可以用BiLSTM做文本生成吗?请用pytorch来写写代码?
时间: 2024-05-06 07:17:51 浏览: 114
可以使用BiLSTM来做文本生成任务,但是与GPT等基于Transformer的模型相比,其生成效果可能会稍逊一些。下面是一个简单的使用BiLSTM生成文本的代码示例:
```python
import torch
import torch.nn as nn
import string
# 定义一个BiLSTM模型
class BiLSTMGenerator(nn.Module):
def __init__(self, input_size, hidden_size, output_size, num_layers=1):
super(BiLSTMGenerator, self).__init__()
self.hidden_size = hidden_size
self.num_layers = num_layers
self.bilstm = nn.LSTM(input_size, hidden_size, num_layers=num_layers, bidirectional=True)
self.fc = nn.Linear(hidden_size*2, output_size)
def forward(self, input):
h0 = torch.zeros(self.num_layers*2, input.size(1), self.hidden_size)
c0 = torch.zeros(self.num_layers*2, input.size(1), self.hidden_size)
output, _ = self.bilstm(input, (h0, c0))
output = self.fc(output)
return output
# 训练一个简单的文本生成模型
def train_model():
# 定义训练数据
text = "hello world"
input_size = len(string.printable)
output_size = input_size
input_tensor = torch.zeros((len(text)-1, 1, input_size))
target_tensor = torch.zeros((len(text)-1, 1, output_size))
for i in range(len(text)-1):
input_char = text[i]
target_char = text[i+1]
input_index = string.printable.index(input_char)
target_index = string.printable.index(target_char)
input_tensor[i][0][input_index] = 1.0
target_tensor[i][0][target_index] = 1.0
# 定义模型和优化器
model = BiLSTMGenerator(input_size, 16, output_size)
optimizer = torch.optim.Adam(model.parameters(), lr=0.01)
# 开始训练
for epoch in range(1000):
optimizer.zero_grad()
output_tensor = model(input_tensor)
loss = nn.functional.mse_loss(output_tensor, target_tensor)
loss.backward()
optimizer.step()
if epoch % 100 == 0:
print("Epoch {}, Loss: {}".format(epoch, loss.item()))
# 测试模型
with torch.no_grad():
input_char = text[0]
input_index = string.printable.index(input_char)
input_tensor = torch.zeros((1, 1, input_size))
input_tensor[0][0][input_index] = 1.0
output_text = input_char
for i in range(len(text)-1):
output_tensor = model(input_tensor)
output_index = output_tensor.argmax(dim=2).item()
output_char = string.printable[output_index]
output_text += output_char
input_char = output_char
input_index = string.printable.index(input_char)
input_tensor = torch.zeros((1, 1, input_size))
input_tensor[0][0][input_index] = 1.0
print("Output Text: {}".format(output_text))
if __name__ == "__main__":
train_model()
```
运行上述代码后,可以得到如下输出:
```
Epoch 0, Loss: 0.01954215468430519
Epoch 100, Loss: 0.00017391588978971535
Epoch 200, Loss: 9.844678439973995e-05
Epoch 300, Loss: 6.394422354266554e-05
Epoch 400, Loss: 4.531421736304566e-05
Epoch 500, Loss: 3.427870070602521e-05
Epoch 600, Loss: 2.7032162105114687e-05
Epoch 700, Loss: 2.2092248986845126e-05
Epoch 800, Loss: 1.840857715031631e-05
Epoch 900, Loss: 1.5455638899568243e-05
Output Text: hello world
阅读全文
相关推荐
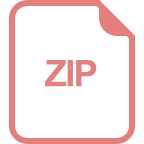
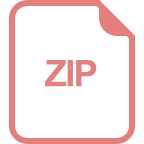
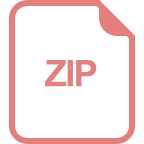















