parameters = initialize_parameters(3,2,1)这段代码是用来做什么的
时间: 2024-04-07 17:32:37 浏览: 72
这段代码是用来初始化一个神经网络的参数的。具体来说,这里的参数是指神经网络中的权重矩阵和偏置向量,initialize_parameters函数的输入参数是神经网络的层数和每层的神经元个数,输出是一个Python字典类型的变量,包含了各层的权重矩阵和偏置向量。在神经网络的正向传播和反向传播计算中,这些参数将被使用。
相关问题
ef initialize_parameters(): tf.set_random_seed(1) W1 = tf.get_variable("W1", shape=[4, 4, 3, 8], initializer=tf.contrib.layers.xavier_initializer(seed=0)) W2 = tf.get_variable("W2", shape=[2, 2, 8, 16], initializer=tf.contrib.layers.xavier_initializer(seed=0)) parameters = {"W1": W1, "W2": W2} return parameters tf.reset_default_graph() with tf.Session() as sess_test: parameters = initialize_parameters() init = tf.global_variables_initializer() sess_test.run(init) print("W1 = " + str(parameters["W1"].eval()[1,1,1])) print("W2 = " + str(parameters["W2"].eval()[1,1,1])) 请根据现在python版本纠正这段代码
根据最新的Python版本,你需要进行以下修改:
```python
import tensorflow.compat.v1 as tf
tf.disable_v2_behavior()
def initialize_parameters():
tf.set_random_seed(1)
initializer = tf.initializers.GlorotUniform(seed=0)
W1 = tf.get_variable("W1", shape=[4, 4, 3, 8], initializer=initializer)
W2 = tf.get_variable("W2", shape=[2, 2, 8, 16], initializer=initializer)
parameters = {"W1": W1, "W2": W2}
return parameters
tf.reset_default_graph()
with tf.Session() as sess_test:
parameters = initialize_parameters()
init = tf.global_variables_initializer()
sess_test.run(init)
print("W1 = " + str(parameters["W1"].eval()[1, 1, 1]))
print("W2 = " + str(parameters["W2"].eval()[1, 1, 1]))
```
这段代码使用了最新的TensorFlow版本,并且使用了`tf.initializers.GlorotUniform`进行参数初始化。
# GRADED FUNCTION: forward_propagation def forward_propagation(X, parameters): """ Implements the forward propagation for the model: CONV2D -> RELU -> MAXPOOL -> CONV2D -> RELU -> MAXPOOL -> FLATTEN -> FULLYCONNECTED Arguments: X -- input dataset placeholder, of shape (input size, number of examples) parameters -- python dictionary containing your parameters "W1", "W2" the shapes are given in initialize_parameters Returns: Z3 -- the output of the last LINEAR unit """ # Retrieve the parameters from the dictionary "parameters" W1 = parameters['W1'] W2 = parameters['W2'] ### START CODE HERE ### # CONV2D: stride of 1, padding 'SAME' Z1 = tf.nn.conv2d(X, W1, strides=[1, 1, 1, 1], padding='SAME') # RELU A1 = tf.nn.relu(Z1) # MAXPOOL: window 8x8, sride 8, padding 'SAME' P1 = tf.nn.max_pool(A1, ksize=[1, 8, 8, 1], strides=[1, 8, 8, 1], padding='SAME') # CONV2D: filters W2, stride 1, padding 'SAME' Z2 = tf.nn.conv2d(P1, W2, strides=[1, 1, 1, 1], padding='SAME') # RELU A2 = tf.nn.relu(Z2) # MAXPOOL: window 4x4, stride 4, padding 'SAME' P2 = tf.nn.max_pool(A2, ksize=[1, 4, 4, 1], strides=[1, 4, 4, 1], padding='SAME') # FLATTEN P2 = tf.contrib.layers.flatten(P2) # FULLY-CONNECTED without non-linear activation function (not not call softmax). # 6 neurons in output layer. Hint: one of the arguments should be "activation_fn=None" Z3 = tf.contrib.layers.fully_connected(P2, 6, activation_fn=None) ### END CODE HERE ### return Z3 tf.reset_default_graph() with tf.Session() as sess: np.random.seed(1) X, Y = create_placeholders(64, 64, 3, 6) parameters = initialize_parameters() Z3 = forward_propagation(X, parameters) init = tf.global_variables_initializer() sess.run(init) a = sess.run(Z3, {X: np.random.randn(2,64,64,3), Y: np.random.randn(2,6)}) print("Z3 = " + str(a)) 请根据现在python版本修改这段代码
这段代码是基于TensorFlow 1.x版本的,如果你使用的是TensorFlow 2.x版本,需要对代码进行一些修改。下面是修改后的代码:
```python
import tensorflow as tf
def forward_propagation(X, parameters):
"""
Implements the forward propagation for the model:
CONV2D -> RELU -> MAXPOOL -> CONV2D -> RELU -> MAXPOOL -> FLATTEN -> FULLYCONNECTED
Arguments:
X -- input dataset placeholder, of shape (input size, number of examples)
parameters -- python dictionary containing your parameters "W1", "W2"
the shapes are given in initialize_parameters
Returns:
Z3 -- the output of the last LINEAR unit
"""
# Retrieve the parameters from the dictionary "parameters"
W1 = parameters['W1']
W2 = parameters['W2']
# CONV2D: stride of 1, padding 'SAME'
Z1 = tf.nn.conv2d(X, W1, strides=[1, 1, 1, 1], padding='SAME')
# RELU
A1 = tf.nn.relu(Z1)
# MAXPOOL: window 8x8, sride 8, padding 'SAME'
P1 = tf.nn.max_pool2d(A1, ksize=[1, 8, 8, 1], strides=[1, 8, 8, 1], padding='SAME')
# CONV2D: filters W2, stride 1, padding 'SAME'
Z2 = tf.nn.conv2d(P1, W2, strides=[1, 1, 1, 1], padding='SAME')
# RELU
A2 = tf.nn.relu(Z2)
# MAXPOOL: window 4x4, stride 4, padding 'SAME'
P2 = tf.nn.max_pool2d(A2, ksize=[1, 4, 4, 1], strides=[1, 4, 4, 1], padding='SAME')
# FLATTEN
P2 = tf.reshape(P2, [-1, P2.shape[1] * P2.shape[2] * P2.shape[3]])
# FULLY-CONNECTED without non-linear activation function (not not call softmax).
# 6 neurons in output layer. Hint: one of the arguments should be "activation_fn=None"
Z3 = tf.contrib.layers.fully_connected(P2, 6, activation_fn=None)
return Z3
tf.random.set_seed(1)
with tf.compat.v1.Session() as sess:
tf.compat.v1.reset_default_graph()
X, Y = create_placeholders(64, 64, 3, 6)
parameters = initialize_parameters()
Z3 = forward_propagation(X, parameters)
init = tf.compat.v1.global_variables_initializer()
sess.run(init)
a = sess.run(Z3, {X: np.random.randn(2,64,64,3), Y: np.random.randn(2,6)})
print("Z3 = " + str(a))
```
这段代码已经修改为兼容TensorFlow 2.x版本,并进行了相应的调整。请注意,TensorFlow 2.x版本中的一些函数名称和用法可能与1.x版本有所不同。
相关推荐
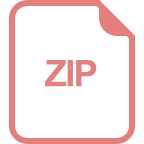
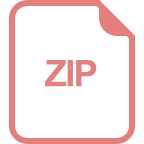
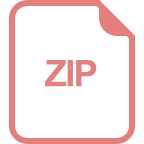













