yolov8 make_anchors
时间: 2023-08-04 12:09:57 浏览: 212
根据引用[1],代码中的align_metric和overlaps需要进行过滤,通过与pos_mask相乘来实现。然后,通过找到每个GT的最大匹配值和最大CIOU值,以及计算每个GT与所有anchor的匹配值与每个GT与所有anchor最大IOU的比值,来为类别one-hot标签添加惩罚项。最后,将类别添加惩罚项后的结果赋值给target_labels。
根据引用[2],可以得到gt_boxes的左上角lt和右下角rb,然后将anchor_points减去lt,rb减去anchor_points,如果结果均为正,则说明该anchor_point在gt_box内部。通过这个操作,可以得到对应的mask,即in_gts_mask。
根据引用[3],可以使用代码中的__get_in_gts_mask函数来判断anchor锚点是否在gtbox内部。
相关问题
File "E:/learning/NEW/code/yolov8/NWPU/yolov8-pytorch-master/train.py", line 548, in <module> fit_one_epoch(model_train, model, ema, yolo_loss, loss_history, eval_callback, optimizer, epoch, epoch_step, epoch_step_val, gen, gen_val, UnFreeze_Epoch, Cuda, fp16, scaler, save_period, save_dir, local_rank) File "E:\learning\NEW\code\yolov8\NWPU\yolov8-pytorch-master\utils\utils_fit.py", line 34, in fit_one_epoch outputs = model_train(images) File "D:\Anaconda3\envs\pytorch\lib\site-packages\torch\nn\modules\module.py", line 1130, in _call_impl return forward_call(*input, **kwargs) File "D:\Anaconda3\envs\pytorch\lib\site-packages\torch\nn\parallel\data_parallel.py", line 166, in forward return self.module(*inputs[0], **kwargs[0]) File "D:\Anaconda3\envs\pytorch\lib\site-packages\torch\nn\modules\module.py", line 1130, in _call_impl return forward_call(*input, **kwargs) File "E:\learning\NEW\code\yolov8\NWPU\yolov8-pytorch-master\nets\yolo.py", line 165, in forward self.anchors, self.strides = (x.transpose(0, 1) for x in make_anchors(x, self.stride, 0.5)) File "E:\learning\NEW\code\yolov8\NWPU\yolov8-pytorch-master\utils\utils_bbox.py", line 25, in make_anchors sy,sx = torch.meshgrid(sy, sx, indexing='ij') if TORCH_1_10 else torch.meshgrid(sy, sx) File "D:\Anaconda3\envs\pytorch\lib\site-packages\torch\functional.py", line 463, in meshgrid return _meshgrid(*tensors, indexing=indexing) File "D:\Anaconda3\envs\pytorch\lib\site-packages\torch\functional.py", line 478, in _meshgrid return _VF.meshgrid(tensors, **kwargs,indexing='ij') # type: ignore[attr-defined] TypeError: meshgrid() got multiple values for keyword argument 'indexing'
这个错误是由于你使用了 PyTorch 的多 GPU 训练,但是在使用 meshgrid 函数时,指定了 indexing 参数两次,导致报错。你可以检查一下代码中使用 meshgrid 函数的地方,确保只指定了一次 indexing 参数。如果还有问题,可以提供更多的代码和错误信息,便于更好地定位问题。
File "E:\learning\NEW\code\yolov8\NWPU\yolov8-pytorch-master\utils\utils_bbox.py", line 25, in make_anchors sy,sx = torch.meshgrid(sy, sx, indexing='ij') if TORCH_1_10 else torch.meshgrid(sy, sx) File "D:\Anaconda3\envs\pytorch\lib\site-packages\torch\functional.py", line 463, in meshgrid return _meshgrid(*tensors, indexing=indexing) File "D:\Anaconda3\envs\pytorch\lib\site-packages\torch\functional.py", line 478, in _meshgrid return _VF.meshgrid(tensors, **kwargs,indexing='ij') # type: ignore[attr-defined] TypeError: meshgrid() got multiple values for keyword argument 'indexing'
这个错误是因为`meshgrid`函数的`indexing`参数在PyTorch 1.10版本中被添加,而早期版本中没有该参数。你可以尝试以下两个解决方法中的任意一个:
1. 如果你使用的是PyTorch 1.10或更高版本,请删除`if TORCH_1_10 else`这一行代码,直接使用以下代码即可:
```
sy, sx = torch.meshgrid(sy, sx, indexing='ij')
```
2. 如果你使用的是早期版本的PyTorch,请将代码改为以下形式:
```
if TORCH_1_10:
sy, sx = torch.meshgrid(sy, sx, indexing='ij')
else:
sy, sx = torch.meshgrid(sy, sx)
```
阅读全文
相关推荐
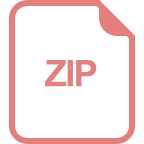
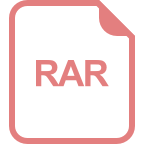
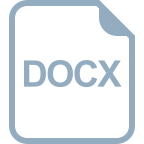
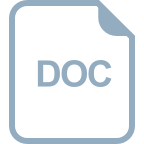
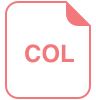










