rgb_view = spectral.imshow(data,(30,20,10),classes=label, title='RGB origin', figsize=(7, 7))
时间: 2023-10-02 20:14:05 浏览: 40
这是一段代码,用于显示带有标签的原始 RGB 图像。它使用了 `spectral.imshow()` 函数,其中 `data` 是 RGB 图像数据,`(30, 20, 10)` 是用于放大图像的增益参数,`label` 是标签数据,`title` 是图像的标题,`figsize` 是图像的大小。执行这段代码后,将会显示带有标签的 RGB 图像。
相关问题
# load the original image X, y = loadData() height = y.shape[0] width = y.shape[1] PATCH_SIZE = 25 numComponents = 4 outputs = [] for i in range(height): row_outputs = [] for j in range(width): target = int(y[i, j]) if target == 0: row_outputs.append(0) else: # extract current patch and its label patch = X[i:i+PATCH_SIZE, j:j+PATCH_SIZE] target_patch = y[i:i+PATCH_SIZE, j:j+PATCH_SIZE] # convert patch into the suitable format to feed into model patch_tensor = patch.reshape(1, PATCH_SIZE, PATCH_SIZE, numComponents, 1).astype('float32') # make prediction for the patch using the trained model prediction = model.predict(patch_tensor) prediction = np.argmax(prediction, axis=1) row_outputs.append(prediction + 1) outputs.append(row_outputs) outputs = np.array(outputs) ground_truth = spectral.imshow(classes=y, figsize=(7, 7)) predict_image = spectral.imshow(classes=outputs.astype(int), figsize=(7, 7)) spectral.save_rgb("predictions.jpg", outputs.astype(int), colors=spectral.spy_colors)
这段代码是一个基于图像分类的模型的预测过程。首先从数据集中读取原始图像和对应的标签,然后通过循环遍历每一个像素点,提取当前像素点周围的 PATCH_SIZE x PATCH_SIZE 的图像块作为输入,将图像块转化为模型可以处理的格式,使用训练好的模型进行预测,将预测结果存储在一个矩阵中。最后使用 spectral 库的函数将预测结果可视化保存为一张图片。其中,代码注释中提到的 numComponents 是指图像中的波段数,输出结果中的 0 表示该像素点为背景,1~N 表示该像素点属于某个类别。
graph = image.img_to_graph(img, mask=mask) graph.data = np.exp(-graph.data / graph.data.std()) labels = spectral_clustering(graph, n_clusters=2, eigen_solver="arpack") label_im = np.full(mask.shape, -1.0) label_im[mask] = labels这段的代码是什么意思
这段代码是图像分割的相关代码。具体解释如下:
1. `image.img_to_graph(img, mask=mask)` 将图像 `img` 转换为图形表示,其中 `mask` 是一个布尔数组,用于指定哪些像素应该包含在图形中。
2. `graph.data = np.exp(-graph.data / graph.data.std())` 对图形中的每个像素之间的边进行加权,即计算欧几里得距离并进行指数变换,这可以增强相邻像素之间的关联性,使得它们更可能被分配到同一类别。
3. `spectral_clustering(graph, n_clusters=2, eigen_solver="arpack")` 将图形表示作为输入,并使用 spectral clustering 算法将像素分成 `n_clusters` 个簇。`eigen_solver` 参数指定用于计算特征向量的求解器。
4. `label_im = np.full(mask.shape, -1.0)` 创建一个形状与 `mask` 相同的数组 `label_im`,并初始化所有元素为 `-1.0`。
5. `label_im[mask] = labels` 将 `spectral_clustering` 返回的标签 `labels` 分配给 `label_im` 中对应的像素,即将标签赋给 `mask` 中指定的像素。
最终,`label_im` 将包含像素的标签,其中 `-1.0` 表示未被分配到任何簇。
相关推荐
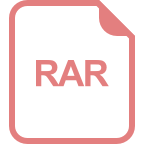
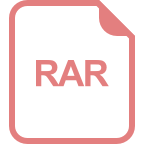
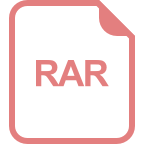












