python中note.shape
时间: 2024-06-08 07:06:42 浏览: 11
在Python中,`note.shape`通常不是一个有效的属性。如果你是指NumPy数组或者Pandas数据框的形状,你可以使用`.shape`属性来获取其维度。例如,对于一个NumPy数组`note`,你可以使用`note.shape`来获取其形状。如果你提供更多关于`note`的背景信息,我可以给你更详细的回答。
相关问题
python中knnimputer源代码
KNNImputer是sklearn.impute模块中的一个类,用于使用KNN算法对缺失值进行填充。以下是KNNImputer的源代码:
```python
import numpy as np
from scipy.spatial import cKDTree
from sklearn.impute import _base as base
from sklearn.utils.validation import check_array
from sklearn.utils.validation import check_is_fitted
class KNNImputer(base.BaseEstimator, base.TransformerMixin):
"""Impute missing values using k-Nearest Neighbors.
Parameters
----------
n_neighbors : int, default=5
Number of neighboring samples to use for imputation.
weights : {'uniform', 'distance'}, default='uniform'
Weight function used in prediction. Possible values:
- 'uniform' : uniform weights. All points in each neighborhood
are weighted equally.
- 'distance' : weight points by the inverse of their distance.
in this case, closer neighbors of a query point will have a
greater influence than neighbors which are further away.
metric : str or callable, default='nan_euclidean'
Distance metric to use. The default metric is 'nan_euclidean', which
is a modified version of 'euclidean' that supports missing values.
Possible values:
- From scikit-learn: ['cityblock', 'cosine', 'euclidean', 'l1', 'l2',
'manhattan']. These metrics support sparse matrix inputs.
- From scipy.spatial.distance: ['braycurtis', 'canberra', 'chebyshev',
'correlation', 'dice', 'hamming', 'jaccard', 'kulsinski',
'mahalanobis', 'matching', 'minkowski', 'rogerstanimoto',
'russellrao', 'seuclidean', 'sokalmichener', 'sokalsneath',
'sqeuclidean', 'yule']. These metrics do not support sparse
matrix inputs.
copy : bool, default=True
If True, a copy of X will be created. If False, imputation will be
done in-place whenever possible. Note that, in the following cases,
a new copy will always be created, even if copy=False:
- If X is not an array of floating values;
- If X is sparse and `missing_values=0`;
- If ``force_all_finite=True`` and X contains non-finite values.
add_indicator : bool, default=False
If True, an additional boolean feature is added for each feature
where missing values exist. The location of missing values is
indicated with ``True``. If ``use_cat_names=True`` and ``X`` is a
pandas DataFrame, the indicator feature names are derived from the
original feature names and appended with '_missing'.
If ``use_cat_names=True``, categorical features with missing values
will have an indicator feature created for each category.
missing_values : {np.nan, None, int, float}, default=np.nan
The placeholder for the missing values. All occurrences of
`missing_values` will be imputed. For missing values encoded as np.nan,
the `KNNImputer` assumes that the data is missing completely at random
(MCAR) and will always impute this value during prediction.
force_all_finite : bool, {'allow-nan', True}, default=True
Whether to raise an error on encountering non-finite values (``True``)
or just skip them (``allow-nan``). If ``allow-nan``, only missing
values will be imputed.
Notes
-----
NaNs are considered as missing values.
See also
--------
IterativeImputer : Multivariate imputation of missing values using
estimators with iterative training.
Examples
--------
>>> import numpy as np
>>> from sklearn.impute import KNNImputer
>>> X = np.array([[1, 2, np.nan], [3, 4, 3], [np.nan, 6, 5], [8, 8, 7]])
>>> imputer = KNNImputer(n_neighbors=2)
>>> imputer.fit_transform(X)
array([[1. , 2. , 4. ],
[3. , 4. , 3. ],
[5.5, 6. , 5. ],
[8. , 8. , 7. ]])
"""
def __init__(self, n_neighbors=5, weights="uniform",
metric="nan_euclidean", copy=True,
add_indicator=False, missing_values=np.nan,
force_all_finite=True):
self.n_neighbors = n_neighbors
self.weights = weights
self.metric = metric
self.copy = copy
self.add_indicator = add_indicator
self.missing_values = missing_values
self.force_all_finite = force_all_finite
def _more_tags(self):
return {'allow_nan': True}
def fit(self, X, y=None):
"""Fit the KNNImputer on X.
Parameters
----------
X : {array-like, sparse matrix} of shape (n_samples, n_features)
Input data.
Returns
-------
self : KNNImputer
"""
X = self._validate_data(X,
accept_sparse="csr",
dtype=[np.float64, np.float32],
force_all_finite=not self.add_indicator and
self.force_all_finite,
copy=self.copy)
n_samples, n_features = X.shape
if n_samples < self.n_neighbors:
raise ValueError("n_neighbors must be less than or equal to "
"the number of samples.")
if self.metric == "precomputed":
self.knn_.fit(X)
else:
self.tree_ = cKDTree(X, leafsize=30.,
metric=self.metric)
self._fit_X = X
if self.add_indicator:
self._indicator = np.zeros((n_samples, n_features),
dtype=bool)
return self
def transform(self, X):
"""Impute all missing values in X.
Parameters
----------
X : {array-like, sparse matrix} of shape (n_samples, n_features)
The input data to complete.
Returns
-------
X : {ndarray, sparse matrix} of shape (n_samples, n_features)
The imputed input data.
"""
check_is_fitted(self)
X = self._validate_data(X,
accept_sparse="csr",
dtype=[np.float64, np.float32],
reset=False,
copy=self.copy,
force_all_finite=self.force_all_finite)
n_samples, n_features = X.shape
if self.add_indicator:
if self._indicator is None:
self._indicator = np.zeros((n_samples, n_features),
dtype=bool)
else:
self._indicator.fill(False)
# Initialize imputed array to input X
X_imputed = X.copy()
# Get indices of missing and non-missing values
missing_mask = np.isnan(X)
n_missing = np.sum(missing_mask, axis=1)
n_non_missing = n_features - n_missing
# KNN imputation step
if np.any(missing_mask):
if self.metric == "precomputed":
X_imputed[missing_mask] = self.knn_.predict(X)[missing_mask]
else:
ind, dist = self.tree_.query(X[missing_mask],
k=self.n_neighbors)
# Compute weights
if self.weights == 'uniform':
weights = np.ones((self.n_neighbors,), dtype=X.dtype)
elif self.weights == 'distance':
# Prevent divide-by-zero errors
dist[dist == 0] = np.nextafter(0, 1)
weights = 1. / dist
# Normalize weights
weights_sum = np.sum(weights, axis=1)[:, np.newaxis]
weights /= weights_sum
# Compute imputed values
if self.add_indicator:
values_imputed = np.ma.array(
self._fit_X[ind],
mask=np.logical_not(missing_mask[:, np.newaxis]),
fill_value=self.missing_values
)
values_imputed.mask |= np.isnan(values_imputed.filled())
values_weighted = np.ma.average(
values_imputed, axis=1, weights=weights
).data
indicator_imputed = np.isnan(values_imputed.filled()).any(axis=1)
self._indicator[missing_mask] = indicator_imputed
else:
values_imputed = np.ma.array(
X_imputed[ind],
mask=np.logical_not(missing_mask[:, np.newaxis]),
fill_value=self.missing_values
)
values_imputed.mask |= np.isnan(values_imputed.filled())
values_weighted = np.ma.average(
values_imputed, axis=1, weights=weights
).data
X_imputed[missing_mask] = values_weighted
# Add indicator features
if self.add_indicator:
if isinstance(X_imputed, np.ndarray):
X_imputed = np.hstack([X_imputed, self._indicator])
else: # sparse matrix
from scipy.sparse import hstack
from scipy.sparse import csr_matrix
indicator_sparse = csr_matrix(self._indicator)
X_imputed = hstack([X_imputed, indicator_sparse])
return X_imputed
```
以上是KNNImputer的完整源代码。
Traceback (most recent call last): File "C:\Users\Adminis\Desktop\practice\颜色校正方法\main.py", line 61, in <module> img = auto_whiteBalance(img_data) File "C:\Users\Adminis\Desktop\practice\颜色校正方法\main.py", line 20, in auto_whiteBalance L_list = list(np.reshape(RL, (RL.shape[0] * RL.shape[1],)).astype(np.int)) File "C:\Users\Adminis\AppData\Local\Programs\Python\Python39\lib\site-packages\numpy\__init__.py", line 305, in __getattr__ raise AttributeError(__former_attrs__[attr]) AttributeError: module 'numpy' has no attribute 'int'. `np.int` was a deprecated alias for the builtin `int`. To avoid this error in existing code, use `int` by itself. Doing this will not modify any behavior and is safe. When replacing `np.int`, you may wish to use e.g. `np.int64` or `np.int32` to specify the precision. If you wish to review your current use, check the release note link for additional information. The aliases was originally deprecated in NumPy 1.20; for more details and guidance see the original release note at: https://numpy.org/devdocs/release/1.20.0-notes.html#deprecations
这个错误是由于您在代码中使用了`np.int`,而在新版本的NumPy中,`np.int`已被弃用。为了避免这个错误,您可以将`np.int`替换为Python内置的`int`。这样做不会改变任何行为并且是安全的。如果您希望指定精度,可以使用`np.int64`或`np.int32`等。您还可以查看NumPy的发布说明链接,了解更多关于这个问题的详细信息。
修改代码中的部分示例:
```python
L_list = list(np.reshape(RL, (RL.shape[0] * RL.shape[1],)).astype(int))
```
请尝试使用上述修改后再次运行代码,看看问题是否得到解决。如果问题仍然存在,请提供更多的代码和数据细节,以便我能够更好地帮助您解决问题。
相关推荐
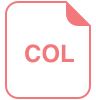
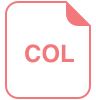
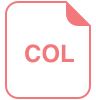
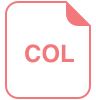
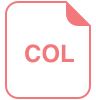







