def __backward(self, dout): dbeta = dout.sum(axis=0) dgamma = np.sum(self.xn * dout, axis=0) dxn = self.gamma * dout dxc = dxn / self.std dstd = -np.sum((dxn * self.xc) / (self.std * self.std), axis=0) dvar = 0.5 * dstd / self.std dxc += (2.0 / self.batch_size) * self.xc * dvar dmu = np.sum(dxc, axis=0) dx = dxc - dmu / self.batch_size self.dgamma = dgamma self.dbeta = dbeta return dx
时间: 2024-02-14 13:22:32 浏览: 212
这段代码是在批量归一化层中进行反向传播的私有方法。它首先计算反向传播过程中需要用到的一些中间变量,包括 dbeta 和 dgamma,它们分别是输入梯度 dout 对于平移参数 beta 和缩放参数 gamma 的梯度。然后,它计算 dxn、dxc、dstd、dvar、dmu 和 dx,它们分别是输入梯度 dout 对于标准化后的张量 xn、标准化前的张量 xc、标准差 std、方差 var、均值 mu 和输入张量 x 的梯度。最后,它将 dgamma 和 dbeta 分别保存在 self.dgamma 和 self.dbeta 中,并返回输入张量 x 的梯度 dx。
相关问题
运行class GuidedBackpropReLUModel: def __init__(self, model, use_cuda): self.model = model self.model.eval() self.cuda = use_cuda if self.cuda: self.model = model.cuda() for idx, module in self.model.features._modules.items(): if module.__class__.__name__ == 'ReLU': self.model.features._modules[idx] = GuidedBackpropReLU() def forward(self, input): return self.model(input) def forward_static(self, input): if self.cuda: output = self.forward(input.cuda()) else: output = self.forward(input) return output def __call__(self, input, index = None): output = self.forward_static(input) if index == None: index = np.argmax(output.cpu().data.numpy()) one_hot = np.zeros((1, output.size()[-1]), dtype = np.float32) one_hot[0][index] = 1 one_hot = Variable(torch.from_numpy(one_hot), requires_grad = True) if self.cuda: one_hot = torch.sum(one_hot.cuda() * output) else: one_hot = torch.sum(one_hot * output) one_hot.backward() output = input.grad.cpu().data.numpy() output = output[0,:,:,:] return output报错Legacy autograd function with non-static forward method is deprecated. Please use new-style autograd function with static forward method. 如何修改代码
这个错误提示是因为代码中使用了旧版本的autograd函数,需要使用新版本的autograd函数。可以使用`@staticmethod`修饰`GuidedBackpropReLU`类中的`forward`方法,将其转换为静态方法。修改后的代码如下:
```
class GuidedBackpropReLU(nn.ReLU):
@staticmethod
def forward(input):
output = torch.clamp(input, min=0.0)
grad = torch.zeros_like(output)
grad[output > 0] = 1.0
output = output * grad
return output
class GuidedBackpropReLUModel:
def __init__(self, model, use_cuda):
self.model = model
self.model.eval()
self.cuda = use_cuda
if self.cuda:
self.model = model.cuda()
for idx, module in self.model.features._modules.items():
if module.__class__.__name__ == 'ReLU':
self.model.features._modules[idx] = GuidedBackpropReLU()
def forward(self, input):
return self.model(input)
def forward_static(self, input):
if self.cuda:
output = self.forward(input.cuda())
else:
output = self.forward(input)
return output
def __call__(self, input, index=None):
output = self.forward_static(input)
if index is None:
index = np.argmax(output.cpu().data.numpy())
one_hot = np.zeros((1, output.size()[-1]), dtype=np.float32)
one_hot[0][index] = 1
one_hot = Variable(torch.from_numpy(one_hot), requires_grad=True)
if self.cuda:
one_hot = torch.sum(one_hot.cuda() * output)
else:
one_hot = torch.sum(one_hot * output)
one_hot.backward()
output = input.grad.cpu().data.numpy()
output = output[0, :, :, :]
return output
```
class GuidedBackpropReLUModel: def init(self, model, use_cuda): self.model = model self.model.eval() self.cuda = use_cuda if self.cuda: self.model = model.cuda() for idx, module in self.model.features._modules.items(): if module.class.name == 'ReLU': self.model.features._modules[idx] = GuidedBackpropReLU() def forward(self, input): model1 = nn.Sequential(*list(self.model.children())[1:]) return model1(input) def forward_static(self, input): if self.cuda: output = self.forward(input.cuda()) else: output = self.forward(input) return output def call(self, input, index=None): output = self.forward_static(input) if index is None: index = np.argmax(output.cpu().data.numpy()) one_hot = np.zeros((1, output.size()[-1]), dtype=np.float32) one_hot[0][index] = 1 one_hot = Variable(torch.from_numpy(one_hot), requires_grad=True) if self.cuda: one_hot = torch.sum(one_hot.cuda() * output) else: one_hot = torch.sum(one_hot * output) one_hot.backward() output = input.grad.cpu().data.numpy() output = output[0, :, :, :] return output 代码中input.gard为空怎么解决,代码如何修改
你的代码中有一个小错误,`__init__` 方法的名称应该是 `__init__` 而不是 `init`。
以下是修改后的代码,其中通过调用 `input.requires_grad_(True)` 将输入的 `input` 设置为需要计算梯度,从而解决了 `input.grad` 为空的问题:
```
class GuidedBackpropReLUModel:
def __init__(self, model, use_cuda):
self.model = model
self.model.eval()
self.cuda = use_cuda
if self.cuda:
self.model = model.cuda()
for idx, module in self.model.features._modules.items():
if module.__class__.__name__ == 'ReLU':
self.model.features._modules[idx] = GuidedBackpropReLU()
def forward(self, input):
model1 = nn.Sequential(*list(self.model.children())[1:])
return model1(input)
def forward_static(self, input):
if self.cuda:
output = self.forward(input.cuda())
else:
output = self.forward(input)
return output
def __call__(self, input, index=None):
input.requires_grad_(True) # 将输入设置为需要计算梯度
output = self.forward_static(input)
if index is None:
index = np.argmax(output.cpu().data.numpy())
one_hot = np.zeros((1, output.size()[-1]), dtype=np.float32)
one_hot[0][index] = 1
one_hot = Variable(torch.from_numpy(one_hot), requires_grad=True)
if self.cuda:
one_hot = torch.sum(one_hot.cuda() * output)
else:
one_hot = torch.sum(one_hot * output)
one_hot.backward()
output = input.grad.cpu().data.numpy()
output = output[0, :, :, :]
return output
```
阅读全文
相关推荐
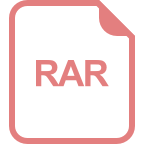
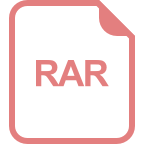
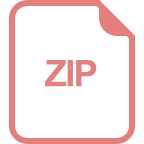














