这段代码是什么意思,是否有错 <view class="device_item"v-for="(item,index) in deviceList" :key="index" @click="devicechannel(item.deviceId)"> </view>
时间: 2023-11-29 10:05:17 浏览: 35
这段代码是一个在Vue.js中使用的模板语法,用于在页面中循环渲染一组设备项,并为每个设备项绑定一个点击事件。
让我逐行解释这段代码的含义:
- `<view class="device_item">`:这是一个`<view>`标签,使用`class="device_item"`来定义样式类,表示设备项的外层容器。
- `v-for="(item, index) in deviceList"`:这是循环渲染的语法,用于遍历`deviceList`数组中的每一项。`item`表示当前遍历到的数组元素,`index`表示当前元素在数组中的索引。
- `:key="index"`:这是为循环渲染的每个元素指定唯一的标识符,用于优化渲染效率。通常使用索引作为`key`。
- `@click="devicechannel(item.deviceId)"`:这是为设备项绑定点击事件的语法。当点击设备项时,会调用名为`devicechannel`的方法,并将`item.deviceId`作为参数传递给该方法。
至于是否有错,从您提供的代码来看,缺少了空格导致的错误。正确的代码应该为:
```html
<view class="device_item" v-for="(item, index) in deviceList" :key="index" @click="devicechannel(item.deviceId)">
<!-- 设备项内容 -->
</view>
```
请注意,在Vue.js中,模板中的属性和指令之间是需要有空格分隔的,否则会导致语法错误。确保添加了正确的空格后,再次运行代码,应该就不会报错了。
相关推荐
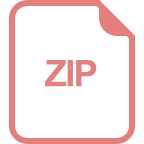
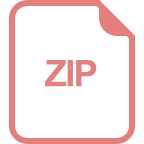
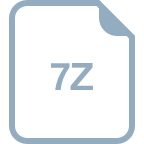














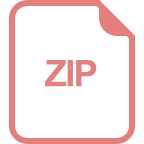