解释new_events = mne.make_fixed_length_events(raw, start=20, stop=70, duration=10.)
时间: 2023-06-12 22:06:50 浏览: 227
这行代码使用MNE-Python库中的`make_fixed_length_events`函数从连续的原始数据中提取事件。其中,`raw`是原始数据,`start`和`stop`是开始和结束的时间(单位为秒),`duration`是每个事件的持续时间(单位为秒)。该函数将原始数据分成多个固定长度的时间段,并将每个时间段视为一个事件。返回的`new_events`是一个包含每个事件的时间戳和事件ID的NumPy数组。
相关问题
import scipy.io import mne from mne.bem import make_watershed_bem # Load .mat files inner_skull = scipy.io.loadmat('E:\MATLABproject\data\MRI\Visit1_040318\\tess_mri_COR_MPRAGE_RECON-mocoMEMPRAGE_FOV_220-298665.inner_skull.mat') outer_skull = scipy.io.loadmat('E:\MATLABproject\data\MRI\Visit1_040318\\tess_mri_COR_MPRAGE_RECON-mocoMEMPRAGE_FOV_220-298665.outer_skull.mat') scalp = scipy.io.loadmat('E:\MATLABproject\data\MRI\Visit1_040318\\tess_mri_COR_MPRAGE_RECON-mocoMEMPRAGE_FOV_220-298665.scalp.mat') print(inner_skull.keys()) # Assuming these .mat files contain triangulated surfaces, we will extract vertices and triangles # This might need adjustment based on the actual structure of your .mat files inner_skull_vertices = inner_skull['Vertices'] inner_skull_triangles = inner_skull['Faces'] outer_skull_vertices = outer_skull['Vertices'] outer_skull_triangles = outer_skull['Faces'] scalp_vertices = scalp['Vertices'] scalp_triangles = scalp['Faces'] # Prepare surfaces for MNE surfs = [ mne.bem.BEMSurface(inner_skull_vertices, inner_skull_triangles, sigma=0.01, id=4), # brain mne.bem.BEMSurface(outer_skull_vertices, outer_skull_triangles, sigma=0.016, id=3), # skull mne.bem.BEMSurface(scalp_vertices, scalp_triangles, sigma=0.33, id=5), # skin ] # Create BEM model model = mne.bem.BEM(surfs, conductivity=[0.3, 0.006, 0.3], is_sphere=False) model.plot(show=False) # Create BEM solution solution = mne.make_bem_solution(model) 运行代码时报错; Traceback (most recent call last): File "E:\pythonProject\MEG\头模型.py", line 24, in <module> mne.bem.BEMSurface(inner_skull_vertices, inner_skull_triangles, sigma=0.01, id=4), # brain AttributeError: module 'mne.bem' has no attribute 'BEMSurface'
根据你的代码和报错信息来看,mne 模块确实没有 `BEMSurface` 这个属性。根据官方文档,在 mne 的最新版本中,使用 `BEMSurface` 已经被废弃,而改用了 `make_watershed_bem` 函数来创建 BEM 模型。
你可以尝试修改你的代码如下:
```python
import scipy.io
import mne
from mne.bem import make_watershed_bem
# Load .mat files
inner_skull = scipy.io.loadmat('E:\MATLABproject\data\MRI\Visit1_040318\\tess_mri_COR_MPRAGE_RECON-mocoMEMPRAGE_FOV_220-298665.inner_skull.mat')
outer_skull = scipy.io.loadmat('E:\MATLABproject\data\MRI\Visit1_040318\\tess_mri_COR_MPRAGE_RECON-mocoMEMPRAGE_FOV_220-298665.outer_skull.mat')
scalp = scipy.io.loadmat('E:\MATLABproject\data\MRI\Visit1_040318\\tess_mri_COR_MPRAGE_RECON-mocoMEMPRAGE_FOV_220-298665.scalp.mat')
print(inner_skull.keys())
# Assuming these .mat files contain triangulated surfaces, we will extract vertices and triangles
# This might need adjustment based on the actual structure of your .mat files
inner_skull_vertices = inner_skull['Vertices']
inner_skull_triangles = inner_skull['Faces']
outer_skull_vertices = outer_skull['Vertices']
outer_skull_triangles = outer_skull['Faces']
scalp_vertices = scalp['Vertices']
scalp_triangles = scalp['Faces']
# Prepare surfaces for MNE
surfs = [
mne.make_bem_model(inner_skull_vertices, inner_skull_triangles, conductivity=0.01), # brain
mne.make_bem_model(outer_skull_vertices, outer_skull_triangles, conductivity=0.016), # skull
mne.make_bem_model(scalp_vertices, scalp_triangles, conductivity=0.33), # skin
]
# Create BEM solution
model = make_watershed_bem(surfs)
solution = mne.make_bem_solution(model)
```
请注意,我还对 `make_bem_model` 的调用进行了修改,以适应新的 API。希望这能解决你的问题!如果还有其他疑问,请随时提问。
如果使用data_path = mne.datasets.ssvep.data_path()这个数据集来进行以上处理呢,请生成代码
以下是使用MNE库加载SSVEP数据集并进行预处理的示例代码:
```python
import mne
# Load SSVEP dataset
data_path = mne.datasets.ssvep.data_path()
raw = mne.io.read_raw_edf(data_path + '/sub-01/ses-test/eeg/sub-01_ses-test_task-ssvep_eeg.edf', preload=True)
# Set channel types and montage
raw.set_channel_types({'HEOG': 'eog', 'VEOG': 'eog'})
montage = mne.channels.make_standard_montage('standard_1005')
raw.set_montage(montage)
# Apply bandpass filter
raw.filter(1, 40)
# Apply notch filter to remove line noise at 50 Hz
raw.notch_filter(50)
# Apply ICA to remove eye blinks and other artifacts
ica = mne.preprocessing.ICA(n_components=20, random_state=0)
ica.fit(raw)
raw = ica.apply(raw)
# Epoch data and apply baseline correction
events, event_id = mne.events_from_annotations(raw)
epochs = mne.Epochs(raw, events, event_id, tmin=0, tmax=4, baseline=(None, 0), preload=True)
# Average across trials
evoked = epochs.average()
# Plot evoked response
evoked.plot()
```
阅读全文
相关推荐
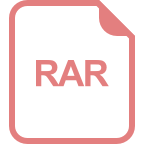
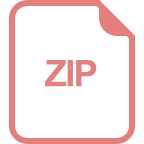













