h=ax.contourf(lon,lat,rp1,cmap='RdYlGn', transform=ccrs.PlateCarree())怎么只画-30-30之间的rp1
时间: 2024-01-16 11:01:49 浏览: 35
你可以使用numpy的ma.masked_outside()函数来只保留-30到30之间的值,并将其它值剔除。例如:
```python
import numpy as np
# 将不在-30到30之间的值设为np.nan
rp1[~np.logical_and(rp1>=-30, rp1<=30)] = np.nan
# 使用ma.masked_outside()函数剔除不在-30到30之间的值
rp1_masked = np.ma.masked_outside(rp1, -30, 30)
# 画图
h = ax.contourf(lon, lat, rp1_masked, cmap='RdYlGn', transform=ccrs.PlateCarree())
```
这样,rp1数组中不在-30到30之间的值就会被剔除,并且不会在画图中显示出来。
相关问题
h=ax.contourf(lon,lat,rp1,cmap='RdYlGn', transform=ccrs.PlateCarree())怎么在画图时剔除缺测值
你可以使用numpy的ma.masked_where()函数来将缺测值剔除。例如,如果你的rp1数组中的缺测值为-999,你可以这样写:
```python
import numpy as np
# 将缺测值设为np.nan
rp1[rp1==-999] = np.nan
# 使用ma.masked_where()函数剔除缺测值
rp1_masked = np.ma.masked_where(np.isnan(rp1), rp1)
# 画图
h = ax.contourf(lon, lat, rp1_masked, cmap='RdYlGn', transform=ccrs.PlateCarree())
```
这样,rp1数组中的缺测值就会被剔除,并且不会在画图中显示出来。
rp1_masked = np.ma.masked_outside(rp1, -30, 30) # 画图 h = ax.contourf(lon, lat, rp1_masked, cmap='RdYlGn', transform=ccrs.PlateCarree())什么意思
这段代码的作用是将`rp1`数组中不在-30到30之间的值剔除,并将剔除后的结果保存在`rp1_masked`中。然后使用`ax.contourf()`函数画出经纬度网格为`lon,lat`,填充值为`rp1_masked`的等值线图,使用的颜色映射为`RdYlGn`,投影方式为`ccrs.PlateCarree()`。
具体来说,`np.ma.masked_outside()`函数的作用是创建一个掩码数组,将不在指定范围内的值设为掩码,只保留在指定范围内的值。在这里,我们将不在-30到30之间的值设为掩码,这些掩码的位置在`rp1_masked`数组中的值为`--`,表示这些值在画图时会被忽略。
然后,我们使用`ax.contourf()`函数画出等值线图,其中填充值为`rp1_masked`,被掩码的值不会被填充,也不会在图中显示出来。这样就实现了只画出-30到30之间的`rp1`值的效果。
相关推荐
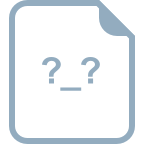
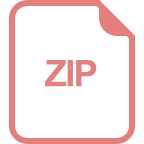
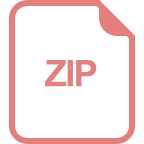












