线性规划求解tsp问题python
时间: 2023-08-24 10:09:56 浏览: 82
要使用线性规划来求解TSP问题,你可以使用Python的优化库,如PuLP或cvxpy。下面是一个使用PuLP库的例子:
```python
from pulp import *
# 创建问题实例
prob = LpProblem("TSP_Problem", LpMinimize)
# 创建变量
n = 4 # 城市数量
cities = range(n) # 城市列表
x = LpVariable.dicts("x", [(i, j) for i in cities for j in cities], cat='Binary')
# 添加目标函数
prob += lpSum(x[i, j] * distance_matrix[i][j] for i in cities for j in cities)
# 添加约束条件
for i in cities:
prob += lpSum(x[i, j] for j in cities if j != i) == 1
prob += lpSum(x[j, i] for j in cities if j != i) == 1
# 避免出现子回路
u = LpVariable.dicts("u", cities, lowBound=0, upBound=n-1, cat='Integer')
for i in cities:
for j in cities:
if i != j and (i != 0 and j != 0):
prob += u[i] - u[j] + n * x[i, j] <= n - 1
# 求解问题
prob.solve()
# 打印结果
print("最优路径:")
for i in cities:
for j in cities:
if value(x[i, j]) == 1:
print(f"从城市 {i} 到城市 {j}")
print("最短距离:", value(prob.objective))
```
这个例子使用了PuLP库来创建一个线性规划问题实例,并使用变量`x`来表示城市间的路径。目标函数是最小化路径的总距离。约束条件包括每个城市仅能连接一条路径,以及避免出现子回路。
你需要自己定义城市之间的距离矩阵`distance_matrix`,并将其替换到代码中。这个例子仅展示了如何使用线性规划库来求解TSP问题的大致思路,具体实现还需要根据你的具体问题进行调整。
相关推荐
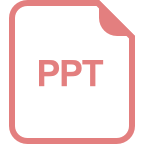
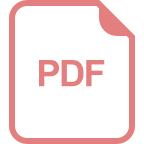
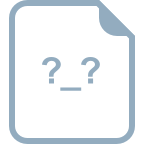














