cap_perframe(iter,:) = tx_bits(1,Mi+log2(Nt)+1:size(tx_bits,2));带有下标的赋值维度不匹配
时间: 2024-04-13 12:26:36 浏览: 194
在这段代码中,`cap_perframe(iter,:)` 的维度是与 `tx_bits(1,Mi+log2(Nt)+1:size(tx_bits,2))` 的维度不匹配。这意味着 `cap_perframe(iter,:)` 的大小与 `tx_bits(1,Mi+log2(Nt)+1:size(tx_bits,2))` 的大小不同。
要解决这个问题,你需要确保两个维度的大小相匹配。可能需要调整或修改代码,确保赋值操作的维度一致。你可以检查 `cap_perframe(iter,:)` 和 `tx_bits(1,Mi+log2(Nt)+1:size(tx_bits,2))` 的大小,确保它们具有相同的列数。
相关问题
优化代码 std::map<int, int> my_map; // 假设 my_map 是要分组的 std::map for(int i=0;i<1234;i++){ my_map[i] = i*2; } const int max_group_size = 200; // 每组最多有 200 个元素 // 将 std::map 转换为 std::vector std::vector<std::pair<int, int>> my_vector(my_map.begin(), my_map.end()); // 使用 std::partition 算法将元素分组 auto partition_iter = std::begin(my_vector); while (partition_iter != std::end(my_vector)) { auto next_partition_iter = std::next(partition_iter,max_group_size); if(next_partition_iter > std::end(my_vector)){ next_partition_iter = std::end(my_vector); } for(auto iter = partition_iter;iter != next_partition_iter;++iter){ std::cout << iter->first << " --> " << iter->second << std::endl; } std::cout << "======group end======" << std::endl; partition_iter = next_partition_iter; }
这段代码看起来已经很不错了,但是还有一些可以优化的地方:
1. 在 for 循环中使用 emplace_back() 而不是直接赋值,可以避免多余的拷贝操作。
2. 在 std::partition 中使用 lambda 表达式,可以避免定义额外的函数对象。
3. 在 std::next 中使用 std::min 可以避免超出边界的问题。
下面是优化后的代码:
```
std::map<int, int> my_map;
for (int i = 0; i < 1234; i++) {
my_map.emplace(i, i * 2);
}
const int max_group_size = 200;
std::vector<std::pair<int, int>> my_vector;
my_vector.reserve(my_map.size());
for (const auto& pair : my_map) {
my_vector.emplace_back(pair.first, pair.second);
}
auto partition_iter = std::begin(my_vector);
while (partition_iter != std::end(my_vector)) {
const auto next_partition_iter = std::next(partition_iter, std::min(max_group_size, static_cast<int>(std::distance(partition_iter, std::end(my_vector)))));
std::for_each(partition_iter, next_partition_iter, [](const auto& pair) {
std::cout << pair.first << " --> " << pair.second << '\n';
});
std::cout << "======group end======" << '\n';
partition_iter = next_partition_iter;
}
```
这样做的好处是减少了不必要的拷贝和函数调用,使代码更加优雅和高效。
解读这段代码class randomSequentialSampler(sampler.Sampler): def __init__(self, data_source, batch_size): self.num_samples = len(data_source) self.batch_size = batch_size def __iter__(self): n_batch = len(self) // self.batch_size tail = len(self) % self.batch_size index = torch.LongTensor(len(self)).fill_(0) for i in range(n_batch): random_start = random.randint(0, len(self) - self.batch_size) batch_index = random_start + torch.range(0, self.batch_size - 1) index[i * self.batch_size:(i + 1) * self.batch_size] = batch_index # deal with tail if tail: random_start = random.randint(0, len(self) - self.batch_size) tail_index = random_start + torch.range(0, tail - 1) index[(i + 1) * self.batch_size:] = tail_index return iter(index) def __len__(self): return self.num_samples
这是一个自定义的 PyTorch 数据集采样器,用于在训练神经网络时对数据进行随机采样。具体来说:
- `randomSequentialSampler` 继承了 PyTorch 中的 `sampler.Sampler` 类,实现了 `__init__`、`__iter__` 和 `__len__` 方法。
- `__init__` 方法初始化了数据集的大小和每个 batch 的大小。
- `__iter__` 方法返回一个迭代器,用于遍历所有的样本,其中:
- `n_batch` 表示数据集中 batch 的数量。
- `tail` 表示剩余样本数。
- `index` 是一个长度为数据集大小的 LongTensor,用于存放样本下标。
- 通过循环,对每个 batch 随机选择起始样本下标,并将 batch 中每个样本的下标存储到 `index` 中。
- 处理剩余的不足一整个 batch 的样本,方法同上。
- 返回一个迭代器,用于遍历 `index` 中的所有样本下标。
- `__len__` 方法返回数据集的大小。
这个采样器的作用是将数据集中的样本随机分成若干个 batch,每个 batch 的大小由用户指定,且每个 batch 中的样本顺序也是随机的。这种采样方式可以增加数据集的多样性,提高模型的泛化能力。
阅读全文
相关推荐
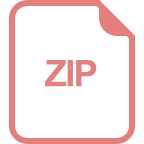
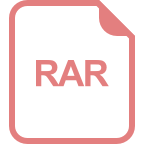










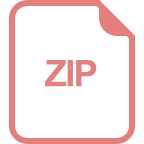
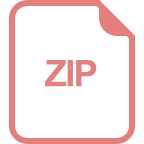

