``` indices = torch.randperm(len(dataset)).tolist() ```
时间: 2024-06-17 21:04:14 浏览: 189
这行代码是在PyTorch中使用随机打乱数据集的函数`randperm()`,将数据集中每个数据的索引打乱,并将其转换为Python中的列表形式。具体而言,`len(dataset)`返回数据集的长度,即数据集中数据的个数,`randperm()`函数将这些数据的索引打乱,返回一个大小为`len(dataset)`的一维张量,这个张量的每个元素是一个数据在数据集中的索引。最后,`.tolist()`将这个一维张量转换为Python中的列表形式,方便后续使用。整行代码的作用是用随机顺序打乱数据集中每个数据的索引。
相关问题
class DistributedSampler(_DistributedSampler): def __init__(self, dataset, num_replicas=None, rank=None, shuffle=True): super().__init__(dataset, num_replicas=num_replicas, rank=rank) self.shuffle = shuffle def __iter__(self): if self.shuffle: g = torch.Generator() g.manual_seed(self.epoch) indices = torch.randperm(len(self.dataset), generator=g).tolist() else: indices = torch.arange(len(self.dataset)).tolist() indices += indices[:(self.total_size - len(indices))] assert len(indices) == self.total_size indices = indices[self.rank:self.total_size:self.num_replicas] assert len(indices) == self.num_samples return iter(indices) def build_dataloader(dataset_cfg, class_names, batch_size, dist, root_path=None, workers=4, seed=None, logger=None, training=True, merge_all_iters_to_one_epoch=False, total_epochs=0): dataset = __all__[dataset_cfg.DATASET]( dataset_cfg=dataset_cfg, class_names=class_names, root_path=root_path, training=training, logger=logger, ) if merge_all_iters_to_one_epoch: assert hasattr(dataset, 'merge_all_iters_to_one_epoch') dataset.merge_all_iters_to_one_epoch(merge=True, epochs=total_epochs) if dist: if training: sampler = torch.utils.data.distributed.DistributedSampler(dataset) else: rank, world_size = common_utils.get_dist_info() sampler = DistributedSampler(dataset, world_size, rank, shuffle=False) else: sampler = None dataloader = DataLoader( dataset, batch_size=batch_size, pin_memory=True, num_workers=workers, shuffle=(sampler is None) and training, collate_fn=dataset.collate_batch, drop_last=False, sampler=sampler, timeout=0, worker_init_fn=partial(common_utils.worker_init_fn, seed=seed) ) return dataset, dataloader, sampler
这段代码是一个用于构建数据加载器的函数。它接受一些参数,包括数据集的配置、类别名称、批次大小、分布式训练标志、数据集的根路径等。
首先,根据数据集的配置和其他参数,创建一个数据集对象。
如果设置了 `merge_all_iters_to_one_epoch` 标志为 `True`,则调用数据集对象的 `merge_all_iters_to_one_epoch` 方法,将所有迭代器合并到一个周期中。
接下来,如果分布式训练标志为 `True`,则根据训练模式创建相应的采样器。对于训练模式,使用 `torch.utils.data.distributed.DistributedSampler` 创建采样器;对于非训练模式,根据当前进程的排名和世界大小创建 `DistributedSampler` 采样器,并设置 `shuffle` 参数为 `False`。
如果不是分布式训练,则采样器为 `None`。
最后,使用 `torch.utils.data.DataLoader` 创建数据加载器,传入数据集对象、批次大小、是否在训练模式下洗牌、数据集对象的 `collate_batch` 方法用于批量整理数据、是否丢弃最后一个批次、采样器以及其他参数。
函数返回数据集对象、数据加载器和采样器。
概述这段代码的作用: # Update image weights (optional) if opt.image_weights: # Generate indices if rank in [-1, 0]: cw = model.class_weights.cpu().numpy() * (1 - maps) ** 2 / nc # class weights iw = labels_to_image_weights(dataset.labels, nc=nc, class_weights=cw) # image weights dataset.indices = random.choices(range(dataset.n), weights=iw, k=dataset.n) # rand weighted idx # Broadcast if DDP if rank != -1: indices = (torch.tensor(dataset.indices) if rank == 0 else torch.zeros(dataset.n)).int() dist.broadcast(indices, 0) if rank != 0: dataset.indices = indices.cpu().numpy()
这段代码的作用是在训练神经网络时,根据图像的权重对图像进行采样,以提高模型对低频类别的分类准确性。具体实现方式是根据训练数据集中每个类别的权重,计算每个图像的权重,并将其用于随机采样。如果使用了分布式数据并行(DDP)训练,还会进行数据广播以确保每个进程使用相同的采样索引。这段代码中的具体实现细节可能会因为不同的神经网络结构、训练任务和数据集而略有不同。
阅读全文
相关推荐
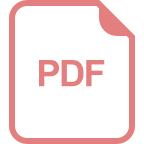
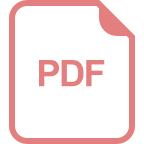













