pi/4=1-1/3+1/5有for语句编程
时间: 2024-10-26 19:03:19 浏览: 23
这个无限级数公式π/4 = 1 - 1/3 + 1/5 - 1/7 + ... 可以通过编写一个简单的 for 循环来计算。你可以创建一个循环,逐步累加正负分数。以下是使用 Python 编程的一个示例:
```python
pi_4_series = 0
n = 1
sign = 1 # 正负交替
while True:
term = sign / n
pi_4_series += term
print(f"Adding {term} to the series (current value: {pi_4_series})")
if abs(term) < 1e-6: # 设置一个极小值,当下一项小于某个阈值时停止迭代
break
n += 2
sign *= -1 # 改变正负号
print("The sum of the series is approximately:", pi_4_series)
```
在这个程序中,我们首先初始化 `pi_4_series` 为0,然后进入一个无限循环,每次循环计算当前项并将其加入总和。随着循环,我们会改变 `n` 的值,并根据需要调整 `sign` 来保证正负交替。当后续项的绝对值非常小(这里设定为1e-6)时,我们认为已经足够接近 π/4 的实际值,就跳出循环。
相关问题
###function approximation f(x)=sin(x) ###2018.08.14 ###激活函数用的是sigmoid import numpy as np import math import matplotlib.pyplot as plt x = np.linspace(-3, 3, 600) # print(x) # print(x[1]) x_size = x.size y = np.zeros((x_size, 1)) # print(y.size) for i in range(x_size): y[i] = math.sin(2*math.pi*0.4*x[i])+ math.sin(2*math.pi*0.1*x[i]) + math.sin(2*math.pi*0.9*x[i]) # print(y) hidesize = 10 W1 = np.random.random((hidesize, 1)) # 输入层与隐层之间的权重 B1 = np.random.random((hidesize, 1)) # 隐含层神经元的阈值 W2 = np.random.random((1, hidesize)) # 隐含层与输出层之间的权重 B2 = np.random.random((1, 1)) # 输出层神经元的阈值 threshold = 0.005 max_steps = 1001 def sigmoid(x_): y_ = 1 / (1 + math.exp(-x_)) return y_ E = np.zeros((max_steps, 1)) # 误差随迭代次数的变化 Y = np.zeros((x_size, 1)) # 模型的输出结果 for k in range(max_steps): temp = 0 for i in range(x_size): hide_in = np.dot(x[i], W1) - B1 # 隐含层输入数据 # print(x[i]) hide_out = np.zeros((hidesize, 1)) # 隐含层的输出数据 for j in range(hidesize): # print("第{}个的值是{}".format(j,hide_in[j])) # print(j,sigmoid(j)) hide_out[j] = sigmoid(hide_in[j]) # print("第{}个的值是{}".format(j, hide_out[j])) # print(hide_out[3]) y_out = np.dot(W2, hide_out) - B2 # 模型输出 # print(y_out) Y[i] = y_out # print(i,Y[i]) e = y_out - y[i] # 模型输出减去实际结果。得出误差 ##反馈,修改参数 dB2 = -1 * threshold * e dW2 = e * threshold * np.transpose(hide_out) dB1 = np.zeros((hidesize, 1)) for j in range(hidesize): dB1[j] = np.dot(np.dot(W2[0][j], sigmoid(hide_in[j])), (1 - sigmoid(hide_in[j])) * (-1) * e * threshold) dW1 = np.zeros((hidesize, 1)) for j in range(hidesize): dW1[j] = np.dot(np.dot(W2[0][j], sigmoid(hide_in[j])), (1 - sigmoid(hide_in[j])) * x[i] * e * threshold) W1 = W1 - dW1 B1 = B1 - dB1 W2 = W2 - dW2 B2 = B2 - dB2 temp = temp + abs(e) E[k] = temp if k % 100 == 0: print(k) plt.figure() plt.plot(x, Y) plt.plot(x, Y, color='red', linestyle='--') plt.show()这个程序如何每迭代100次就输出一次图片
要每迭代100次输出一次图片,你需要在代码中添加相应的逻辑。以下是修改后的代码示例:
```python
import numpy as np
import math
import matplotlib.pyplot as plt
x = np.linspace(-3, 3, 600)
x_size = x.size
y = np.zeros((x_size, 1))
for i in range(x_size):
y[i] = math.sin(2*math.pi*0.4*x[i]) + math.sin(2*math.pi*0.1*x[i]) + math.sin(2*math.pi*0.9*x[i])
hidesize = 10
W1 = np.random.random((hidesize, 1))
B1 = np.random.random((hidesize, 1))
W2 = np.random.random((1, hidesize))
B2 = np.random.random((1, 1))
threshold = 0.005
max_steps = 1001
def sigmoid(x_):
y_ = 1 / (1 + math.exp(-x_))
return y_
E = np.zeros((max_steps, 1))
Y = np.zeros((x_size, 1))
for k in range(max_steps):
temp = 0
for i in range(x_size):
hide_in = np.dot(x[i], W1) - B1
hide_out = np.zeros((hidesize, 1))
for j in range(hidesize):
hide_out[j] = sigmoid(hide_in[j])
y_out = np.dot(W2, hide_out) - B2
Y[i] = y_out
e = y_out - y[i]
dB2 = -1 * threshold * e
dW2 = e * threshold * np.transpose(hide_out)
dB1 = np.zeros((hidesize, 1))
for j in range(hidesize):
dB1[j] = np.dot(np.dot(W2[0][j], sigmoid(hide_in[j])), (1 - sigmoid(hide_in[j])) * (-1) * e * threshold)
dW1 = np.zeros((hidesize, 1))
for j in range(hidesize):
dW1[j] = np.dot(np.dot(W2[0][j], sigmoid(hide_in[j])), (1 - sigmoid(hide_in[j])) * x[i] * e * threshold)
W1 = W1 - dW1
B1 = B1 - dB1
W2 = W2 - dW2
B2 = B2 - dB2
temp = temp + abs(e)
E[k] = temp
if k % 100 == 0:
plt.figure()
plt.plot(x, Y)
plt.plot(x, Y, color='red', linestyle='--')
plt.savefig(f'iteration_{k}.png')
plt.close()
plt.figure()
plt.plot(x, Y)
plt.plot(x, Y, color='red', linestyle='--')
plt.show()
```
在上述示例中,我添加了一个条件判断语句`if k % 100 == 0`来判断是否达到每100次迭代的条件。如果满足条件,则在该迭代结束后绘制并保存图片,文件名为`iteration_k.png`,其中`k`表示当前迭代次数。
请根据你的具体需求和编程环境进行相应的调整。
第1关:计算圆周率——蒙特卡洛法编程实现用蒙特卡洛方法计算π值,为了自动测评的需要,请先读入一个正整数sd作为随机数种子,并要求使用 x,y = random.uniform(-1,1) , random.uniform(-1,1) 语句来生成随机点的坐标值。
好的,这是一个计算圆周率的问题,可以使用蒙特卡洛方法来实现。以下是用Python实现的代码:
```python
import random
# 读入随机数种子
sd = int(input())
# 初始化计数器
count = 0
# 循环生成随机点,并判断是否在圆内
for i in range(100000):
x, y = random.uniform(-1, 1), random.uniform(-1, 1)
if x ** 2 + y ** 2 <= 1:
count += 1
# 计算圆的面积和正方形的面积,估算圆周率
pi = 4 * count / 100000
print(pi)
```
代码的思路是,在一个边长为2的正方形内,随机生成大量的点,然后统计落在一个以(0,0)为圆心,半径为1的圆内的点的数量。根据概率统计的知识,可以得出圆的面积和正方形的面积的比值,而圆的面积和正方形的面积的比值等于圆的面积占正方形面积的比例,而圆的面积等于πr²,而正方形面积等于(2r)²=4r²,因此可以用这个比例来估算圆周率π的值。
阅读全文
相关推荐
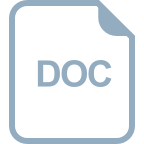
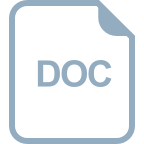
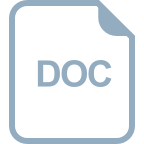
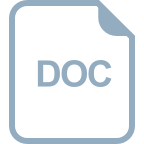
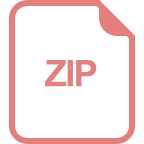
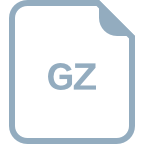
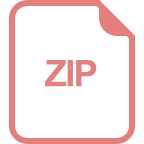
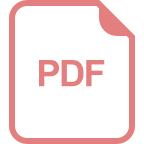
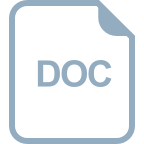
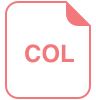







