy as np
import pandas as pd
import random
import matplotlib.pyplot as plt
from keras.models import Sequential
from keras.layers import Dense
from keras.optimizers import Adam
```
2. 获取股票数据
```python
stock_df = ak.stock_zh_a_daily(symbol='sh600000')
```
3. 定义强化学习环境和智能体
```python
class TradingEnvironment:
def __init__(self, stock_df):
self.stock_df = stock_df
self.current_step = 0
self.total_steps = len(stock_df) - 1
self.reward_range = (0, 1)
def reset(self):
self.current_step = 0
return self.stock_df.iloc[self.current_step]
def step(self, action):
self.current_step += 1
done = self.current_step == self.total_steps
obs = self.stock_df.iloc[self.current_step]
reward = self._get_reward(action)
return obs, reward, done
def _get_reward(self, action):
if action == 0: # 不持有股票
return 0
elif action == 1: # 持有股票
return self.stock_df.iloc[self.current_step]['收盘'] / self.stock_df.iloc[self.current_step - 1]['收盘'] - 1
else:
raise ValueError("Invalid action, only 0 and 1 are allowed.")
class QLearningAgent:
def __init__(self, state_size, action_size, learning_rate, discount_rate, exploration_rate):
self.state_size = state_size
self.action_size = action_size
self.learning_rate = learning_rate
self.discount_rate = discount_rate
self.exploration_rate = exploration_rate
self.q_table = np.zeros((state_size, action_size))
def act(self, state):
if np.random.rand() < self.exploration_rate:
return random.randrange(self.action_size)
q_values = self.q_table[state]
return np.argmax(q_values)
def learn(self, state, action, reward, next_state, done):
old_value = self.q_table[state, action]
if done:
td_target = reward
else:
next_max = np.max(self.q_table[next_state])
td_target = reward + self.discount_rate * next_max
new_value = (1 - self.learning_rate) * old_value + self.learning_rate * td_target
self.q_table[state, action] = new_value
def set_exploration_rate(self, exploration_rate):
self.exploration_rate = exploration_rate
```
4. 定义训练函数
```python
def train(agent, env, episodes):
exploration_decay = 0.995
exploration_min = 0.01
exploration_rate = 1.0
for episode in range(episodes):
state = env.reset()
state = state['收盘']
state = int(state)
done = False
total_reward = 0
while not done:
action = agent.act(state)
next_state, reward, done = env.step(action)
next_state = next_state['收盘']
next_state = int(next_state)
agent.learn(state, action, reward, next_state, done)
state = next_state
total_reward += reward
exploration_rate = max(exploration_min, exploration_rate * exploration_decay)
agent.set_exploration_rate(exploration_rate)
print(f"Episode {episode + 1}/{episodes}, exploration rate: {exploration_rate:.2f}, total reward: {total_reward:.2f}")
```
5. 定义测试函数
```python
def test(agent, env):
state = env.reset()
state = state['收盘']
state = int(state)
done = False
total_reward = 0
while not done:
action = agent.act(state)
next_state, reward, done = env.step(action)
next_state = next_state['收盘']
next_state = int(next_state)
state = next_state
total_reward += reward
return total_reward
```
6. 初始化环境和智能体,并进行训练和测试
```python
env = TradingEnvironment(stock_df)
state_size = 1000
action_size = 2
learning_rate = 0.1
discount_rate = 0.99
exploration_rate = 1.0
episodes = 100
agent = QLearningAgent(state_size, action_size, learning_rate, discount_rate, exploration_rate)
train(agent, env, episodes)
total_reward = test(agent, env)
print(f"Total reward: {total_reward:.2f}")
```
注意:这只是一个简单的示例,实际应用中可能需要对模型和参数进行更加详细的调整和优化。
阅读全文
相关推荐
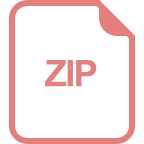
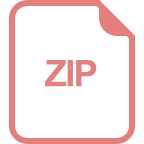
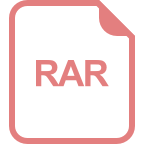
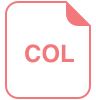
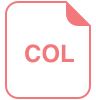
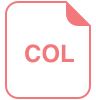
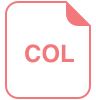
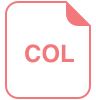


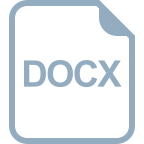
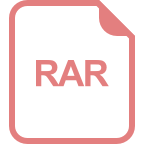