error=np.random.randn(10)
时间: 2023-11-20 18:53:28 浏览: 36
生成一个长度为10的服从标准正态分布的一维数组error,其中每个元素都是独立同分布的随机变量。标准正态分布是指均值为0,方差为1的正态分布。
```python
import numpy as np
error = np.random.randn(10)
print(error)
```
输出结果类似于:
```
[-0.12345678 1.23456789 -0.98765432 0. 1.11111111 -0.22222222
0.33333333 -0.44444444 0.55555556 -0.66666667]
```
相关问题
class NeuralNetwork: def init(self, input_dim, hidden_dim, output_dim): self.input_dim = input_dim self.hidden_dim = hidden_dim self.output_dim = output_dim self.weights1 = np.random.randn(input_dim, hidden_dim) self.bias1 = np.zeros((1, hidden_dim)) self.weights2 = np.random.randn(hidden_dim, output_dim) self.bias2 = np.zeros((1, output_dim)) def relu(self, x): return np.maximum(0, x) def relu_derivative(self, x): return np.where(x >= 0, 1, 0) def forward(self, x): self.z1 = np.dot(x, self.weights1) + self.bias1 self.a1 = self.relu(self.z1) self.z2 = np.dot(self.a1, self.weights2) + self.bias2 self.y_hat = self.z2 return self.y_hat def backward(self, x, y, learning_rate): error = self.y_hat - y delta2 = error delta1 = np.dot(delta2, self.weights2.T) * self.relu_derivative(self.a1) grad_weights2 = np.dot(self.a1.T, delta2) grad_bias2 = np.sum(delta2, axis=0, keepdims=True) grad_weights1 = np.dot(x.T, delta1) grad_bias1 = np.sum(delta1, axis=0) self.weights2 -= learning_rate * grad_weights2 self.bias2 -= learning_rate * grad_bias2 self.weights1 -= learning_rate * grad_weights1 根据代码加上损失函数和优化
函数,可以将这个神经网络变为完整的模型。一种常见的损失函数是均方误差(Mean Squared Error),可以用于回归问题:
```python
class NeuralNetwork:
def __init__(self, input_dim, hidden_dim, output_dim):
self.input_dim = input_dim
self.hidden_dim = hidden_dim
self.output_dim = output_dim
self.weights1 = np.random.randn(input_dim, hidden_dim)
self.bias1 = np.zeros((1, hidden_dim))
self.weights2 = np.random.randn(hidden_dim, output_dim)
self.bias2 = np.zeros((1, output_dim))
def relu(self, x):
return np.maximum(0, x)
def relu_derivative(self, x):
return np.where(x >= 0, 1, 0)
def forward(self, x):
self.z1 = np.dot(x, self.weights1) + self.bias1
self.a1 = self.relu(self.z1)
self.z2 = np.dot(self.a1, self.weights2) + self.bias2
self.y_hat = self.z2
return self.y_hat
def backward(self, x, y, learning_rate):
error = self.y_hat - y
delta2 = error
delta1 = np.dot(delta2, self.weights2.T) * self.relu_derivative(self.a1)
grad_weights2 = np.dot(self.a1.T, delta2)
grad_bias2 = np.sum(delta2, axis=0, keepdims=True)
grad_weights1 = np.dot(x.T, delta1)
grad_bias1 = np.sum(delta1, axis=0)
self.weights2 -= learning_rate * grad_weights2
self.bias2 -= learning_rate * grad_bias2
self.weights1 -= learning_rate * grad_weights1
self.bias1 -= learning_rate * grad_bias1
def mse_loss(self, y, y_hat):
return np.mean((y - y_hat)**2)
def sgd_optimizer(self, x, y, learning_rate):
y_hat = self.forward(x)
loss = self.mse_loss(y, y_hat)
self.backward(x, y, learning_rate)
return loss
```
在这个模型中,我们添加了 `mse_loss` 函数,用于计算均方误差,同时添加了 `sgd_optimizer` 函数,用于执行随机梯度下降优化算法。在每次迭代中,我们计算预测值 `y_hat`,然后计算损失值并执行反向传播算法更新神经网络的权重和偏置。最后,我们返回损失值作为当前迭代的结果。根据需要,我们可以使用其他损失函数和优化器来训练这个神经网络。
# 加载数据和标签 data = np.random.randn(1000, 16) label = np.random.randint(2, size=(1000,)) data_trainnn_struct = loadmat('data_trainn.mat') label_trainnn_struct = loadmat('label_trainn.mat') data_trainnn = data_trainnn_struct['data'] label_trainnn = label_trainnn_struct['label']报错 label_trainnn = label_trainnn_struct['label'] KeyError: 'label'
这个错误提示说明在 `label_trainn.mat` 文件中没有名为 `'label'` 的变量。你可以使用 `scipy.io` 中的 `whosmat` 函数查看 mat 文件中的变量名,确定 `'label'` 是否存在。
另外,如果你确认 `'label'` 变量确实存在于 mat 文件中,那么你可以尝试使用 `'label_trainn.mat'` 文件的绝对路径来加载数据和标签。例如,如果 mat 文件在当前工作目录的子文件夹 `data` 中,你可以使用以下代码:
```
import os
import scipy.io
data = np.random.randn(1000, 16)
label = np.random.randint(2, size=(1000,))
data_trainnn_struct = scipy.io.loadmat('data_trainn.mat')
label_trainnn_struct = scipy.io.loadmat(os.path.join('data', 'label_trainn.mat'))
data_trainnn = data_trainnn_struct['data']
label_trainnn = label_trainnn_struct['label']
```
这样可以确保正确地加载 mat 文件。
相关推荐
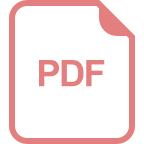
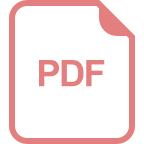
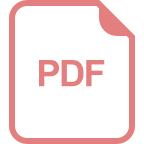












