实现一个Linear层(torch.nn.Linear)功能,分别基于torch.nn.Module和torch.autograd.Function
时间: 2023-07-23 21:06:58 浏览: 253
好的,下面分别给出基于`torch.nn.Module`和`torch.autograd.Function`的实现示例。
基于`torch.nn.Module`的实现示例:
```python
import torch
import torch.nn as nn
class LinearLayer(nn.Module):
def __init__(self, input_size, output_size):
super(LinearLayer, self).__init__()
self.weight = nn.Parameter(torch.Tensor(output_size, input_size))
self.bias = nn.Parameter(torch.Tensor(output_size))
self.reset_parameters()
def reset_parameters(self):
nn.init.kaiming_uniform_(self.weight, a=math.sqrt(5))
if self.bias is not None:
fan_in, _ = nn.init._calculate_fan_in_and_fan_out(self.weight)
bound = 1 / math.sqrt(fan_in)
nn.init.uniform_(self.bias, -bound, bound)
def forward(self, input):
return torch.matmul(input, self.weight.t()) + self.bias
```
基于`torch.autograd.Function`的实现示例:
```python
import torch
from torch.autograd import Function
class LinearFunction(Function):
@staticmethod
def forward(ctx, input, weight, bias=None):
ctx.save_for_backward(input, weight, bias)
output = torch.matmul(input, weight.t())
if bias is not None:
output += bias.unsqueeze(0).expand_as(output)
return output
@staticmethod
def backward(ctx, grad_output):
input, weight, bias = ctx.saved_tensors
grad_input = grad_weight = grad_bias = None
if ctx.needs_input_grad[0]:
grad_input = torch.matmul(grad_output, weight)
if ctx.needs_input_grad[1]:
grad_weight = torch.matmul(grad_output.t(), input)
if bias is not None and ctx.needs_input_grad[2]:
grad_bias = grad_output.sum(0)
return grad_input, grad_weight, grad_bias
class LinearLayer(Function):
@staticmethod
def forward(ctx, input, weight, bias=None):
ctx.save_for_backward(input, weight, bias)
output = torch.matmul(input, weight.t())
if bias is not None:
output += bias.unsqueeze(0).expand_as(output)
return output
@staticmethod
def backward(ctx, grad_output):
input, weight, bias = ctx.saved_tensors
grad_input = grad_weight = grad_bias = None
if ctx.needs_input_grad[0]:
grad_input = torch.matmul(grad_output, weight)
if ctx.needs_input_grad[1]:
grad_weight = torch.matmul(grad_output.t(), input)
if bias is not None and ctx.needs_input_grad[2]:
grad_bias = grad_output.sum(0)
return grad_input, grad_weight, grad_bias
```
这两个示例分别基于`torch.nn.Module`和`torch.autograd.Function`实现了一个Linear层。你可以根据需要选择其中一种实现方式。希望对你有所帮助!
阅读全文
相关推荐
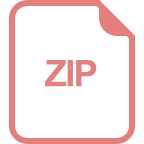
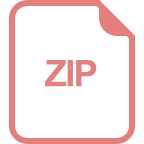
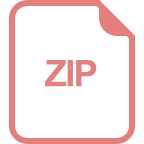
















