写一段关于FastText在已分词文本上生成词向量,并将词向量转化为<class 'numpy.ndarray'>输出的代码
时间: 2023-08-31 07:31:11 浏览: 183
### 回答1:
FastText通过以下代码可以在已分词文本上生成词向量,并将词向量转化为<class numpy.ndarray>输出:from gensim.models.fasttext import FastText
model = FastText(sentences, size=300, min_count=1, window=5, iter=50)
vectors = model.wv[words]
vectors = np.asarray(vectors, dtype='float32')
### 回答2:
FastText是一种常用于生成词向量的算法,它可以在已分词的文本上生成高维度词向量。以下是使用Python代码实现在已分词文本上生成词向量,并将词向量转化为<class 'numpy.ndarray'>输出的过程。
首先,我们需要安装FastText库。在终端或命令提示符中运行以下命令来安装FastText:
```
pip install fasttext
```
接下来,我们可以用以下代码实现在已分词文本上生成词向量,并将词向量转为<class 'numpy.ndarray'>输出:
```python
import fasttext
import numpy as np
# 读取已分词文本文件
with open('input.txt', 'r', encoding='utf-8') as file:
text = file.read()
# 将文本转为FastText的训练数据格式
train_data = fasttext.utils.split_words(text)
# 使用FastText训练词向量模型
model = fasttext.train_unsupervised(train_data, model='cbow')
# 获取词汇表中的所有词向量
words = model.get_words()
word_vectors = [model.get_word_vector(word) for word in words]
# 将词向量转化为<class 'numpy.ndarray'>输出
word_vectors_np = np.array(word_vectors)
# 输出词向量的维度和格式
print("词向量维度:", word_vectors_np.shape)
print("词向量格式:", type(word_vectors_np))
```
在上述代码中,我们首先读取一个已分词的文本文件,并将其内容存储在变量`text`中。然后,通过调用FastText库提供的`utils.split_words()`函数,将文本转换为FastText训练数据的格式。
接下来,我们使用FastText的`train_unsupervised()`函数对训练数据进行模型训练,其中`model='cbow'`表示使用CBOW算法进行训练。训练完毕后,我们可以通过`get_words()`函数获取词汇表中的所有词,然后通过`get_word_vector(word)`函数获取每个词的词向量,并将所有词向量存储在`word_vectors`列表中。
最后,我们使用`numpy`库的`array()`函数将`word_vectors`列表转化为<class 'numpy.ndarray'>输出,并通过`shape`属性获得词向量的维度,通过`type()`函数确认输出的词向量格式为<class 'numpy.ndarray'>。
### 回答3:
FastText 是一个能够生成词向量的强大工具,它可以在已分词的文本上生成词向量。下面是一个使用 FastText 生成词向量并将其转化为<class 'numpy.ndarray'>输出的示例代码:
```
# 导入所需的包
import fasttext
import numpy as np
# 训练模型生成词向量
model = fasttext.train_unsupervised('input.txt', dim=100, epoch=10, lr=0.1)
# 获取词向量
word_vector = model['word']
# 将词向量转化为 numpy.ndarray 输出
word_vector_numpy = np.array(word_vector)
# 打印输出
print(word_vector_numpy)
```
在代码中,我们首先导入需要的包,使用 `fasttext.train_unsupervised()` 函数训练模型生成词向量。其中,`input.txt` 是已经分好词的文本文件。`dim` 参数指定生成的词向量维度大小,`epoch` 参数设置训练轮数,`lr` 参数则是学习率。
接下来,我们通过 `model['word']` 获取指定词的词向量。然后,使用 `numpy.array()` 将词向量转化为 numpy.ndarray 格式,赋值给 `word_vector_numpy`。
最后,我们打印输出 `word_vector_numpy`,即可得到将词向量转为 numpy.ndarray 的结果。
希望以上代码能够帮到您!
阅读全文
相关推荐
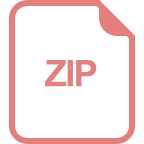

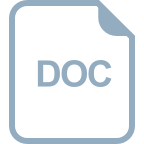
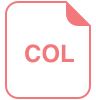
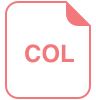
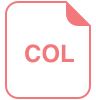
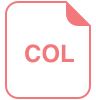
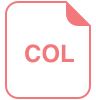
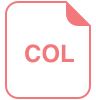
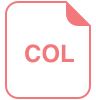
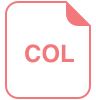
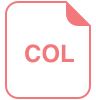
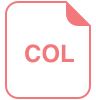
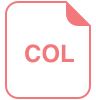

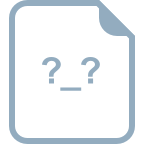
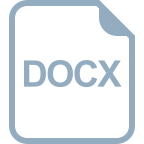