设计softmax回归模型在Fashion-MNIST数据集上实现多类别分类任务的实验目的是什么?
时间: 2024-06-02 22:08:41 浏览: 170
设计softmax回归模型在Fashion-MNIST数据集上实现多类别分类任务的实验目的是为了研究和验证softmax回归模型在图像分类任务中的表现和效果。通过该实验,我们可以了解softmax回归模型的基本原理和实现方法,以及如何使用深度学习框架(如TensorFlow、PyTorch等)构建和训练模型。此外,该实验还可以帮助我们了解Fashion-MNIST数据集,该数据集是一个常用的图像分类数据集,可以用来测试和比较不同模型的性能。最后,该实验还可以帮助我们进一步理解深度学习的基本概念和方法,为进一步研究和应用深度学习提供基础。
相关问题
softmax回归预测Fashion-MNIST图像数据集
本文将介绍如何使用softmax回归对Fashion-MNIST图像数据集进行预测。
Fashion-MNIST是一个替代MNIST手写数字集的图像数据集,用于训练和测试机器学习模型。它包含了10个类别的70,000张灰度图像,每个图像的大小为28x28像素。这些图像涵盖了从衣服、鞋子到手提包等各种物品。
为了使用softmax回归对Fashion-MNIST图像数据集进行预测,我们需要完成以下步骤:
1.加载Fashion-MNIST数据集
首先,我们需要下载并加载Fashion-MNIST数据集。可以使用以下代码块下载和加载数据集:
```
import tensorflow as tf
from tensorflow import keras
(x_train, y_train), (x_test, y_test) = keras.datasets.fashion_mnist.load_data()
```
2.预处理数据集
接下来,我们需要对数据集进行预处理。我们需要将每个图像的像素值缩放到0到1之间,并将标签转换为独热编码。可以使用以下代码块完成预处理:
```
x_train = x_train.astype('float32') / 255.0
x_test = x_test.astype('float32') / 255.0
y_train = keras.utils.to_categorical(y_train)
y_test = keras.utils.to_categorical(y_test)
```
3.构建模型
接下来,我们需要构建一个softmax回归模型。我们可以使用一个全连接层作为模型的唯一层,并将softmax函数应用于输出。可以使用以下代码块构建模型:
```
model = keras.Sequential([
keras.layers.Flatten(input_shape=(28, 28)),
keras.layers.Dense(10, activation='softmax')
])
```
4.编译模型
接下来,我们需要编译模型。我们可以使用categorical_crossentropy作为损失函数,并使用adam优化器进行优化。可以使用以下代码块编译模型:
```
model.compile(loss='categorical_crossentropy', optimizer='adam', metrics=['accuracy'])
```
5.训练模型
接下来,我们需要训练模型。我们可以使用fit方法来训练模型,并指定训练数据、批量大小、训练迭代次数和验证数据。可以使用以下代码块训练模型:
```
model.fit(x_train, y_train, batch_size=128, epochs=10, validation_data=(x_test, y_test))
```
6.评估模型
最后,我们需要评估模型。我们可以使用evaluate方法来评估模型,并指定测试数据。可以使用以下代码块评估模型:
```
loss, accuracy = model.evaluate(x_test, y_test)
print('Test accuracy:', accuracy)
```
完整代码如下:
```
import tensorflow as tf
from tensorflow import keras
(x_train, y_train), (x_test, y_test) = keras.datasets.fashion_mnist.load_data()
x_train = x_train.astype('float32') / 255.0
x_test = x_test.astype('float32') / 255.0
y_train = keras.utils.to_categorical(y_train)
y_test = keras.utils.to_categorical(y_test)
model = keras.Sequential([
keras.layers.Flatten(input_shape=(28, 28)),
keras.layers.Dense(10, activation='softmax')
])
model.compile(loss='categorical_crossentropy', optimizer='adam', metrics=['accuracy'])
model.fit(x_train, y_train, batch_size=128, epochs=10, validation_data=(x_test, y_test))
loss, accuracy = model.evaluate(x_test, y_test)
print('Test accuracy:', accuracy)
```
通过以上步骤,我们就可以使用softmax回归对Fashion-MNIST图像数据集进行预测。
利用torch.nn实现 softmax 回归在Fashion-MNIST数据集上进行训练和测试,并从loss,训 练集以及测试集上的准确率等多个角度对结果进行分析
好的,以下是使用torch.nn实现softmax回归在Fashion-MNIST数据集上进行训练和测试,并对结果进行分析的步骤:
首先,导入所需的库和模块:
```python
import torch
import torch.nn as nn
import torch.optim as optim
from torchvision import datasets, transforms
```
然后,加载Fashion-MNIST数据集并进行预处理:
```python
# 定义数据转换
transform = transforms.Compose([
transforms.ToTensor(),
transforms.Normalize((0.5,), (0.5,))
])
# 加载训练集和测试集
train_dataset = datasets.FashionMNIST(root='./data', train=True, transform=transform, download=True)
test_dataset = datasets.FashionMNIST(root='./data', train=False, transform=transform, download=True)
# 创建数据加载器
train_loader = torch.utils.data.DataLoader(dataset=train_dataset, batch_size=64, shuffle=True)
test_loader = torch.utils.data.DataLoader(dataset=test_dataset, batch_size=64, shuffle=False)
```
接下来,定义softmax回归模型:
```python
class SoftmaxRegression(nn.Module):
def __init__(self, input_dim, num_classes):
super(SoftmaxRegression, self).__init__()
self.linear = nn.Linear(input_dim, num_classes)
def forward(self, x):
out = self.linear(x)
return out
model = SoftmaxRegression(28 * 28, 10)
```
然后,定义损失函数和优化器:
```python
criterion = nn.CrossEntropyLoss()
optimizer = optim.SGD(model.parameters(), lr=0.01)
```
接着,进行模型的训练:
```python
num_epochs = 10
for epoch in range(num_epochs):
total_loss = 0
for images, labels in train_loader:
images = images.view(-1, 28 * 28)
# 前向传播
outputs = model(images)
loss = criterion(outputs, labels)
# 反向传播和优化
optimizer.zero_grad()
loss.backward()
optimizer.step()
total_loss += loss.item()
# 打印每个epoch的损失函数值
print('Epoch [{}/{}], Loss: {:.4f}'.format(epoch+1, num_epochs, total_loss))
```
最后,对模型进行测试并分析结果:
```python
# 在训练集上进行预测
correct_train = 0
total_train = 0
with torch.no_grad():
for images, labels in train_loader:
images = images.view(-1, 28 * 28)
outputs = model(images)
_, train_predicted = torch.max(outputs.data, 1)
total_train += labels.size(0)
correct_train += (train_predicted == labels).sum().item()
train_accuracy = correct_train / total_train
# 在测试集上进行预测
correct_test = 0
total_test = 0
with torch.no_grad():
for images, labels in test_loader:
images = images.view(-1, 28 * 28)
outputs = model(images)
_, test_predicted = torch.max(outputs.data, 1)
total_test += labels.size(0)
correct_test += (test_predicted == labels).sum().item()
test_accuracy = correct_test / total_test
print('Train Accuracy: {:.2f}%'.format(train_accuracy * 100))
print('Test Accuracy: {:.2f}%'.format(test_accuracy * 100))
```
通过以上步骤,我们可以使用torch.nn实现softmax回归模型在Fashion-MNIST数据集上进行训练和测试,并从loss、训练集以及测试集上的准确率等多个角度对结果进行分析。可以观察每个epoch的损失函数逐渐减小,同时计算训练集和测试集上的准确率来评估模型的性能。
阅读全文
相关推荐
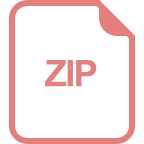
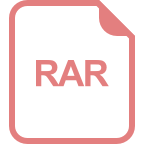
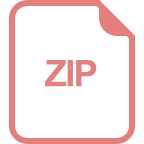
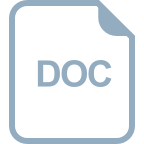






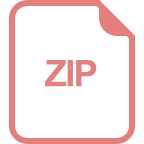
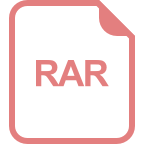
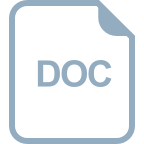
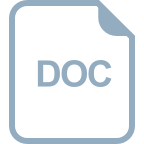



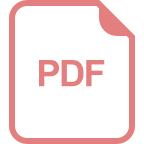