python 代码使用Louvain算法进行有向网络的社团划分
时间: 2024-12-15 09:30:45 浏览: 14
在Python中,可以使用`networkx`库结合`community`模块中的`louvain`函数来执行Louvain算法对有向网络进行社区划分。Louvain算法是一种基于模ularity优化的无标度社区检测方法,它将网络分解成各个紧密连接的小社区。
下面是一个简单的例子,假设你已经安装了`networkx`和`community`库:
```python
import networkx as nx
from community import louvain
# 创建一个有向网络示例
G = nx.DiGraph() # 或者使用nx.DiGraph()
G.add_edges_from([(0, 1), (1, 2), (2, 3), (3, 4), (4, 5), (5, 0)]) # 添加边
# 初始化并运行Louvain算法
partition = louvain.best_partition(G)
# 打印每个节点所属的社团
for node, label in partition.items():
print(f"Node {node} belongs to community {label}")
# 获取社团信息
communities = [nodes for nodes in nx.connected_components(G.subgraph(partition.values()))]
print("Communities:", communities)
相关问题
请帮我写一个代码,我有一份节点城市之间的两两出行量数据,是一个有向加权网络,我希望使用 Louvain 算法对这个网络进行社团划分,并对划分结果进行网络可视化,将不同社团的节点设置成不同颜色,最后在全国地图上进行地理可视化
首先,为了完成这项任务,你需要使用Python结合一些库,如NetworkX用于处理图数据、Louvain算法的实现如igraph或者community模块、以及matplotlib和geopandas进行可视化。这里提供一个简化版的步骤:
```python
import pandas as pd
import networkx as nx
from igraph import Graph
import community
import matplotlib.pyplot as plt
import geopandas as gpd
# 假设你的数据存储在一个CSV文件中,例如data.csv
data = pd.read_csv('data.csv')
# 创建有向加权网络
G = nx.DiGraph()
for _, row in data.iterrows():
source = row['Source City']
destination = row['Destination City']
weight = row['Travel Quantity']
G.add_edge(source, destination, weight=weight)
# 使用Louvain算法进行社团划分
partition = community.best_partition(G)
# 绘制网络,不同社团的颜色对应于分区
node_colors = ['red' if partition[node] == i else 'blue' for i, node in enumerate(G.nodes)]
pos = nx.spring_layout(G)
nx.draw_networkx_nodes(G, pos, nodelist=list(G), node_color=node_colors, cmap='Set1')
nx.draw_networkx_edges(G, pos, alpha=0.5)
plt.show()
# 地理可视化(假设你已经有了包含城市经纬度的geojson文件)
city_gdf = gpd.read_file('cities.geojson')
city_gdf['Community'] = partition
fig, ax = plt.subplots(figsize=(10, 10))
ax.set_title("City Map with Communities")
city_gdf.plot(column='Community', categorical=True, legend=True, linewidth=0.8, ax=ax)
plt.show()
```
请注意,这只是一个基本框架,实际应用中可能需要处理缺失的数据、错误边缘等细节,并可能需要调整代码以适应你的具体需求。此外,对于全国范围的地图,可能需要从外部获取地理信息服务(GIS)或者使用像folium这样的库来进行更复杂的交互式地图展示。
请帮我写一个代码,我有一个城市和城市之间的出行量数据,是一个有向加权网络,我希望使用 Louvain 算法对这个网络进行社团划分,并对划分结果进行网络可视化,将不同社团的节点设置成不同颜色,最后在全国地图上进行地理可视化
首先,我们需要安装必要的库,如 NetworkX (用于创建和操作图)、igraph (Louvain算法的一个强大实现) 和 geopandas (地理空间数据处理)。以下是一个简单的步骤示例:
1. 安装所需库:
```bash
pip install networkx igraph geopandas matplotlib
```
2. 导入并读取数据(这里假设数据是以CSV格式存储的,包含城市名、目的地、出行量和经度纬度信息):
```python
import pandas as pd
import numpy as np
data = pd.read_csv('city_travel_data.csv') # 替换为您实际的文件路径
```
3. 创建NetworkX图:
```python
import networkx as nx
# 将出行数据转换为边和权重
G = nx.DiGraph()
for _, row in data.iterrows():
source_city = row['City']
destinations = [row['Destination']]
weights = [row['Travel Quantity']] * len(destinations)
for dest, weight in zip(destinations, weights):
G.add_edge(source_city, dest, weight=weight)
```
4. 使用Louvain算法划分社团:
```python
from igraph import Graph
import community
# 转换成iGraph图并运行Louvain算法
ig = Graph(G.to_undirected(), edge_attrs=['weight'])
partition = community.best_partition(ig, weight='weight')
```
5. 将社团标签应用到原图上:
```python
nx.set_node_attributes(G, 'community', partition)
```
6. 可视化网络:
```python
import matplotlib.pyplot as plt
plt.figure(figsize=(10, 8))
pos = nx.spring_layout(G, with_labels=True)
colors = [G.nodes[node]['community'] for node in G.nodes]
nx.draw_networkx_nodes(G, pos, cmap=plt.cm.get_cmap('viridis', max(partition.values()) + 1), node_color=colors)
nx.draw_networkx_edges(G, pos, width=nx.get_edge_attributes(G, 'weight').values() / 10, alpha=0.7)
plt.title("City Travel Network with Louvain Clustering")
plt.show()
```
7. 地理可视化:
```python
import geopandas as gpd
from shapely.geometry import Point
# 假设有一个全国城市的GeoDataFrame gdf_cities
gdf_cities = ... # 加载全国城市的地理信息
G_gdf = gpd.GeoDataFrame(geometry=[Point(xy) for xy in list(G.nodes(data='pos'))], columns=['geometry'])
G_gdf['community'] = G.nodes(data='community')
# 绘制地图并将社团用不同颜色显示
ax = gdf_cities.plot(column='community', cmap='viridis', legend=True)
G_gdf.plot(ax=ax, markersize=5, color='black', marker='o')
plt.show()
```
注意:这只是一个基本示例,实际操作中可能需要根据数据格式进行调整,也可能需要使用其他库来提高性能或处理特定的地图可视化需求。请确保您的数据已准备好并且格式正确。另外,对于大规模地理数据,地理空间分析可能会非常耗时,可能需要考虑使用分布式计算或专门的地理信息系统工具。
阅读全文
相关推荐
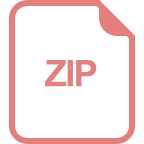
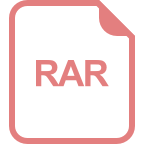
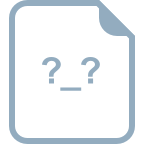













