json_str = re.findall('g_page_config = (.*);', html_data)[0] IndexError: list index out of range
时间: 2024-04-15 17:26:19 浏览: 27
这个错误提示表明在使用正则表达式 `re.findall` 提取数据时,没有找到与给定模式匹配的数据。导致这个错误的原因可能是:
1. 正则表达式模式不匹配:确保正则表达式模式与要提取的数据匹配。可能是模式中存在错误或不完整,导致无法成功匹配到目标字符串。
2. HTML 数据不包含目标字符串:确认 HTML 数据中是否包含你想要提取的目标字符串。可以先打印出 `html_data` 的内容,检查其中是否存在 `g_page_config`。
3. 数据提取位置错误:如果目标字符串在 HTML 数据中的位置不是唯一的,你可能需要调整提取位置。可能需要改变提取的方式,或者使用更精确的正则表达式来匹配目标字符串。
以下是一些调试步骤,可以帮助你解决这个问题:
1. 检查正则表达式模式:确保你的正则表达式模式正确,并且与目标字符串的格式匹配。
2. 检查 HTML 数据:打印出 `html_data` 的内容,检查其中是否包含你要提取的字符串。
3. 尝试使用其他提取方法:如果使用正则表达式无法成功提取数据,可以尝试使用其他方法(如字符串操作、JSON 解析库等)来提取目标数据。
4. 调整提取位置:如果目标字符串在 HTML 数据中的位置不唯一,可以尝试调整提取的位置,确保提取的数据是正确的。
请注意,这只是一些常见的解决方法,具体解决方案可能因情况而异。如果问题仍然存在,请提供更多的代码和数据上下文,以便我能更好地帮助你。
相关问题
video_url=re.findall(ex,detail_url_text)[0] IndexError: list index out of range
这个错误通常意味着你正在尝试访问一个列表中不存在的索引位置。这可能是由于列表为空或索引超出了列表的范围而导致的。
要解决此错误,您可以尝试以下几个步骤:
1. 确保列表不为空。您可以使用 `print` 语句或调试器检查列表中的内容。
2. 确保您正在尝试访问正确的索引位置。请注意,Python 中的列表索引从 0 开始,因此最后一个元素的索引为 `len(list)-1`。
3. 如果列表的长度是可变的,请确保您的索引位置不会超出列表的范围。您可以使用 `len` 函数获取列表的长度,并根据需要进行检查。
4. 如果您的代码中存在循环结构,请确保循环不会在列表为空的情况下继续执行,否则会导致类似的错误。
希望这些提示可以帮助您解决问题。
json_result = json.loads(re.findall("<return>(.*?)</return>", response.text)[0])可能有越界,请修改一下
你说得对,如果正则表达式没有匹配到结果,那么在调用索引[0]时会出现IndexError异常。为了避免这种情况,可以在调用索引之前先判断列表的长度是否大于0。以下是修改后的代码:
```
result_list = re.findall("<return>(.*?)</return>", response.text)
if len(result_list) > 0:
json_result = json.loads(result_list[0])
else:
json_result = {}
```
这样就可以避免IndexError异常了。
相关推荐
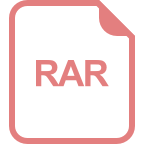
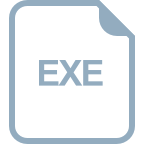
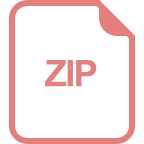












