根据矩阵绘制有向加权网络
时间: 2024-02-03 20:01:42 浏览: 70
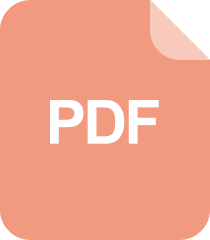
Python根据已知邻接矩阵绘制无向图操作示例
根据矩阵绘制有向加权网络可以使用Python中的networkx和matplotlib库。以下是一个简单的例子:
```python
import networkx as nx
import matplotlib.pyplot as plt
import numpy as np
# 创建邻接矩阵
matrix = np.array([[0, 0.6, 0.2, 0],
[0, 0, 0, 0],
[0, 0, 0, 0.9],
[0.3, 0, 0, 0]])
# 创建有向加权图
G = nx.DiGraph(matrix)
# 绘制有向加权图
pos = nx.spring_layout(G)
edges = G.edges()
weights = [G[u][v]['weight'] for u,v in edges]
nx.draw_networkx_edges(G, pos, edgelist=edges, width=weights)
nx.draw_networkx_nodes(G, pos)
nx.draw_networkx_labels(G, pos)
# 显示图像
plt.show()
```
这里使用了邻接矩阵来创建有向加权图,可以根据实际需要调整矩阵的值。另外,同样可以根据需要调整节点和边的大小、形状、颜色等参数。
阅读全文
相关推荐
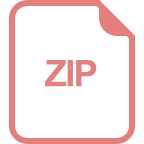
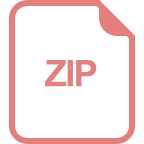















