matrix power python
时间: 2023-07-14 21:05:48 浏览: 123
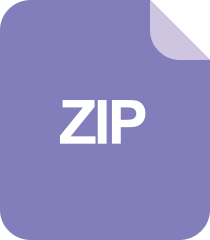
斐波那契数列python
你可以使用numpy库中的函数来计算矩阵的幂。以下是一个示例代码:
```python
import numpy as np
# 定义矩阵
matrix = np.array([[1, 2], [3, 4]])
# 计算矩阵的幂
power = 3
result = np.linalg.matrix_power(matrix, power)
print(result)
```
这将打印出矩阵的3次幂的结果。请注意,你需要安装numpy库才能运行此代码。
阅读全文
相关推荐
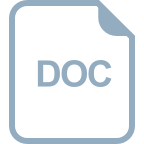
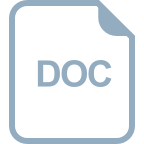
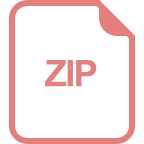
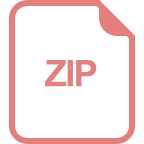
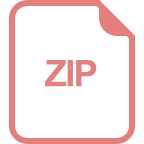
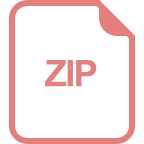
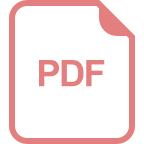










