下面是我的模型部分定义,如果加入ResNet,应该怎么加 : lstm2 = LSTM(128, return_sequences=True)(context_dim2) # 加入LSTM层 concat = concatenate([lstm1,lstm2]) # 增加一个TimeDistributed层,以便对每个时间步进行相同的处理 td = TimeDistributed(Dense(128, activation='relu'))(concat) td = TimeDistributed(Dropout(0.2))(td) lstm_out = LSTM(64, return_sequences=False)(td) # 加入LSTM层 lstm_out = Dense(32, activation='relu')(lstm_out) lstm_out = Dense(16, activation='tanh')(lstm_out) res = Dense(trainY.shape[1])(lstm_out)
时间: 2024-01-12 19:05:20 浏览: 59
如果要在您现有的模型中加入ResNet块,可以按照以下步骤进行:
1. 将输入数据连接到第一个LSTM层之前,以便保持原始输入的信息。具体来说,可以使用Keras中的Input层来定义输入数据,然后将其连接到LSTM层之前的卷积层中。
2. 定义ResNet块,将其插入到两个LSTM层之间。具体来说,可以按照ResNet块的结构,在两个LSTM层之间添加两个卷积层和一个跳跃连接。跳跃连接将输入数据直接连接到输出数据上,以便保留原始输入的信息。
3. 将ResNet块的输出连接到第二个LSTM层之前的Dense层中,以便进行下一步的处理。
4. 继续定义模型的输出层,并编译模型。
下面是一种可能的实现方法:
```python
# 定义输入层
input_layer = Input(shape=(input_shape,))
# 定义卷积层和ResNet块
conv1 = Conv1D(64, kernel_size=3, padding="same")(input_layer)
bn1 = BatchNormalization()(conv1)
act1 = Activation("relu")(bn1)
conv2 = Conv1D(64, kernel_size=3, padding="same")(act1)
bn2 = BatchNormalization()(conv2)
shortcut = input_layer
add1 = Add()([bn2, shortcut])
act2 = Activation("relu")(add1)
# 定义LSTM层和TimeDistributed层
lstm1 = LSTM(128, return_sequences=True)(act2)
context_dim2 = Input(shape=(time_steps, feature_dim))
lstm2_input = TimeDistributed(Dense(64))(context_dim2)
concat = concatenate([lstm1, lstm2_input])
td = TimeDistributed(Dense(128, activation='relu'))(concat)
td = TimeDistributed(Dropout(0.2))(td)
# 定义ResNet块
conv3 = Conv1D(64, kernel_size=3, padding="same")(td)
bn3 = BatchNormalization()(conv3)
act3 = Activation("relu")(bn3)
conv4 = Conv1D(64, kernel_size=3, padding="same")(act3)
bn4 = BatchNormalization()(conv4)
add2 = Add()([bn4, td])
act4 = Activation("relu")(add2)
# 定义LSTM层和输出层
lstm_out = LSTM(64, return_sequences=False)(act4)
lstm_out = Dense(32, activation='relu')(lstm_out)
lstm_out = Dense(16, activation='tanh')(lstm_out)
output_layer = Dense(trainY.shape[1])(lstm_out)
# 定义模型
model = Model(inputs=[input_layer, context_dim2], outputs=output_layer)
# 编译模型
model.compile(optimizer='adam', loss='mse')
```
需要注意的是,这只是一种可能的实现方法,具体的实现方式可能会因为数据集的不同而有所变化。您需要根据自己的情况进行调整和优化。
相关推荐
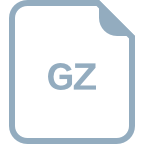
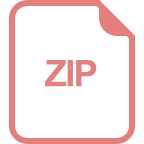
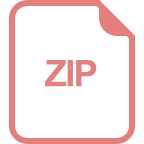














