Q = tf.layers.dense(queries, numUnits, activation=tf.nn.relu)在新版本的tensorflow中应该怎么写
时间: 2024-02-18 14:58:58 浏览: 28
在新版本的 TensorFlow 中,`tf.layers` 子模块已被弃用,其功能被转移到 `tf.keras.layers` 中。因此,您可以使用 `tf.keras.layers.Dense` 函数来替换 `tf.layers.dense`。
以下是使用 `tf.keras.layers.Dense` 函数的示例代码:
```python
Q = tf.keras.layers.Dense(units=numUnits, activation=tf.nn.relu)(queries)
```
这里,我们首先将 `numUnits` 作为 `units` 参数传递给 `tf.keras.layers.Dense` 函数,将 `tf.nn.relu` 作为 `activation` 参数传递给该函数,然后将 `queries` 作为输入张量传递给该函数。`tf.keras.layers.Dense` 的输出是一个张量,可以通过调用该函数并传递输入张量来获取它。
注意,如果您需要在 `tf.keras.layers.Dense` 函数之后添加其他层,请将每个层作为函数调用的一部分传递,就像上面的示例代码一样。
相关问题
Q = tf.layers.dense(inputs=Q, units=units, activation=tf.nn.relu) AttributeError: module 'tensorflow' has no attribute 'layers'
这个错误通常是因为使用的 TensorFlow 版本太低导致的。在较旧的 TensorFlow 版本中,`layers` 模块不是 TensorFlow 的一部分,而是一个单独的模块。
为了解决这个问题,您可以升级 TensorFlow 到最新版本。如果您已经使用了最新版本的 TensorFlow,那么您的代码可能存在其他问题,需要进一步调试。
Q = tf.keras.layers.Dense(queries, numUnits,activation='relu') # [64,10,300] TypeError: __init__() got multiple values for argument 'activation'
在使用 `tf.keras.layers.Dense()` 函数时,第一个参数应该是输出维度(即 `units`),第二个参数是输入维度(即 `input_shape` 或 `input_dim`),而不是输入张量。因此,您需要将 `queries` 作为 `input_shape` 或 `input_dim` 参数传递,而将 `numUnits` 作为 `units` 参数传递。
另外,`activation` 参数只能传递一个值,您可能在函数调用中重复传递了该参数。请检查您的代码并确保只有一个 `activation` 参数传递给 `tf.keras.layers.Dense()` 函数。
以下是使用正确参数调用 `tf.keras.layers.Dense()` 函数的示例代码:
```python
Q = tf.keras.layers.Dense(units=numUnits, activation='relu', input_shape=(queries,))
```
这应该能够解决您遇到的错误。
相关推荐
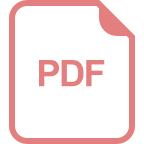
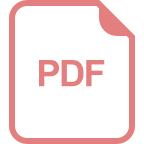
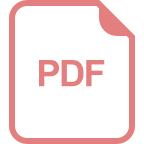













