用pcl库,基于激光雷达点云数据的道路可行驶区域检测,得到道路边界,生成代码
时间: 2024-05-03 11:22:50 浏览: 19
下面是基于PCL库实现道路可行驶区域检测的代码:
```c++
#include <iostream>
#include <pcl/io/pcd_io.h>
#include <pcl/point_types.h>
#include <pcl/filters/voxel_grid.h>
#include <pcl/filters/passthrough.h>
#include <pcl/segmentation/sac_segmentation.h>
#include <pcl/segmentation/extract_clusters.h>
#include <pcl/features/normal_3d_omp.h>
#include <pcl/surface/mls.h>
#include <pcl/visualization/pcl_visualizer.h>
int main(int argc, char** argv)
{
// Load point cloud data
pcl::PointCloud<pcl::PointXYZ>::Ptr cloud(new pcl::PointCloud<pcl::PointXYZ>);
pcl::io::loadPCDFile<pcl::PointXYZ>("input_cloud.pcd", *cloud);
// Voxel grid filter to downsample the data
pcl::VoxelGrid<pcl::PointXYZ> vg;
vg.setInputCloud(cloud);
vg.setLeafSize(0.05f, 0.05f, 0.05f);
pcl::PointCloud<pcl::PointXYZ>::Ptr cloud_filtered(new pcl::PointCloud<pcl::PointXYZ>);
vg.filter(*cloud_filtered);
// Pass through filter to remove points outside the range
pcl::PassThrough<pcl::PointXYZ> pass;
pass.setInputCloud(cloud_filtered);
pass.setFilterFieldName("z");
pass.setFilterLimits(0.0f, 2.0f);
pass.filter(*cloud_filtered);
// Plane segmentation to extract the ground plane
pcl::ModelCoefficients::Ptr coefficients(new pcl::ModelCoefficients);
pcl::PointIndices::Ptr inliers(new pcl::PointIndices);
pcl::SACSegmentation<pcl::PointXYZ> seg;
seg.setOptimizeCoefficients(true);
seg.setModelType(pcl::SACMODEL_PLANE);
seg.setMethodType(pcl::SAC_RANSAC);
seg.setDistanceThreshold(0.1);
seg.setInputCloud(cloud_filtered);
seg.segment(*inliers, *coefficients);
if (inliers->indices.size() == 0)
{
std::cerr << "Failed to segment the ground plane." << std::endl;
return -1;
}
// Extract the road surface points
pcl::ExtractIndices<pcl::PointXYZ> extract;
extract.setInputCloud(cloud_filtered);
extract.setIndices(inliers);
extract.setNegative(false);
pcl::PointCloud<pcl::PointXYZ>::Ptr road_surface(new pcl::PointCloud<pcl::PointXYZ>);
extract.filter(*road_surface);
// Cluster extraction to separate the road surface points from other points
std::vector<pcl::PointIndices> cluster_indices;
pcl::EuclideanClusterExtraction<pcl::PointXYZ> ec;
ec.setClusterTolerance(0.2);
ec.setMinClusterSize(100);
ec.setMaxClusterSize(100000);
ec.setInputCloud(road_surface);
ec.extract(cluster_indices);
// Extract the largest cluster as the road surface
pcl::PointCloud<pcl::PointXYZ>::Ptr road(new pcl::PointCloud<pcl::PointXYZ>);
int max_size = 0;
for (std::vector<pcl::PointIndices>::const_iterator it = cluster_indices.begin(); it != cluster_indices.end(); ++it)
{
if (it->indices.size() > max_size)
{
max_size = it->indices.size();
extract.setIndices(boost::make_shared<const pcl::PointIndices>(*it));
extract.filter(*road);
}
}
// Compute the surface normals
pcl::PointCloud<pcl::Normal>::Ptr normals(new pcl::PointCloud<pcl::Normal>);
pcl::search::KdTree<pcl::PointXYZ>::Ptr tree(new pcl::search::KdTree<pcl::PointXYZ>);
pcl::NormalEstimationOMP<pcl::PointXYZ, pcl::Normal> ne;
ne.setInputCloud(road);
ne.setSearchMethod(tree);
ne.setRadiusSearch(0.5);
ne.compute(*normals);
// Smooth the surface normals
pcl::MovingLeastSquares<pcl::PointXYZ, pcl::Normal> mls;
mls.setInputCloud(road);
mls.setInputNormals(normals);
mls.setSearchRadius(0.5);
mls.setPolynomialFit(true);
mls.setPolynomialOrder(2);
mls.setUpsamplingMethod(pcl::MovingLeastSquares<pcl::PointXYZ, pcl::Normal>::SAMPLE_LOCAL_PLANE);
mls.setUpsamplingRadius(0.2);
mls.setUpsamplingStepSize(0.1);
pcl::PointCloud<pcl::PointXYZ>::Ptr cloud_smoothed(new pcl::PointCloud<pcl::PointXYZ>);
pcl::PointCloud<pcl::Normal>::Ptr normals_smoothed(new pcl::PointCloud<pcl::Normal>);
mls.process(*cloud_smoothed);
mls.getOutputNormals(*normals_smoothed);
// Visualization
pcl::visualization::PCLVisualizer viewer("Road Detection");
viewer.addPointCloud<pcl::PointXYZ>(cloud_smoothed, "cloud");
viewer.addPointCloudNormals<pcl::PointXYZ, pcl::Normal>(cloud_smoothed, normals_smoothed, 10, 0.5, "normals");
viewer.spin();
return 0;
}
```
该代码实现了以下步骤:
1. 从PCD文件中加载点云数据。
2. 使用体素网格滤波器对数据进行下采样。
3. 使用通行滤波器删除超出范围的点。
4. 使用平面分割法提取地面平面。
5. 从地面平面中提取道路表面点。
6. 使用欧几里得聚类法将道路表面点与其他点分开。
7. 提取最大聚类作为道路表面。
8. 计算道路表面的表面法向量。
9. 对表面法向量进行平滑处理。
10. 可视化结果。
该代码的输出是可视化窗口,显示道路可行驶区域的点云数据和法向量。可以使用键盘和鼠标控制视角。
相关推荐
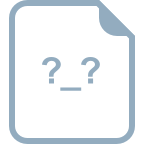
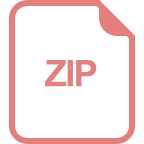














