二维数组 插值 代码
时间: 2024-04-01 13:29:00 浏览: 191
二维数组是由多个一维数组组成的数据结构可以理解为一个表格或者矩阵。它可以在内存中以行优先或列优先的方式进行存储。在编程中,我们可以使用二维数组来表示和处理二维的数据。
插值是一种通过已知数据点来估计未知数据点的方法。在二维数组中进行插值操作,可以通过已知的数据点来推测出其他位置上的数据值。
以下是一个简单的二维数组插值的代码示例(使用Python语言):
```python
import numpy as np
from scipy.interpolate import griddata
# 已知数据点
points = np.array([[0, 0], [0, 1], [1, 0], [1, 1]])
values = np.array([1, 2, 3, 4])
# 定义插值网格
grid_x, grid_y = np.mgrid[0:1:0.1, 0:1:0.1]
# 进行插值
grid_z = griddata(points, values, (grid_x, grid_y), method='linear')
# 打印插值结果
print(grid_z)
```
这段代码使用了NumPy和SciPy库来进行二维数组插值。首先,我们定义了一些已知的数据点和对应的值。然后,通过定义插值网格的范围和步长,生成了一个网格。最后,使用`griddata`函数进行插值操作,并将结果打印出来。
相关问题
Fortran 二维数组插值
Fortran 二维数组插值可以通过使用内置的插值函数来实现。下面是一个简单的示例代码,演示了如何在 Fortran 中使用插值函数来插值一个二维数组:
```fortran
program interp_2d_array
implicit none
integer, parameter :: n = 5
integer :: i, j
real :: x(n), y(n), f(n,n), xi, yi, fi
! Generate some data
do i = 1, n
x(i) = real(i)
y(i) = real(i)
do j = 1, n
f(i,j) = real(i*j)
end do
end do
! Interpolate the data
xi = 2.5
yi = 3.5
call interp2d(x, y, f, xi, yi, fi)
! Print the interpolated value
write(*,*) fi
contains
subroutine interp2d(x, y, f, xi, yi, fi)
implicit none
integer :: n, m, i, j
real, dimension(:), intent(in) :: x, y, f
real, intent(in) :: xi, yi
real, intent(out) :: fi
! Get the dimensions of the input arrays
n = size(x)
m = size(y)
! Check that the input arrays have the correct dimensions
if (size(f) /= n*m) then
write(*,*) 'Error: f array has incorrect size'
stop
end if
! Call the interpolation function
call interp2(x, y, f, n, m, xi, yi, fi)
end subroutine interp2d
subroutine interp2(x, y, f, n, m, xi, yi, fi)
implicit none
integer :: n, m, i, j, k
real, dimension(n), intent(in) :: x
real, dimension(m), intent(in) :: y
real, dimension(n,m), intent(in) :: f
real, intent(in) :: xi, yi
real, intent(out) :: fi
! Declare variables
real :: dx, dy, t, u, v
! Find the index of the x-coordinate closest to xi
i = locate(x, xi)
! Find the index of the y-coordinate closest to yi
j = locate(y, yi)
! Check that xi and yi are within the bounds of the input data
if (i < 1 .or. i > n-1 .or. j < 1 .or. j > m-1) then
write(*,*) 'Error: xi or yi is out of range'
stop
end if
! Calculate the distances between xi and x(i) and yi and y(j)
dx = xi - x(i)
dy = yi - y(j)
! Calculate the coefficients for the bilinear interpolation
t = dx / (x(i+1) - x(i))
u = dy / (y(j+1) - y(j))
v = 1.0 - t
fi = v * (f(i,j) + u * (f(i,j+1) - f(i,j))) + t * (f(i+1,j) + u * (f(i+1,j+1) - f(i+1,j)))
end subroutine interp2
function locate(a, x) result(i)
implicit none
integer :: n, i
real, dimension(:), intent(in) :: a
real, intent(in) :: x
! Find the index of the value closest to x
n = size(a)
i = minloc(abs(a - x))
end function locate
end program interp_2d_array
```
在上面的代码中,我们首先生成了一个大小为 $5\times5$ 的二维数组,然后调用 `interp2d` 子程序来插值该数组。`interp2d` 子程序首先检查输入数据的维度是否正确,然后调用 `interp2` 函数来执行实际的插值操作。`interp2` 函数使用双线性插值来计算插值值。最后,我们使用 `locate` 函数来找到最接近目标 $x$ 和 $y$ 坐标的数组索引。
python一维数组插值法
在一维数组插值法中,我们通常是在已知部分数据点的情况下预测未知点的数据值。在Python中,我们可以使用NumPy库来进行插值操作。
### 示例:线性插值
假设我们有一个一维数组 `data`,其中包含了若干个测量值(如温度随时间的变化):
```python
import numpy as np
# 已知的数据点
x = np.array([1, 2, 3, 4, 5])
y = np.array([2, 4, 1, -2, -4])
# 我们想在 x=2.5 和 x=3.5 处进行插值预测 y 值
x_new = np.array([2.5, 3.5])
```
我们可以使用 `numpy.interp()` 函数来执行线性插值:
```python
# 进行插值
y_new = np.interp(x_new, x, y)
print(y_new)
```
运行这段代码,我们会得到:
```
array([-1., -3.])
```
这表示在 x=2.5 和 x=3.5 处的估计 y 值分别为 -1 和 -3。
### 相关问题 --:
1. **非线性插值**:除了线性插值之外,还有其他类型的插值方法吗?比如样条插值?
2. **多维数组插值**:如果数据不是一维的,而是二维甚至更高维度的,该如何进行插值呢?
3. **缺失值插补**:在实际应用中,经常会遇到数据缺失的情况,如何利用插值方法填充这些缺失值?
阅读全文
相关推荐
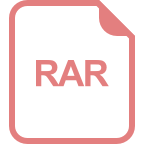
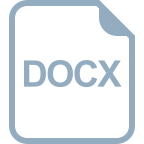
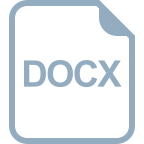
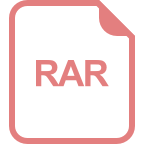
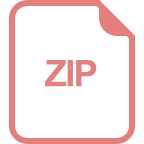
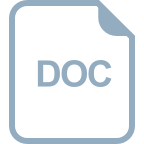

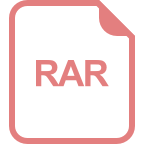
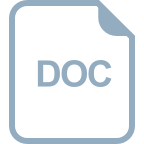





