train_X, test_X, train_y, test_y = train_test_split(content_list, label_list)
时间: 2024-05-06 17:14:19 浏览: 10
根据你提供的引用,train_test_split()函数将传入的数据集随机划分为训练集和测试集。其中,train_data表示自变量(输入),train_target表示因变量(输出),test_size表示测试集占总数据集的比例,random_state则是随机数种子,保证每次随机结果相同。函数会返回四个list,分别为训练集的自变量train_X、测试集的自变量test_X、训练集的因变量train_y和测试集的因变量test_y。
所以对于你的问题,假设content_list和label_list分别为输入和输出数据,可以使用以下代码进行划分:
```python
from sklearn.model_selection import train_test_split
train_X, test_X, train_y, test_y = train_test_split(content_list, label_list, test_size=0.4, random_state=0)
```
这里test_size=0.4表示测试集占40%,random_state=0保证每次随机结果相同。
相关问题
df_1_final_test = df_1.loc[list(set(df_1.index.tolist()).difference(set(df_train_1.index.tolist())))] #df_9_final_test = df_9.copy() 使负样本验证集等于正样本的验证集 df_9_final_test = df_9.sample(round(len(df_1_final_test)), random_state=int(cfg_train_dict['random_state'])) df_9_final_test['label'] = 0 df_ft = df_1_final_test.append(df_9_final_test, sort=False) # 随机分训练集和测试集 from sklearn.model_selection import train_test_split x_train, x_test, y_train, y_test = train_test_split(df_train.drop(['号码', 'label'], axis=1), df_train['label'], test_size=0.2, random_state=int(cfg_train_dict['random_state']))
这段代码是将数据集划分为训练集和测试集的代码。
首先,代码根据 df_1 和 df_train_1 的索引的差异,获取了 df_1 中不在训练集中的样本,并将其赋值给 df_1_final_test。
接着,代码从 df_9 数据框中随机抽样数量为 round(len(df_1_final_test)) 的样本作为负例测试集,并给其添加一个名为 'label' 的列,所有行的值都设置为 0。
然后,代码将 df_1_final_test 和 df_9_final_test 两个数据框按行合并成一个新的数据框 df_ft。
接下来,代码使用 train_test_split 函数将 df_train 数据框划分为训练集和测试集。其中,参数 df_train.drop(['号码', 'label'], axis=1) 表示训练集的特征数据,df_train['label'] 表示训练集的标签数据。test_size 参数设置了测试集的比例,这里是 0.2,即 20% 的样本被划分为测试集。random_state 参数用于设置随机种子。
最后,代码将划分好的训练集和测试集分别赋值给 x_train、x_test、y_train、y_test 变量。
这段代码的作用是将数据集划分为训练集和测试集,用于模型的训练和评估。其中,df_train 包含了正例样本和负例样本,df_ft 包含了未在训练集中出现的正例样本和负例样本。x_train、x_test、y_train、y_test 则是划分好的训练集和测试集的特征数据和标签数据。
# 封装函数来进行knn试探性运算 def knn_score(k,X,y): # 构造算法对象 knn = KNeighborsClassifier(n_neighbors = k) scores = [] train_scores = [] for i in range(100): # 拆分 X_train,X_test,y_train,y_test = train_test_split(X,y,test_size=0.2,random_state=1) # 训练 knn.fit(X_train,y_train) # 评价模型 scores.append(knn.score(X_test,y_test)) # 经验评分 train_scores.append(knn.score(X_train,y_train)) return np.array(scores).mean(),np.array(train_scores).mean() # 调参 result_dict = {} k_list = [1,3,5,7,9,11] for k in k_list: score,train_score = knn_score(k,X,y) result_dict[k] = [score,train_score]利用这段代码调用tkinter库实现界面
可以使用`tkinter`库来创建一个窗口界面,然后在界面上添加按钮,当按钮被点击时,调用`knn_score()`函数进行试探性运算并将结果显示在界面上。
以下是一个简单的示例代码:
```python
import tkinter as tk
from sklearn.neighbors import KNeighborsClassifier
from sklearn.model_selection import train_test_split
import numpy as np
def knn_score(k,X,y):
# 构造算法对象
knn = KNeighborsClassifier(n_neighbors = k)
scores = []
train_scores = []
for i in range(100):
# 拆分
X_train,X_test,y_train,y_test = train_test_split(X,y,test_size=0.2,random_state=1)
# 训练
knn.fit(X_train,y_train)
# 评价模型
scores.append(knn.score(X_test,y_test))
# 经验评分
train_scores.append(knn.score(X_train,y_train))
return np.array(scores).mean(),np.array(train_scores).mean()
# 创建窗口
root = tk.Tk()
root.title("KNN试探性运算")
# 添加标签
label1 = tk.Label(root, text="KNN试探性运算", font=("Arial", 16))
label1.pack()
# 添加按钮
button1 = tk.Button(root, text="运算", font=("Arial", 12))
button1.pack()
# 添加文本框
text1 = tk.Text(root, width=50, height=10)
text1.pack()
# 绑定按钮事件
def button_click():
X = # 定义特征矩阵
y = # 定义目标变量
result_dict = {}
k_list = [1,3,5,7,9,11]
for k in k_list:
score,train_score = knn_score(k,X,y)
result_dict[k] = [score,train_score]
# 将结果显示在文本框中
text1.delete(1.0, tk.END)
text1.insert(tk.END, str(result_dict))
button1.config(command=button_click)
# 运行窗口
root.mainloop()
```
注意:此示例代码中的`X`和`y`需要根据实际情况进行定义。
相关推荐
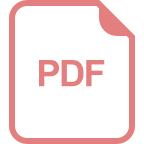
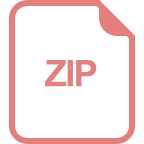












