python tcn
时间: 2023-11-02 10:03:25 浏览: 203
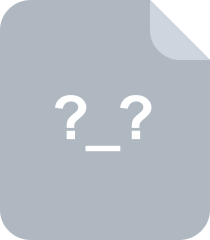
TCN.py

Python TCN是一个使用Python编写的时间序列预测库。TCN代表时序卷积网络,它是一种基于卷积神经网络的模型,用于处理时间序列数据。TCN可以有效地捕捉时间序列中的长期依赖关系,具有较好的预测性能。
TCN的特点是具有高度的并行性和灵活性。它使用一系列的卷积层和残差连接来构建模型。卷积层用于捕捉时间序列中的局部模式,而残差连接则用于解决梯度消失的问题,允许信息在不同的层间进行跳跃传递。这使得TCN能够有效地处理长期依赖关系,并且具有较好的模型表现。
在使用Python TCN进行时间序列预测时,首先需要准备好训练数据和测试数据。然后,可以使用Python TCN提供的API来构建TCN模型,并对训练数据进行拟合。在训练过程中,可以根据需要调整模型的超参数,如卷积核大小、卷积核数量等。拟合完成后,可以使用模型对测试数据进行预测,并评估模型的预测性能。
Python TCN还提供了一些辅助功能,如模型的保存和加载,以及对模型做进一步的调优。此外,Python TCN还支持多变量时间序列预测,可以同时处理多个时间序列的预测问题。
总之,Python TCN是一个强大的时间序列预测库,具有高效的模型结构和灵活的使用方式。它在处理长期依赖关系和多变量时间序列预测方面具有优势,可以帮助我们更好地理解和预测时间序列数据。
阅读全文
相关推荐

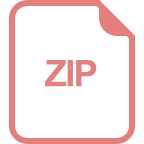
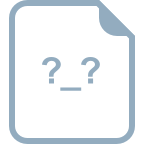
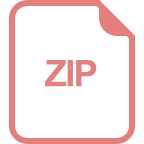











