resnet18+交叉注意力机制代码
时间: 2023-07-08 20:50:42 浏览: 264
以下是使用ResNet18和交叉注意力机制的代码示例:
```python
import torch
import torch.nn as nn
class CrossAttentionBlock(nn.Module):
def __init__(self, in_channels):
super(CrossAttentionBlock, self).__init__()
self.conv1 = nn.Conv2d(in_channels, in_channels, kernel_size=1, stride=1, padding=0)
self.conv2 = nn.Conv2d(in_channels, in_channels, kernel_size=1, stride=1, padding=0)
self.softmax = nn.Softmax(dim=-1)
def forward(self, x):
out = x
batch_size, channels, height, width = x.size()
# Global average pooling
f = x.mean(dim=-1).mean(dim=-1).unsqueeze(-1) # (batch_size, channels, 1)
# Query, key, value
q = self.conv1(x).view(batch_size, -1, width*height).transpose(1, 2) # (batch_size, width*height, channels)
k = self.conv2(x).view(batch_size, -1, width*height) # (batch_size, channels, width*height)
v = x.view(batch_size, channels, -1) # (batch_size, channels, width*height)
# Cross-Attention
qk = torch.bmm(q, k) # (batch_size, width*height, width*height)
a = self.softmax(qk) # (batch_size, width*height, width*height)
out = torch.bmm(v, a.transpose(1, 2)) # (batch_size, channels, width*height)
out = out.view(batch_size, channels, height, width)
# Residual connection
out = out + x
return out
class ResNet18_CrossAttention(nn.Module):
def __init__(self, num_classes=10):
super(ResNet18_CrossAttention, self).__init__()
self.conv1 = nn.Conv2d(3, 64, kernel_size=3, stride=1, padding=1, bias=False)
self.bn1 = nn.BatchNorm2d(64)
self.relu = nn.ReLU(inplace=True)
self.layer1 = nn.Sequential(
nn.Conv2d(64, 64, kernel_size=3, stride=1, padding=1, bias=False),
nn.BatchNorm2d(64),
nn.ReLU(inplace=True),
CrossAttentionBlock(64),
nn.Conv2d(64, 64, kernel_size=3, stride=1, padding=1, bias=False),
nn.BatchNorm2d(64),
nn.ReLU(inplace=True),
CrossAttentionBlock(64)
)
self.layer2 = nn.Sequential(
nn.Conv2d(64, 128, kernel_size=3, stride=2, padding=1, bias=False),
nn.BatchNorm2d(128),
nn.ReLU(inplace=True),
CrossAttentionBlock(128),
nn.Conv2d(128, 128, kernel_size=3, stride=1, padding=1, bias=False),
nn.BatchNorm2d(128),
nn.ReLU(inplace=True),
CrossAttentionBlock(128)
)
self.layer3 = nn.Sequential(
nn.Conv2d(128, 256, kernel_size=3, stride=2, padding=1, bias=False),
nn.BatchNorm2d(256),
nn.ReLU(inplace=True),
CrossAttentionBlock(256),
nn.Conv2d(256, 256, kernel_size=3, stride=1, padding=1, bias=False),
nn.BatchNorm2d(256),
nn.ReLU(inplace=True),
CrossAttentionBlock(256)
)
self.layer4 = nn.Sequential(
nn.Conv2d(256, 512, kernel_size=3, stride=2, padding=1, bias=False),
nn.BatchNorm2d(512),
nn.ReLU(inplace=True),
CrossAttentionBlock(512),
nn.Conv2d(512, 512, kernel_size=3, stride=1, padding=1, bias=False),
nn.BatchNorm2d(512),
nn.ReLU(inplace=True),
CrossAttentionBlock(512)
)
self.avgpool = nn.AdaptiveAvgPool2d((1, 1))
self.fc = nn.Linear(512, num_classes)
def forward(self, x):
x = self.conv1(x)
x = self.bn1(x)
x = self.relu(x)
x = self.layer1(x)
x = self.layer2(x)
x = self.layer3(x)
x = self.layer4(x)
x = self.avgpool(x)
x = x.view(x.size(0), -1)
x = self.fc(x)
return x
```
这里我们添加了一个名为CrossAttentionBlock的模块,它实现了交叉注意力机制,用于增强ResNet18的特征表示能力。在ResNet18_CrossAttention中,我们将CrossAttentionBlock插入到ResNet18的每个阶段中,以获得更强的特征表示能力。
阅读全文
相关推荐
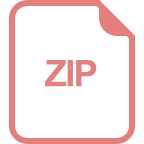
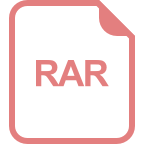
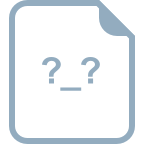
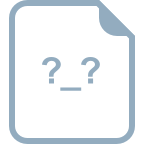
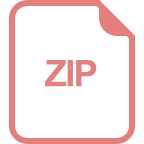
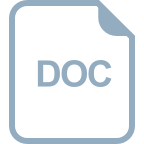
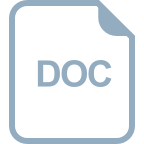
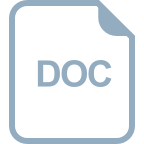
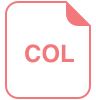
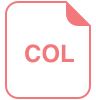
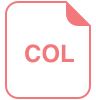
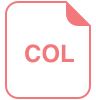
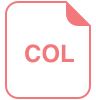
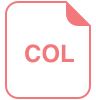
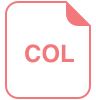
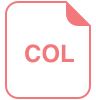
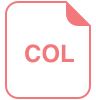
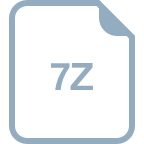
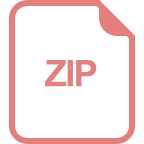