np.copy(np_image)
时间: 2023-11-27 11:03:18 浏览: 101
np.copy(np_image) 是什么意思?
这是一条关于Python编程的问题,np.copy() 是Numpy库中的一个函数,用于对数组进行深复制,即完全独立于原数组,不会对原数组造成影响。np_image 则是传入该函数的数组参数。
希望我能够对你的问题有所帮助!如果您还有其他问题,请继续提出。
相关问题
mask = np.array(Image.open(maskpath)) mask_copy = np.ones_like(mask, dtype=np.uint8) * 255 for clsID, trID in clsID_to_trID.items(): mask_copy[mask == clsID] = trID seg_filename = ( osp.join(out_mask_dir, "train2017" + suffix, osp.basename(maskpath)) if is_train else osp.join(out_mask_dir, "val2017" + suffix, osp.basename(maskpath)) ) if len(np.unique(mask_copy)) == 1 and np.unique(mask_copy)[0] == 255: return Image.fromarray(mask_copy).save(seg_filename, "PNG")
这段代码的功能是将给定的 mask 图像转换为训练时所需的 ID 标注图像,并将其保存为 PNG 格式。具体实现过程为首先读入 mask 图像,然后根据提供的 clsID_to_trID 字典将每个像素点的类别 ID 转换为对应的训练 ID,将处理后的图像保存到指定的文件路径。如果转换后的图像中只有一个像素值为 255,则直接返回。
这段代码什么意思def run_posmap_300W_LP(bfm, image_path, mat_path, save_folder, uv_h = 256, uv_w = 256, image_h = 256, image_w = 256): # 1. load image and fitted parameters image_name = image_path.strip().split('/')[-1] image = io.imread(image_path)/255. [h, w, c] = image.shape info = sio.loadmat(mat_path) pose_para = info['Pose_Para'].T.astype(np.float32) shape_para = info['Shape_Para'].astype(np.float32) exp_para = info['Exp_Para'].astype(np.float32) # 2. generate mesh # generate shape vertices = bfm.generate_vertices(shape_para, exp_para) # transform mesh s = pose_para[-1, 0] angles = pose_para[:3, 0] t = pose_para[3:6, 0] transformed_vertices = bfm.transform_3ddfa(vertices, s, angles, t) projected_vertices = transformed_vertices.copy() # using stantard camera & orth projection as in 3DDFA image_vertices = projected_vertices.copy() image_vertices[:,1] = h - image_vertices[:,1] - 1 # 3. crop image with key points kpt = image_vertices[bfm.kpt_ind, :].astype(np.int32) left = np.min(kpt[:, 0]) right = np.max(kpt[:, 0]) top = np.min(kpt[:, 1]) bottom = np.max(kpt[:, 1]) center = np.array([right - (right - left) / 2.0, bottom - (bottom - top) / 2.0]) old_size = (right - left + bottom - top)/2 size = int(old_size*1.5) # random pertube. you can change the numbers marg = old_size*0.1 t_x = np.random.rand()*marg*2 - marg t_y = np.random.rand()*marg*2 - marg center[0] = center[0]+t_x; center[1] = center[1]+t_y size = size*(np.random.rand()*0.2 + 0.9) # crop and record the transform parameters src_pts = np.array([[center[0]-size/2, center[1]-size/2], [center[0] - size/2, center[1]+size/2], [center[0]+size/2, center[1]-size/2]]) DST_PTS = np.array([[0, 0], [0, image_h - 1], [image_w - 1, 0]]) tform = skimage.transform.estimate_transform('similarity', src_pts, DST_PTS) cropped_image = skimage.transform.warp(image, tform.inverse, output_shape=(image_h, image_w)) # transform face position(image vertices) along with 2d facial image position = image_vertices.copy() position[:, 2] = 1 position = np.dot(position, tform.params.T) position[:, 2] = image_vertices[:, 2]*tform.params[0, 0] # scale z position[:, 2] = position[:, 2] - np.min(position[:, 2]) # translate z # 4. uv position map: render position in uv space uv_position_map = mesh.render.render_colors(uv_coords, bfm.full_triangles, position, uv_h, uv_w, c = 3) # 5. save files io.imsave('{}/{}'.format(save_folder, image_name), np.squeeze(cropped_image)) np.save('{}/{}'.format(save_folder, image_name.replace('jpg', 'npy')), uv_position_map) io.imsave('{}/{}'.format(save_folder, image_name.replace('.jpg', '_posmap.jpg')), (uv_position_map)/max(image_h, image_w)) # only for show # --verify # import cv2 # uv_texture_map_rec = cv2.remap(cropped_image, uv_position_map[:,:,:2].astype(np.float32), None, interpolation=cv2.INTER_LINEAR, borderMode=cv2.BORDER_CONSTANT,borderValue=(0)) # io.imsave('{}/{}'.format(save_folder, image_name.replace('.jpg', '_tex.jpg')), np.squeeze(uv_texture_map_rec))
这段代码是一个函数,用于将一张人脸图像进行三维重建并生成该人脸在二维图像上的 UV 位置图。具体步骤如下:
1. 加载人脸图像和拟合参数。
2. 生成人脸三维模型,并进行变换,得到变换后的人脸模型顶点位置。
3. 根据人脸关键点进行裁剪,得到人脸区域的图像,并记录变换参数。
4. 在 UV 空间中渲染人脸模型,并生成 UV 位置图。
5. 保存裁剪后的图像和 UV 位置图。
其中,变换参数包括旋转、平移、缩放等。UV 位置图是将人脸模型在 UV 空间中的位置映射到二维图像上的一张纹理图。
阅读全文
相关推荐
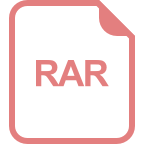
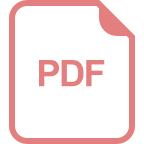
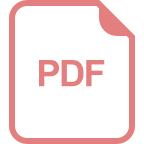













