怎么对.txt文件里的评论进行情感分析python
时间: 2024-05-16 14:15:35 浏览: 104
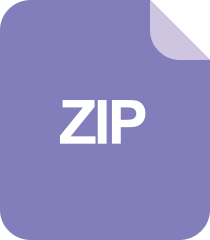
【项目实战】Python实现基于LDA主题模型进行电商产品评论数据情感分析

要进行情感分析,你需要使用自然语言处理(NLP)和机器学习技术。下面是一些步骤来对.txt文件里的评论进行情感分析:
1. 导入所需的Python库,例如`nltk`、`pandas`、`numpy`、`sklearn`和`matplotlib`。
2. 读取.txt文件并将其转换为Pandas dataframe。
3. 清理文本数据,例如去除标点符号和停用词,并将文本转换为小写。
4. 对文本数据进行标记化和词形还原。
5. 使用情感词典,例如`nltk`的`vader_lexicon`或`AFINN`,对每个评论进行情感分析得分。
6. 将情感分析得分添加到Pandas dataframe中。
7. 可以使用一些可视化工具,例如Matplotlib或Seaborn,来绘制评论情感得分的分布图。
下面是一个示例代码,其中使用了nltk的vader_lexicon情感词典进行情感分析:
```python
import pandas as pd
import numpy as np
import nltk
nltk.download('vader_lexicon')
from nltk.sentiment.vader import SentimentIntensityAnalyzer
# 读取txt文件
with open('comments.txt', 'r', encoding='utf-8') as f:
data = f.readlines()
# 转换为Pandas dataframe
df = pd.DataFrame(data, columns=['comment'])
# 清理文本数据
df['comment'] = df['comment'].str.replace('[^\w\s]','')
stopwords = nltk.corpus.stopwords.words('english')
df['comment'] = df['comment'].apply(lambda x: ' '.join([word.lower() for word in x.split() if word.lower() not in stopwords]))
# 标记化和词形还原
from nltk.tokenize import word_tokenize
from nltk.stem import WordNetLemmatizer
nltk.download('punkt')
nltk.download('wordnet')
lemmatizer = WordNetLemmatizer()
df['comment'] = df['comment'].apply(lambda x: ' '.join([lemmatizer.lemmatize(word) for word in word_tokenize(x)]))
# 情感分析
sia = SentimentIntensityAnalyzer()
df['sentiment_score'] = df['comment'].apply(lambda x: sia.polarity_scores(x)['compound'])
# 可视化
import matplotlib.pyplot as plt
import seaborn as sns
sns.histplot(data=df, x='sentiment_score', bins=10, kde=True)
plt.show()
```
这将生成一个情感分数分布的直方图。您可以根据需要进行调整和扩展。
阅读全文
相关推荐
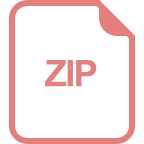
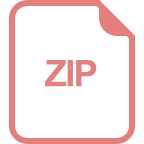















