for h in range(a2015): g=e[h].sum() if g >=10: y_label.append(1) else: y_label.append(0)是什么意思
时间: 2024-04-01 08:34:21 浏览: 37
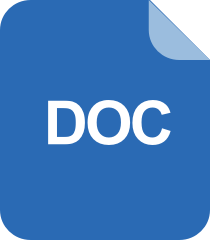
oracle8i&9i&10g参考手册
这段代码是一个Python的循环语句,其中a2015是一个变量,循环的次数为a2015的值。在每次循环中,代码会计算e[h]的元素之和,并将结果赋值给变量g。接着,如果g的值大于等于10,则向列表y_label中添加数字1,否则添加数字0。这段代码的作用是将e列表中的多个子列表的元素求和,然后根据求和结果是否大于等于10,将0或1添加到y_label列表中。
阅读全文
相关推荐
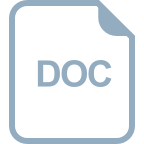
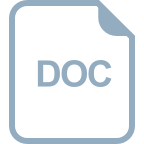
















