LSTM dimension
时间: 2023-11-07 13:48:54 浏览: 42
维度(dimension)是指在LSTM(长短期记忆网络)中,输入数据的维度或者大小。在引用中提到了维度混洗层(dimension shuffle layer),它是将时间序列输入进行维度改组,以提高训练效率。当使用不带维度改组的LSTM处理具有N个时间步长和M个变量的数据集时,LSTM需要N个时间步长来处理一批M个变量。而通过应用维度改组,可以使LSTM模型以M个时间步长处理一批N个变量。这意味着,只要变量数M显着小于时间步长N,维度改组将大大提高训练速度。
因此,在LSTM中的维度(dimension)是指输入数据的大小或维度,它的改组可以对模型的训练速度产生重要影响。
相关问题
双重注意力机制LSTM代码
以下是一个使用双重注意力机制LSTM的代码示例:
```python
import torch
import torch.nn as nn
class DualAttentionLSTM(nn.Module):
def __init__(self, input_size, hidden_size):
super(DualAttentionLSTM, self).__init__()
self.hidden_size = hidden_size
self.lstm = nn.LSTM(input_size, hidden_size)
self.time_attention = nn.Linear(hidden_size, 1)
self.element_attention = nn.Linear(hidden_size, 1)
def forward(self, inputs):
# inputs shape: (sequence_length, batch_size, input_size)
outputs, hidden = self.lstm(inputs)
time_weights = torch.softmax(self.time_attention(outputs), dim=0)
element_weights = torch.softmax(self.element_attention(outputs), dim=1)
# shape of time_weights: (sequence_length, batch_size, 1)
# shape of element_weights: (sequence_length, batch_size, hidden_size, 1)
weighted_outputs = outputs * time_weights * element_weights.squeeze(3)
# sum over the sequence length dimension
final_outputs = torch.sum(weighted_outputs, dim=0)
return final_outputs
```
这个模型使用了PyTorch框架,包括一个LSTM层和两个线性层,用于计算时间注意力和元素注意力。在前向传播中,输入序列通过LSTM层,然后通过时间注意力和元素注意力层进行加权。最后,将加权的输出相加并返回最终输出。
写一段BiLSTM的代码
Sure, here's an example of BiLSTM code using PyTorch:
```python
import torch
import torch.nn as nn
class BiLSTM(nn.Module):
def __init__(self, input_dim, hidden_dim, output_dim):
super(BiLSTM, self).__init__()
self.hidden_dim = hidden_dim
self.lstm = nn.LSTM(input_dim, hidden_dim, bidirectional=True)
self.fc = nn.Linear(hidden_dim * 2, output_dim) # *2 for bidirectional
def forward(self, x):
lstm_out, _ = self.lstm(x)
out = self.fc(lstm_out[:, -1, :]) # take the last time step
return out
```
In this code snippet, we define a `BiLSTM` class which inherits from `nn.Module`. The constructor takes the input dimension, hidden dimension, and output dimension as arguments. Inside the constructor, we define the layers of the network: an LSTM layer and a fully connected layer.
In the `forward` method, we pass the input `x` through the LSTM layer. The output of the LSTM is then passed through the fully connected layer, and the final output is returned.
Note that in this example, we assume the input `x` has a shape of `(sequence_length, batch_size, input_dim)`. The output will have a shape of `(batch_size, output_dim)`.
I hope this helps! Let me know if you have any more questions.
相关推荐
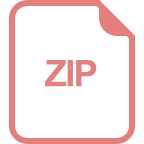












