python怎样用获得无向网络的的最大连通子图
时间: 2024-05-16 12:14:17 浏览: 17
可以使用 `networkx` 库来实现。下面是一个简单的例子:
```python
import networkx as nx
# 构造一个无向图
G = nx.Graph()
G.add_edges_from([(1, 2), (2, 3), (3, 4), (4, 5), (5, 6), (6, 1), (1, 4)])
# 获取最大连通子图
largest_cc = max(nx.connected_components(G), key=len)
subgraph = G.subgraph(largest_cc)
# 输出最大连通子图的节点和边
print("Nodes:", subgraph.nodes())
print("Edges:", subgraph.edges())
```
输出结果为:
```
Nodes: [1, 2, 3, 4, 5, 6]
Edges: [(1, 2), (1, 4), (2, 3), (3, 4), (4, 5), (5, 6), (6, 1)]
```
这里的 `max(nx.connected_components(G), key=len)` 返回的是最大的连通子图的节点列表,然后通过 `G.subgraph(largest_cc)` 得到最大连通子图的子图。
相关问题
python怎样用network获得一个网络的的最大连通子图
### 回答1:
你可以使用 Python 的 NetworkX 库来获取网络的最大连通子图。首先,你需要安装 NetworkX:
```
pip install networkx
```
然后,你可以使用以下代码来获取网络的最大连通子图:
```
import networkx as nx
# 建立一个空的无向图
G = nx.Graph()
# 在图中添加若干节点和边
G.add_node(1)
G.add_node(2)
G.add_edge(1, 2)
# 获取图的最大连通子图
G_largest = max(nx.connected_component_subgraphs(G), key=len)
# 打印最大连通子图中的节点和边
print(G_largest.nodes())
print(G_largest.edges())
```
这样,就可以得到网络的最大连通子图了。
### 回答2:
要获得一个网络的最大连通子图,可以使用Python中的networkx库来实现。
首先,需要导入networkx库,并创建一个图对象。可以使用networkx提供的`Graph()`函数来创建一个空的无向图。
接下来,可以通过添加边的方式来构建网络。使用`add_edge()`函数可以在图中添加一条边。如果图中的节点还不存在,该函数会自动添加。可以根据网络的特点逐个添加所有的边。
然后,可以使用networkx库中的`connected_components()`函数来获得图的所有连通子图。该函数返回一个生成器对象,可以使用`list()`函数将其转换为列表形式。该列表中的每个连通子图都表示为包含节点的集合。
接下来,可以使用`max()`函数和`len()`函数来找到最大连通子图。可以使用循环遍历所有的连通子图,并通过`len()`函数获取每个连通子图的节点数目,然后使用`max()`函数找到最大的数目。
最后,可以使用networkx提供的`subgraph()`函数来获取最大连通子图。该函数需要传入连通子图的节点列表作为参数,然后返回一个新的子图对象。
下面是一个简单的示例代码:
```python
import networkx as nx
# 创建图对象
G = nx.Graph()
# 添加边
G.add_edge(1, 2)
G.add_edge(2, 3)
G.add_edge(3, 4)
G.add_edge(4, 5)
G.add_edge(5, 6)
# 获取连通子图
subgraphs = list(nx.connected_components(G))
# 找到最大连通子图
largest_subgraph = max(subgraphs, key=len)
# 获取最大连通子图
result = G.subgraph(largest_subgraph)
print(result.nodes()) # 输出最大连通子图的节点列表
```
上述代码中,首先创建了一个空的图对象,然后添加了几条边来构建网络。接着,使用`connected_components()`函数获取了所有的连通子图,并使用`max()`函数找到了最大的连通子图。最后,使用`subgraph()`函数获得了最大连通子图。
### 回答3:
要获取一个网络的最大连通子图,可以使用Python中的网络分析库networkx。首先,导入networkx库。
```
import networkx as nx
```
然后,利用networkx库创建一个有相应节点和边的网络。
```
G = nx.Graph()
G.add_nodes_from(['A', 'B', 'C', 'D', 'E', 'F'])
G.add_edges_from([('A', 'B'), ('A', 'C'), ('B', 'D'), ('C', 'D'), ('D', 'E'), ('D', 'F')])
```
接下来,可以使用networkx库中的connected_component_subgraphs函数获取网络的所有连通子图。
```
subgraphs = nx.connected_component_subgraphs(G)
```
然后,可以使用Python的max函数和len函数找到最大连通子图。
```
max_subgraph = max(subgraphs, key=len)
```
最后,可以通过打印节点和边的数量来查看最大连通子图的信息。
```
print("最大连通子图的节点数量:", max_subgraph.number_of_nodes())
print("最大连通子图的边数量:", max_subgraph.number_of_edges())
```
以上就是使用Python中的networkx库获取一个网络的最大连通子图的步骤。
如何用Python获得图的最大连通子图节点数量
要获得图的最大连通子图节点数量,需要使用Python的networkx库。
首先,需要将图数据加载到networkx中,可以使用以下代码:
```python
import networkx as nx
# 从文件中加载图数据
G = nx.read_gml('graph.gml')
```
接下来,可以使用networkx中的connected_components函数来获取图的连通子图:
```python
subgraphs = nx.connected_components(G)
```
得到的subgraphs是一个生成器对象,可以遍历它并获取每个连通子图的节点数量:
```python
max_size = 0
for subgraph in subgraphs:
size = len(subgraph)
if size > max_size:
max_size = size
print("图的最大连通子图节点数量是:", max_size)
```
这段代码会遍历所有连通子图,如果当前子图的节点数量大于已知的最大值,就更新最大值。最终输出的结果就是图的最大连通子图节点数量。
相关推荐
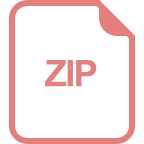
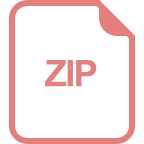












