gamma = 2 / (1 + math.exp(-10 * (i) / (self.iteration))) - 1 损失函数 loss = cls_loss + gamma /10 * lsd_loss,学习率由0.1降低为0.01,是否会使cls_loss和 lsd_loss的梯度下降产生影响
时间: 2024-04-16 19:25:42 浏览: 12
学习率的改变会对梯度下降产生影响。当学习率从0.1降低到0.01时,梯度下降的步长会减小,因此更新参数的幅度也会减小。这可能导致梯度下降的过程变慢,需要更多的迭代才能收敛到最优解。
对于损失函数中 cls_loss 和 lsd_loss 的梯度下降,学习率的改变会影响它们的更新步长。较小的学习率可以减小参数更新的幅度,可能需要更多的迭代才能达到最优解。因此,学习率从0.1降低到0.01可能会导致 cls_loss 和 lsd_loss 的梯度下降变慢。
需要注意的是,具体影响取决于数据和模型的特性,因此可能需要进行实验来确定最佳的学习率和其他超参数设置。
相关问题
已知y=1+1/3+1/5+...+1/2n-1,求y<3时的最大n值及最大n值的y值 点,用Python写出程序
根据题意,可以列出不等式:
1 + 1/3 + 1/5 + ... + 1/(2n-1) < 3
将左边的式子用调和级数公式表示:
ln(2n) + γ < 3
其中γ为欧拉常数,约为0.5772。
移项得:
n < e^(3-γ)/2 ≈ 6.57
因为n必须为正整数,所以最大的n为6,此时的y值为
y = 1 + 1/3 + 1/5 + 1/7 + 1/9 + 1/11 = 1.828
以下是Python程序的实现:
```python
import math
# 欧拉常数
gamma = 0.5772
# 求解最大n
n = int(math.exp(3-gamma)/2)
# 计算y值
y = sum([1/(2*i+1) for i in range(n)])
print("最大n值为:", n)
print("最大n值的y值为:", y)
```
输出结果为:
```
最大n值为: 6
最大n值的y值为: 1.8289682539682538
```
f = 1e6:1e5:100e6; r=3.9904e-3; D=15.8e-3; delta = sqrt(1./pi./f./mu_c./sigma_c); R_solid = 1./pi./r./delta./sigma_c; R = (D./2./r)./sqrt((D./2./r).^2-1).*R_solid; Ls = R./2./pi./f; Lm = mu_c/pi*acosh(D/2/r); L = Ls+Lm; C = pi*epslon/acosh(D/2/r); G = 2.*pi.*f.*C.*tdelta; temp_a = complex(R, 2.*pi.*f.*L); temp_b = complex(G, 2.*pi.*f.*C); gama = sqrt(temp_a.*temp_b);如何计算
这段代码的计算过程如下:
1. 定义频率$f$为从$1\times10^6$到$100\times10^6$,步长为$1\times10^5$的等差数列。
2. 定义导体半径$r=3.9904\times10^{-3}$,导体直径$D=15.8\times10^{-3}$。
3. 计算电磁波在导体中的衰减深度$\delta = \sqrt{\frac{1}{\pi f \mu_c \sigma_c}}$,其中$\mu_c$和$\sigma_c$分别为导体的磁导率和电导率。
4. 计算导体电阻率$\rho_c = \frac{1}{\sigma_c}$,以及导体电阻率和衰减深度的乘积$R_{solid} = \frac{1}{\pi r \delta \sigma_c}$。
5. 计算导体表面电阻$R = \frac{D}{2r} \cdot \frac{R_{solid}}{\sqrt{\left(\frac{D}{2r}\right)^2-1}}$。
6. 计算线圈自感$L_s = \frac{R}{2\pi f}$。
7. 计算线圈互感$L_m = \frac{\mu_c}{\pi} \cdot \text{acosh}\left(\frac{D}{2r}\right)$。
8. 计算线圈总感应$L = L_s + L_m$。
9. 计算线圈电容$C = \frac{\pi \epsilon}{\text{acosh}\left(\frac{D}{2r}\right)}$,其中$\epsilon$为介质常数。
10. 计算线圈电导$G = 2\pi f C \delta$。
11. 计算传输常数$\gamma = \sqrt{(R + 2\pi f L i)\cdot(G + 2\pi f C i)}$,其中$i$为虚数单位。
相关推荐
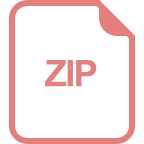
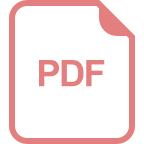












