${sizerequired} Evaluate 2048*1024 runPass fs_size=$(df | grep -w ${USERDATAMOUNTPOINT} | awk '{print $4}') ${output} run if [ \${fs_size} -le ${sizerequired} ];then echo "${check_magic}";fi ${count} get count ${output} ${check_magic} 这段话是什么意思
时间: 2023-11-01 19:52:36 浏览: 34
这段话是一个脚本的片段,用于检查磁盘空间是否满足要求,并统计 `${check_magic}` 在输出中的出现次数。
具体解释如下:
1. `${sizerequired}` 是一个变量,用于指定所需的磁盘空间大小。
2. `Evaluate 2048*1024` 是一个命令,用于计算表达式 `2048*1024` 的结果,即 2097152。
3. `runPass` 是一个命令,用于执行一个子任务。在这个子任务中,`fs_size=$(df | grep -w ${USERDATAMOUNTPOINT} | awk '{print $4}')` 是一个命令,通过调用 `df` 命令获取磁盘空间信息,并使用 `grep` 和 `awk` 过滤和提取出可用磁盘空间大小,并将其存储在变量 `fs_size` 中。
4. `${output}` 是一个占位符,表示输出结果的位置。
5. `run if [ \${fs_size} -le ${sizerequired} ];then echo "${check_magic}";fi` 是一个条件语句,如果可用磁盘空间 `${fs_size}` 小于或等于所需大小 `${sizerequired}`,则输出 `${check_magic}`。
6. `${count} get count ${output} ${check_magic}` 是一个命令,用于统计输出中 `${check_magic}` 的出现次数。
总结起来,这段脚本的作用是计算磁盘空间是否满足要求,并统计输出中 `${check_magic}` 的出现次数。
相关问题
car_type == 2 || cValue.length < 6 || (type === 1 && index < 6) || type === 3什么意思
&& cValue.indexOf(' ') !== -1)
This code is incomplete and does not make logical sense as it is missing an ending parenthesis and an ending bracket, causing a syntax error. However, assuming the missing code is added, this is a conditional statement that checks three conditions using logical operators:
1. car_type == 2 - checks if the value of car_type is equal to 2
2. cValue.length < 6 - checks if the length of cValue is less than 6
3. (type === 1 && cValue.indexOf(' ') !== -1) - checks if the value of type is equal to 1 AND if there is a space character in cValue
The || (OR) operator is used to specify that any one of these conditions can be true for the entire statement to evaluate to true. Therefore, if any of the three conditions are true, the statement will evaluate to true.
请将此代码修改为tensorflow2.7,cuda11.2版本的代码 import tensorflow as tf from tensorflow import keras from tensorflow.keras.datasets import mnist from tensorflow.keras.models import Sequential from tensorflow.keras.layers import Conv2D, MaxPooling2D, Flatten, Dense, ReLU from tensorflow.keras.utils import to_categorical # 加载MNIST数据集 (x_train, y_train), (x_test, y_test) = mnist.load_data() # 数据预处理 x_train = x_train.astype("float32") / 255.0 x_test = x_test.astype("float32") / 255.0 x_train = tf.expand_dims(x_train, axis=3) x_test = tf.expand_dims(x_test, axis=3) y_train = to_categorical(y_train, num_classes=10) y_test = to_categorical(y_test, num_classes=10) # 构建LeNet-5模型 model = Sequential([ Conv2D(6, kernel_size=3, strides=1), MaxPooling2D(pool_size=2, strides=2), ReLU(), Conv2D(16, kernel_size=3, strides=1), MaxPooling2D(pool_size=2, strides=2), ReLU(), Flatten(), Dense(units=120, activation='relu'), Dense(units=84, activation='relu'), Dense(units=10, activation='softmax') ]) # 编译模型 model.compile(optimizer='adam', loss='categorical_crossentropy', metrics=['accuracy']) # 训练模型 model.fit(x_train, y_train, batch_size=128, epochs=30, validation_data=(x_test, y_test)) # 在测试集上输出精度 test_loss, test_acc = model.evaluate(x_test, y_test) print('Test accuracy:', test_acc)
这是一个类似的 TensorFlow 2.7 和 CUDA 11.2 版本的代码示例:
```python
import tensorflow as tf
from tensorflow import keras
from tensorflow.keras.datasets import mnist
from tensorflow.keras.models import Sequential
from tensorflow.keras.layers import Conv2D, MaxPooling2D, Flatten, Dense, ReLU
from tensorflow.keras.utils import to_categorical
# Set GPU device
gpus = tf.config.experimental.list_physical_devices('GPU')
if gpus:
try:
tf.config.experimental.set_visible_devices(gpus[0], 'GPU')
tf.config.experimental.set_memory_growth(gpus[0], True)
print("Using GPU:", tf.test.gpu_device_name())
except RuntimeError as e:
print(e)
# Load MNIST dataset
(x_train, y_train), (x_test, y_test) = mnist.load_data()
# Preprocess data
x_train = x_train.astype("float32") / 255.0
x_test = x_test.astype("float32") / 255.0
x_train = tf.expand_dims(x_train, axis=3)
x_test = tf.expand_dims(x_test, axis=3)
y_train = to_categorical(y_train, num_classes=10)
y_test = to_categorical(y_test, num_classes=10)
# Define LeNet-5 model
model = Sequential([
Conv2D(6, kernel_size=3, strides=1),
MaxPooling2D(pool_size=2, strides=2),
ReLU(),
Conv2D(16, kernel_size=3, strides=1),
MaxPooling2D(pool_size=2, strides=2),
ReLU(),
Flatten(),
Dense(units=120, activation='relu'),
Dense(units=84, activation='relu'),
Dense(units=10, activation='softmax')
])
# Compile model
model.compile(optimizer='adam',
loss='categorical_crossentropy',
metrics=['accuracy'])
# Train model
model.fit(x_train, y_train, batch_size=128, epochs=30, validation_data=(x_test, y_test))
# Evaluate model on test set
test_loss, test_acc = model.evaluate(x_test, y_test)
print('Test accuracy:', test_acc)
```
这个代码实现了一个 LeNet-5 模型,用于识别 MNIST 手写数字。它使用了 TensorFlow 2.7 和 CUDA 11.2 版本,并且在 GPU 上训练模型。在代码中,我们首先检查是否有可用的 GPU 设备,并设置 TensorFlow 只使用第一个 GPU 设备。然后,我们加载 MNIST 数据集并对其进行预处理。接下来,我们定义了一个包含两个卷积层和三个全连接层的 LeNet-5 模型,并使用 Adam 优化器和交叉熵损失函数编译了模型。最后,我们在训练集上训练模型,并在测试集上评估模型的准确性。
相关推荐
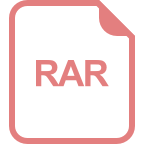














