python 张量所有元素求和
时间: 2023-09-05 18:14:23 浏览: 113
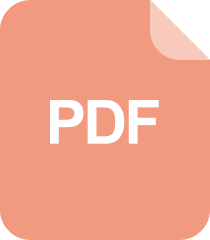
Python之list对应元素求和的方法
在Python中,可以使用NumPy或TensorFlow等库来进行张量的操作。以下是两种方法来对张量中的所有元素求和:
使用NumPy:
```python
import numpy as np
# 创建一个2x3的张量
x = np.array([[1, 2, 3], [4, 5, 6]])
# 对所有元素求和
sum_x = np.sum(x)
print(sum_x) # 输出: 21
```
使用TensorFlow:
```python
import tensorflow as tf
# 创建一个2x3的张量
x = tf.constant([[1, 2, 3], [4, 5, 6]])
# 对所有元素求和
sum_x = tf.reduce_sum(x)
with tf.Session() as sess:
print(sess.run(sum_x)) # 输出: 21
```
以上两种方法都可以对张量中的所有元素进行求和操作。其中,NumPy是一个通用的数学库,可以用于大多数数学操作,而TensorFlow则专注于张量计算和机器学习。
阅读全文
相关推荐
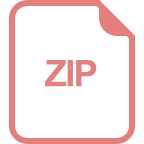
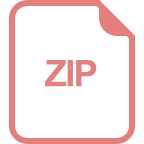
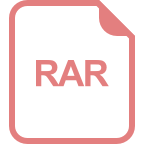
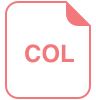
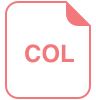
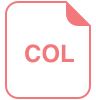
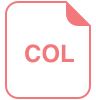








