data = pd.read_json(r'D:\pycharm\douban_film.txt', lines=True)作用
时间: 2024-04-07 07:31:24 浏览: 172
这行代码的作用是读取位于`D:\pycharm\douban_film.txt`文件中的JSON格式数据,并将其转换为Pandas DataFrame格式。其中,`lines=True`参数表示将文件中的每一行看作独立的JSON对象进行解析。
具体来说,当我们使用`read_json()`函数读取JSON格式数据时,可以设置多种参数来控制读取方式和解析方式。其中,`lines`参数是最常用的参数之一,其默认值为`False`,表示JSON数据是整体存储在一个文件中的,可以直接使用`read_json()`函数进行读取。但是,当JSON数据是一行一行地存储在文件中时,我们需要将`lines`参数设置为`True`,表示将每一行看作一个独立的JSON对象进行解析。
在这个例子中,我们可以假设`D:\pycharm\douban_film.txt`文件中存储了多个JSON对象,每个对象表示一部电影的相关信息,例如电影名称、评分、导演等。通过设置`lines=True`参数,`read_json()`函数会自动将每一行解析为一个JSON对象,并将多个JSON对象合并成一个Pandas DataFrame对象,方便我们进行数据分析和处理。
相关问题
dataset = pd.read_csv('D:\\PycharmProject\\Residual life prediction-SPD\\data_set\\2-train.csv',parse_dates=['Date'],index_col=[0])
这一段 Python 代码,使用 pandas 库中的 read_csv() 函数来读取名为 "2-train.csv" 的文件,并将其解析为一个 pandas DataFrame 对象。同时,它将 "Date" 列作为日期类型来解析,并将其设置为 DataFrame 的索引列。这段代码的前提条件是,你需要将 "2-train.csv" 文件放在 D:\PycharmProject\Residual life prediction-SPD\data_set 目录下。
在下面代码中添加一个可视化图,用来画出r经过t_sne之后前15行数据的图 import pandas as pd from sklearn import cluster from sklearn import metrics import matplotlib.pyplot as plt from sklearn.manifold import TSNE from sklearn.decomposition import PCA def k_means(data_set, output_file, png_file, png_file1, t_labels, score_file, set_name): model = cluster.KMeans(n_clusters=7, max_iter=1000, init="k-means++") model.fit(data_set) # print(list(model.labels_)) p_labels = list(model.labels_) r = pd.concat([data_set, pd.Series(model.labels_, index=data_set.index)], axis=1) r.columns = list(data_set.columns) + [u'聚类类别'] print(r) # r.to_excel(output_file) with open(score_file, "a") as sf: sf.write("By k-means, the f-m_score of " + set_name + " is: " + str(metrics.fowlkes_mallows_score(t_labels, p_labels))+"\n") sf.write("By k-means, the rand_score of " + set_name + " is: " + str(metrics.adjusted_rand_score(t_labels, p_labels))+"\n") '''pca = PCA(n_components=2) pca.fit(data_set) pca_result = pca.transform(data_set) t_sne = pd.DataFrame(pca_result, index=data_set.index)''' t_sne = TSNE() t_sne.fit(data_set) t_sne = pd.DataFrame(t_sne.embedding_, index=data_set.index) plt.rcParams['font.sans-serif'] = ['SimHei'] plt.rcParams['axes.unicode_minus'] = False dd = t_sne[r[u'聚类类别'] == 0] plt.plot(dd[0], dd[1], 'r.') dd = t_sne[r[u'聚类类别'] == 1] plt.plot(dd[0], dd[1], 'go') dd = t_sne[r[u'聚类类别'] == 2] plt.plot(dd[0], dd[1], 'b*') dd = t_sne[r[u'聚类类别'] == 3] plt.plot(dd[0], dd[1], 'o') dd = t_sne[r[u'聚类类别'] == 4] plt.plot(dd[0], dd[1], 'm.') dd = t_sne[r[u'聚类类别'] == 5] plt.plot(dd[0], dd[1], 'co') dd = t_sne[r[u'聚类类别'] == 6] plt.plot(dd[0], dd[1], 'y*') plt.savefig(png_file) '''plt.scatter(data_set.iloc[:, 0], data_set.iloc[:, 1], c=model.labels_) plt.savefig(png_file) plt.clf()''' frog_data = pd.read_csv("D:/PyCharmPython/pythonProject/mfcc3.csv") tLabel = [] for family in frog_data['name']: if family == "A": tLabel.append(0) elif family == "B": tLabel.append(1) elif family == "C": tLabel.append(2) elif family == "D": tLabel.append(3) elif family == "E": tLabel.append(4) elif family == "F": tLabel.append(5) elif family == "G": tLabel.append(6) scoreFile = "D:/PyCharmPython/pythonProject/scoreOfClustering.txt" first_set = frog_data.iloc[:, 1:1327] k_means(first_set, "D:/PyCharmPython/pythonProject/kMeansSet_1.xlsx", "D:/PyCharmPython/pythonProject/kMeansSet_2.png", "D:/PyCharmPython/pythonProject/kMeansSet_2_1.png", tLabel, scoreFile, "Set_1")
你可以在以下代码中添加一个可视化图来画出r经过t_sne之后前15行数据的图:
```python
import pandas as pd
from sklearn import cluster
from sklearn import metrics
import matplotlib.pyplot as plt
from sklearn.manifold import TSNE
from sklearn.decomposition import PCA
def k_means(data_set, output_file, png_file, png_file1, t_labels, score_file, set_name):
model = cluster.KMeans(n_clusters=7, max_iter=1000, init="k-means++")
model.fit(data_set)
p_labels = list(model.labels_)
r = pd.concat([data_set, pd.Series(model.labels_, index=data_set.index)], axis=1)
r.columns = list(data_set.columns) + [u'聚类类别']
print(r)
with open(score_file, "a") as sf:
sf.write("By k-means, the f-m_score of " + set_name + " is: " + str(metrics.fowlkes_mallows_score(t_labels, p_labels))+"\n")
sf.write("By k-means, the rand_score of " + set_name + " is: " + str(metrics.adjusted_rand_score(t_labels, p_labels))+"\n")
t_sne = TSNE()
t_sne.fit(data_set)
t_sne = pd.DataFrame(t_sne.embedding_, index=data_set.index)
plt.rcParams['font.sans-serif'] = ['SimHei']
plt.rcParams['axes.unicode_minus'] = False
dd = t_sne[r[u'聚类类别'] == 0]
plt.plot(dd[0], dd[1], 'r.')
dd = t_sne[r[u'聚类类别'] == 1]
plt.plot(dd[0], dd[1], 'go')
dd = t_sne[r[u'聚类类别'] == 2]
plt.plot(dd[0], dd[1], 'b*')
dd = t_sne[r[u'聚类类别'] == 3]
plt.plot(dd[0], dd[1], 'o')
dd = t_sne[r[u'聚类类别'] == 4]
plt.plot(dd[0], dd[1], 'm.')
dd = t_sne[r[u'聚类类别'] == 5]
plt.plot(dd[0], dd[1], 'co')
dd = t_sne[r[u'聚类类别'] == 6]
plt.plot(dd[0], dd[1], 'y*')
plt.savefig(png_file)
frog_data = pd.read_csv("D:/PyCharmPython/pythonProject/mfcc3.csv")
tLabel = []
for family in frog_data['name']:
if family == "A":
tLabel.append(0)
elif family == "B":
tLabel.append(1)
elif family == "C":
tLabel.append(2)
elif family == "D":
tLabel.append(3)
elif family == "E":
tLabel.append(4)
elif family == "F":
tLabel.append(5)
elif family == "G":
tLabel.append(6)
scoreFile = "D:/PyCharmPython/pythonProject/scoreOfClustering.txt"
first_set = frog_data.iloc[:, 1:1327]
k_means(first_set, "D:/PyCharmPython/pythonProject/kMeansSet_1.xlsx", "D:/PyCharmPython/pythonProject/kMeansSet_2.png", "D:/PyCharmPython/pythonProject/kMeansSet_2_1.png", tLabel, scoreFile, "Set_1")
```
请确保你已经安装了所需的库,并且将文件路径修改为你实际的文件路径。这段代码会将数据集进行聚类,并使用t-SNE进行降维,然后绘制出前15行数据的聚类可视化图。保存的图像将会保存在`png_file`路径下。
阅读全文
相关推荐
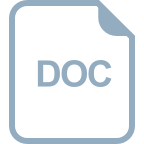
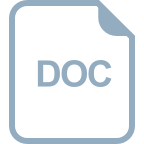
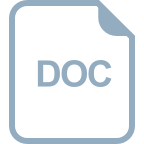








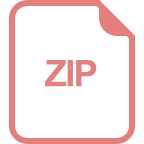




