w = torch.cat((size_tensor * torch.sqrt(ratio_tensor[0]), sizes[0] * torch.sqrt(ratio_tensor[1:]))) \ * in_height / in_width # 处理矩形输入 h = torch.cat((size_tensor / torch.sqrt(ratio_tensor[0]), sizes[0] / torch.sqrt(ratio_tensor[1:])))
时间: 2024-04-12 11:32:53 浏览: 231
这段代码是用于计算矩形输入的宽度和高度。首先,通过torch.sqrt函数计算ratio_tensor的平方根,并使用torch.cat函数将得到的张量连接起来。对于宽度w,首先计算size_tensor与ratio_tensor[0]相乘的结果,然后再与sizes[0]与ratio_tensor[1:]相乘的结果连接起来。最后,将得到的张量再与in_height除以in_width相乘。对于高度h,首先计算size_tensor与ratio_tensor[0]相除的结果,然后再与sizes[0]与ratio_tensor[1:]相除的结果连接起来。这样就得到了矩形输入的宽度和高度。
相关问题
LDAM损失函数pytorch代码如下:class LDAMLoss(nn.Module): def __init__(self, cls_num_list, max_m=0.5, weight=None, s=30): super(LDAMLoss, self).__init__() m_list = 1.0 / np.sqrt(np.sqrt(cls_num_list)) m_list = m_list * (max_m / np.max(m_list)) m_list = torch.cuda.FloatTensor(m_list) self.m_list = m_list assert s > 0 self.s = s if weight is not None: weight = torch.FloatTensor(weight).cuda() self.weight = weight self.cls_num_list = cls_num_list def forward(self, x, target): index = torch.zeros_like(x, dtype=torch.uint8) index_float = index.type(torch.cuda.FloatTensor) batch_m = torch.matmul(self.m_list[None, :], index_float.transpose(1,0)) # 0,1 batch_m = batch_m.view((16, 1)) # size=(batch_size, 1) (-1,1) x_m = x - batch_m output = torch.where(index, x_m, x) if self.weight is not None: output = output * self.weight[None, :] target = torch.flatten(target) # 将 target 转换成 1D Tensor logit = output * self.s return F.cross_entropy(logit, target, weight=self.weight) 模型部分参数如下:# 设置全局参数 model_lr = 1e-5 BATCH_SIZE = 16 EPOCHS = 50 DEVICE = torch.device('cuda:0' if torch.cuda.is_available() else 'cpu') use_amp = True use_dp = True classes = 7 resume = None CLIP_GRAD = 5.0 Best_ACC = 0 #记录最高得分 use_ema=True model_ema_decay=0.9998 start_epoch=1 seed=1 seed_everything(seed) # 数据增强 mixup mixup_fn = Mixup( mixup_alpha=0.8, cutmix_alpha=1.0, cutmix_minmax=None, prob=0.1, switch_prob=0.5, mode='batch', label_smoothing=0.1, num_classes=classes) 帮我用pytorch实现模型在模型训练中使用LDAM损失函数
好的,以下是使用LDAM损失函数进行模型训练的示例代码:
```python
import torch
import torch.nn as nn
import torch.optim as optim
import torch.nn.functional as F
from ldam_loss import LDAMLoss # 导入LDAM损失函数
# 定义模型
class MyModel(nn.Module):
def __init__(self):
super(MyModel, self).__init__()
self.conv1 = nn.Conv2d(3, 64, 3, padding=1)
self.bn1 = nn.BatchNorm2d(64)
self.conv2 = nn.Conv2d(64, 128, 3, padding=1)
self.bn2 = nn.BatchNorm2d(128)
self.conv3 = nn.Conv2d(128, 256, 3, padding=1)
self.bn3 = nn.BatchNorm2d(256)
self.fc1 = nn.Linear(256 * 4 * 4, 1024)
self.fc2 = nn.Linear(1024, 10)
def forward(self, x):
x = F.relu(self.bn1(self.conv1(x)))
x = F.max_pool2d(x, 2)
x = F.relu(self.bn2(self.conv2(x)))
x = F.max_pool2d(x, 2)
x = F.relu(self.bn3(self.conv3(x)))
x = F.max_pool2d(x, 2)
x = x.view(-1, 256 * 4 * 4)
x = F.relu(self.fc1(x))
x = self.fc2(x)
return x
# 定义数据集和数据加载器
train_dataset = ... # 定义训练集
test_dataset = ... # 定义测试集
train_loader = torch.utils.data.DataLoader(train_dataset, batch_size=16, shuffle=True)
test_loader = torch.utils.data.DataLoader(test_dataset, batch_size=16, shuffle=False)
# 定义LDAM损失函数
cls_num_list = ... # 定义每个类别的样本数量
criterion = LDAMLoss(cls_num_list, max_m=0.5, s=30)
# 定义优化器和学习率调度器
model = MyModel().to('cuda')
optimizer = optim.Adam(model.parameters(), lr=1e-3)
scheduler = optim.lr_scheduler.StepLR(optimizer, step_size=10, gamma=0.1)
# 训练模型
for epoch in range(50):
model.train()
for batch_idx, (data, target) in enumerate(train_loader):
data, target = data.to('cuda'), target.to('cuda')
data, target_a, target_b, lam = mixup_fn(data, target)
optimizer.zero_grad()
output = model(data)
loss = criterion(output, target_a, target_b, lam) # 使用LDAM损失函数
loss.backward()
nn.utils.clip_grad_norm_(model.parameters(), CLIP_GRAD) # 防止梯度爆炸
optimizer.step()
model.eval()
with torch.no_grad():
total = 0
correct = 0
for data, target in test_loader:
data, target = data.to('cuda'), target.to('cuda')
output = model(data)
_, predicted = torch.max(output.data, 1)
total += target.size(0)
correct += (predicted == target).sum().item()
acc = 100 * correct / total
print('Epoch {}: Test Acc = {}%'.format(epoch+1, acc))
scheduler.step()
```
在以上代码中,我们首先定义了一个名为`MyModel`的模型,然后定义了训练集和测试集的数据加载器。接着,我们使用LDAM损失函数来定义模型的损失函数,然后使用Adam优化器来训练模型。在每个epoch结束后,我们使用测试集来评估模型的性能,并使用学习率调度器来调整学习率。
解释代码: if i < tensor.shape[1]-2: vec_a = F.normalize(torch.tensor((tensor[0][i+2], tensor[1][i+2])) - torch.tensor((tensor[0][i], tensor[1][i])), dim=0) vec_x = torch.tensor((1.0,0)) cosa = torch.matmul(vec_x, vec_a) sina = torch.sqrt(torch.abs(1-cosa**2)) vec[2][i] = cosa vec[3][i] = sina
这段代码主要是计算给定的张量 tensor 中每一列的 cos 和 sin 值。具体的解释如下:
- 第一行的 if 语句判断变量 i 是否小于 tensor 矩阵的列数减 2,是为了避免越界。
- 第二行的 vec_a 变量计算了当前列(即第 i 列)中相邻两个点的向量,并使用 F.normalize 函数将其归一化。
- 第三行的 vec_x 变量表示基准向量(即 x 轴正方向),然后通过 torch.matmul 函数计算出 vec_x 和 vec_a 向量的点积,从而得到它们之间的夹角的 cos 值。
- 第四行的 sina 变量根据 trigonometric identity 计算出其对应的 sin 值。
- 最后两行将计算得到的 cos 和 sin 值保存在 vec 矩阵的第 2 行和第 3 行中的第 i 列中。
阅读全文
相关推荐
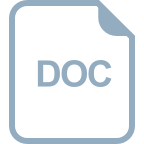
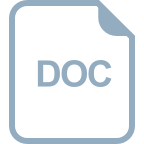
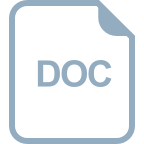












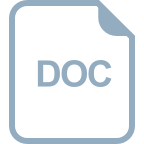
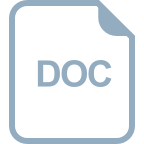