data={'scores':[random.randint(50,100) for i in range(20)]}
时间: 2024-02-29 07:57:24 浏览: 180
这段代码使用了 Python 的列表推导式,生成了一个包含 20 个随机整数的列表,每个整数的取值范围在 50 到 100 之间(包括 50 和 100)。然后将这个列表作为值,以 'scores' 为键,存储在一个字典变量 data 中。这段代码的作用是生成一个包含 20 个随机成绩的数据集,可以用于后续的数据分析和处理。
相关问题
以下这段代码是关于CatBoost模型的超参数调整,但里面好像不是在五倍交叉验证下做的分析,请问应该怎么加上五倍交叉验证呢?import os import time import pandas as pd from catboost import CatBoostRegressor from hyperopt import fmin, hp, partial, Trials, tpe,rand from sklearn.metrics import r2_score, mean_squared_error from sklearn.model_selection import train_test_split from sklearn.model_selection import KFold, cross_val_score as CVS, train_test_split as TTS 自定义hyperopt的参数空间 space = {"iterations": hp.choice("iterations", range(1, 30)), "depth": hp.randint("depth", 16), "l2_leaf_reg": hp.randint("l2_leaf_reg", 222), "border_count": hp.randint("border_count", 222), 'learning_rate': hp.uniform('learning_rate', 0.001, 0.9), } data = pd.read_csv(r"E:\exercise\synthesis\synthesis_dummy_2.csv") #验证随机森林填补缺失值方法是否有效 X = data.iloc[:,1:] y = data.iloc[:,0] Xtrain,Xtest,Ytrain,Ytest = TTS(X_wrapper,y,test_size=0.2,random_state=100) def epoch_time(start_time, end_time): elapsed_secs = end_time - start_time elapsed_mins = elapsed_secs / 60 return elapsed_mins, elapsed_secs 自动化调参并训练 def cat_factory(argsDict): estimator = CatBoostRegressor(loss_function='RMSE', random_seed=22, learning_rate=argsDict['learning_rate'], iterations=argsDict['iterations'], l2_leaf_reg=argsDict['l2_leaf_reg'], border_count=argsDict['border_count'], depth=argsDict['depth'], verbose=0) estimator.fit(Xtrain, Ytrain) val_pred = estimator.predict(Xtest) mse = mean_squared_error(Ytest, val_pred) return mse
要在这段代码中添加五倍交叉验证,你可以按照以下步骤进行修改:
1. 导入所需的包:
```python
from sklearn.model_selection import KFold, cross_val_score
```
2. 设置五倍交叉验证的参数:
```python
n_folds = 5
```
3. 修改数据划分部分,将数据划分为训练集和测试集:
```python
Xtrain, Xtest, Ytrain, Ytest = train_test_split(X_wrapper, y, test_size=0.2, random_state=100)
```
4. 修改自动化调参并训练的函数,使用五倍交叉验证进行模型评估:
```python
def cat_factory(argsDict):
estimator = CatBoostRegressor(loss_function='RMSE', random_seed=22,
learning_rate=argsDict['learning_rate'],
iterations=argsDict['iterations'],
l2_leaf_reg=argsDict['l2_leaf_reg'],
border_count=argsDict['border_count'],
depth=argsDict['depth'], verbose=0)
# 设置五倍交叉验证
kf = KFold(n_splits=n_folds)
mse_scores = []
for train_index, val_index in kf.split(Xtrain):
X_train, X_val = Xtrain.iloc[train_index], Xtrain.iloc[val_index]
Y_train, Y_val = Ytrain.iloc[train_index], Ytrain.iloc[val_index]
estimator.fit(X_train, Y_train)
val_pred = estimator.predict(X_val)
mse = mean_squared_error(Y_val, val_pred)
mse_scores.append(mse)
mse_mean = np.mean(mse_scores)
return mse_mean
```
5. 修改自定义hyperopt的参数空间部分,将模型评估函数替换为修改后的函数:
```python
space = {"iterations": hp.choice("iterations", range(1, 30)),
"depth": hp.randint("depth", 16),
"l2_leaf_reg": hp.randint("l2_leaf_reg", 222),
"border_count": hp.randint("border_count", 222),
'learning_rate': hp.uniform('learning_rate', 0.001, 0.9),
}
trials = Trials()
best = fmin(fn=cat_factory, space=space, algo=tpe.suggest, max_evals=100, trials=trials)
```
这样,你就在代码中添加了五倍交叉验证来评估CatBoost模型的超参数调整效果。
将以下适用于pt模型的代码改为适用于tflite模型的代码weights = r'weights/best.pt' # 指定设备,如果是 'cpu' 则使用 CPU,如果是 '0' 则使用 GPU 0,以此类推 opt_device = '' device = select_device(opt_device) # 指定图片大小 imgsz = 640 # 指定置信度和 IOU 阈值 opt_conf_thres = 0.6 opt_iou_thres = 0.45 # 初始化日志记录 set_logging() # 加载模型 model = attempt_load(weights, map_location=device) # 检查图片大小是否符合要求,如果不符合则调整 imgsz = check_img_size(imgsz, s=model.stride.max()) # 如果设备支持半精度 FP16,则将模型转换为半精度 half = device.type != 'cpu' if half: model.half() # 获取预测结果中标签的名字和颜色,分别存储在 names 和 colors 中 names = model.module.names if hasattr(model, 'module') else model.names colors = [[random.randint(0, 255) for _ in range(3)] for _ in names]
由于tflite模型和pt模型在结构上存在显著差异,因此需要重新编写代码以适用于tflite模型。具体来说,以下是你可以考虑的更改:
1. 通过使用 TensorFlow Lite Python Interpreter 加载和运行模型,而不是使用 PyTorch 的相关功能。
2. 更改模型路径和设备参数,以适应 TensorFlow Lite 模型。
3. 更改检查图像大小和转换模型精度的代码。
4. 修改获取预测结果中标签名称和颜色的方式,以适应 TensorFlow Lite 模型。
下面是一种可能的修改:
```python
import numpy as np
import random
import cv2
import tensorflow as tf
# 指定模型路径
model_path = 'weights/best.tflite'
# 指定输入和输出张量名称
input_details = None
output_details = None
# 加载模型
interpreter = tf.lite.Interpreter(model_path=model_path)
interpreter.allocate_tensors()
# 获取输入和输出张量名称
input_details = interpreter.get_input_details()
output_details = interpreter.get_output_details()
# 检查图片大小是否符合要求,如果不符合则调整
imgsz = 640
if input_details[0]['shape'][1] != imgsz:
interpreter.resize_tensor_input(input_details[0]['index'], (1, imgsz, imgsz, 3))
interpreter.allocate_tensors()
# 初始化标签名称和颜色
names = ['person', 'car', 'truck', 'bus'] # 假设一共有四个类别
colors = [[random.randint(0, 255) for _ in range(3)] for _ in names]
# 进行推理
def detect(image):
# 对图像进行预处理
image = cv2.cvtColor(image, cv2.COLOR_BGR2RGB)
image = cv2.resize(image, (imgsz, imgsz))
input_data = np.expand_dims(image, axis=0)
input_data = (np.float32(input_data) - 127.5) / 127.5
# 运行模型
interpreter.set_tensor(input_details[0]['index'], input_data)
interpreter.invoke()
output_data = interpreter.get_tensor(output_details[0]['index'])
# 对输出进行后处理
output_data = np.squeeze(output_data)
boxes = output_data[:, :4]
scores = output_data[:, 4]
classes = output_data[:, 5].astype(int)
# 过滤掉置信度较低的检测结果
keep = np.where(scores > 0.6)[0]
boxes = boxes[keep]
scores = scores[keep]
classes = classes[keep]
# 进行非极大值抑制 (NMS) 处理
indices = cv2.dnn.NMSBoxes(boxes, scores, 0.6, 0.45)
# 构造最终的检测结果
results = []
for i in indices:
i = i[0]
box = boxes[i]
score = scores[i]
cls = classes[i]
label = names[cls]
color = colors[cls]
x1, y1, x2, y2 = box.astype(int)
results.append({
'label': label,
'score': float(score),
'box': [x1, y1, x2, y2],
'color': color
})
return results
```
请注意,这只是一个示例代码,你可能需要对其进行一些修改,以适应你的具体情况。
阅读全文
相关推荐
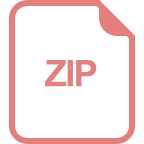
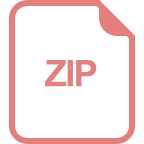
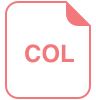
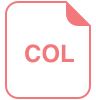
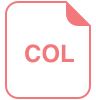
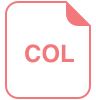
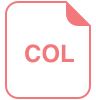
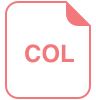
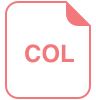
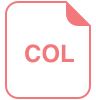






