用python实现双向RRT算法
时间: 2024-03-28 18:34:41 浏览: 75
双向RRT算法是一种用于路径规划的算法,它通过同时从起点和终点开始构建两棵树,直到两棵树相交或找到一条连接两棵树的路径。下面是用Python实现双向RRT算法的基本步骤:
1. 定义节点类:首先,我们需要定义一个节点类来表示树中的节点。节点类通常包含节点的坐标、父节点等信息。
2. 初始化树:创建两棵树,分别从起点和终点开始。起点和终点分别作为两棵树的根节点。
3. 扩展树:在每次迭代中,从起点树和终点树中选择一个树进行扩展。选择的方法可以是随机选择或者根据一定的启发式规则选择。
4. 生成新节点:在选择的树中,生成一个新的节点。可以通过随机采样或者使用启发式规则生成新节点。
5. 连接节点:将新生成的节点与树中最近的节点进行连接。可以使用欧氏距离或其他距离度量方法来计算最近的节点。
6. 检查碰撞:检查连接的路径是否与障碍物相交。如果相交,则舍弃该路径。
7. 更新树:将新生成的节点添加到选择的树中,并更新节点的父节点。
8. 判断终止条件:判断两棵树是否相交,或者是否找到了一条连接两棵树的路径。如果满足终止条件,则算法结束。
9. 生成路径:如果找到了连接两棵树的路径,从起点树和终点树中分别沿着父节点指针回溯,生成最终的路径。
下面是一些相关问题:
相关问题
双向rrt算法python代码
以下是双向RRT算法的Python代码示例:
```python
import random
import math
import matplotlib.pyplot as plt
class RRTNode:
def __init__(self, x, y):
self.x = x
self.y = y
self.parent = None
class BiRRT:
def __init__(self, start, goal, obstacle_list, rand_area, expand_dist=0.5, goal_sample_rate=20, max_iter=500):
self.start_node = RRTNode(start[0], start[1])
self.goal_node = RRTNode(goal[0], goal[1])
self.obstacle_list = obstacle_list
self.min_rand = rand_area[0]
self.max_rand = rand_area[1]
self.expand_dist = expand_dist
self.goal_sample_rate = goal_sample_rate
self.max_iter = max_iter
self.node_list1 = [self.start_node]
self.node_list2 = [self.goal_node]
self.path = []
def planning(self):
for i in range(self.max_iter):
if random.randint(0, 100) > self.goal_sample_rate:
rnd = self.get_random_node()
else:
rnd = self.goal_node
nearest_node1 = self.nearest_node(self.node_list1, rnd)
nearest_node2 = self.nearest_node(self.node_list2, rnd)
new_node1 = self.steer(nearest_node1, rnd, self.expand_dist)
new_node2 = self.steer(nearest_node2, rnd, self.expand_dist)
if self.check_collision(new_node1) and self.check_collision(new_node2):
self.node_list1.append(new_node1)
self.node_list2.append(new_node2)
self.connect(new_node1, new_node2)
if self.calc_dist(new_node1, self.goal_node) < self.expand_dist:
final_node = self.steer(new_node1, self.goal_node, self.expand_dist)
if self.check_collision(final_node):
self.path = self.get_path(final_node)
return self.path
self.node_list1, self.node_list2 = self.node_list2, self.node_list1
def steer(self, from_node, to_node, extend_length=float("inf")):
new_node = RRTNode(from_node.x, from_node.y)
dist, angle = self.calc_distance_and_angle(new_node, to_node)
if dist < extend_length:
extend_length = dist
new_node.x += extend_length * math.cos(angle)
new_node.y += extend_length * math.sin(angle)
new_node.parent = from_node
return new_node
def connect(self, from_node, to_node):
from_node.parent = to_node
def get_path(self, node):
path = [[node.x, node.y]]
while node.parent:
node = node.parent
path.append([node.x, node.y])
return path
def nearest_node(self, node_list, node):
nearest_node = node_list[0]
for n in node_list:
if self.calc_dist(n, node) < self.calc_dist(nearest_node, node):
nearest_node = n
return nearest_node
def calc_dist(self, from_node, to_node):
return math.sqrt((from_node.x - to_node.x) ** 2 + (from_node.y - to_node.y) ** 2)
def calc_distance_and_angle(self, from_node, to_node):
dx = to_node.x - from_node.x
dy = to_node.y - from_node.y
dist = math.sqrt(dx ** 2 + dy ** 2)
angle = math.atan2(dy, dx)
return dist, angle
def check_collision(self, node):
for (ox, oy, size) in self.obstacle_list:
if math.sqrt((node.x - ox) ** 2 + (node.y - oy) ** 2) <= size:
return False
return True
def get_random_node(self):
if random.randint(0, 100) > self.goal_sample_rate:
rnd = RRTNode(random.uniform(self.min_rand, self.max_rand), random.uniform(self.min_rand, self.max_rand))
else:
rnd = self.goal_node
return rnd
def plot_path(self):
plt.plot([x for (x, y) in self.path], [y for (x, y) in self.path], '-r')
for (ox, oy, size) in obstacleList:
circle = plt.Circle((ox, oy), size, color='b')
plt.gcf().gca().add_artist(circle)
plt.plot(self.start_node.x, self.start_node.y, 'xr')
plt.plot(self.goal_node.x, self.goal_node.y, 'xr')
plt.axis('equal')
plt.show()
if __name__ == '__main__':
obstacleList = [(5, 5, 1), (3, 6, 2), (3, 8, 2), (5, 10, 2), (7, 9, 2), (9, 10, 2)]
start = [0.0, 0.0]
goal = [10.0, 10.0]
rrt = BiRRT(start, goal, obstacleList, [-2, 12])
path = rrt.planning()
if path:
print(path)
rrt.plot_path()
else:
print("Cannot find path!")
```
请注意,此示例仅用于演示双向RRT算法,并且可能需要根据您的具体需求进行修改。
双向rrt算法路径规划python
双向RRT(Rapidly-exploring Random Tree)算法是一种用于路径规划的算法,它通过构建两棵树来搜索起始点和目标点之间的路径。下面是双向RRT算法路径规划的Python实现介绍:
1. 初始化:定义起始点和目标点,并创建两棵树,分别从起始点和目标点开始生长。
2. 生长树:在每次迭代中,随机生成一个点,并在树中找到最近的节点。然后,从该最近节点沿着随机生成的点方向生长一段距离,直到达到树中的一个新节点。
3. 连接树:在每次迭代中,检查两棵树是否相交。如果相交,则找到相交点,并将两棵树连接起来,形成一条路径。
4. 判断终止条件:当两棵树连接成功,或者达到最大迭代次数时,停止算法。
5. 生成路径:从连接成功的节点开始,依次回溯到起始点和目标点,形成一条完整的路径。
下面是一个简单的双向RRT算法的Python实现示例:
```python
import numpy as np
class Node:
def __init__(self, point):
self.point = point
self.parent = None
def distance(point1, point2):
return np.linalg.norm(point1 - point2)
def generate_random_point():
# 生成随机点的逻辑
...
def extend_tree(tree, random_point):
nearest_node = None
nearest_distance = float('inf')
for node in tree:
dist = distance(node.point, random_point)
if dist < nearest_distance:
nearest_node = node
nearest_distance = dist
new_point = # 从nearest_node沿着random_point方向生长一段距离得到的新点
new_node = Node(new_point)
new_node.parent = nearest_node
tree.append(new_node)
def connect_trees(tree1, tree2):
# 判断两棵树是否相交的逻辑
...
def rrt(start_point, goal_point, max_iterations):
start_node = Node(start_point)
goal_node = Node(goal_point)
tree1 = [start_node]
tree2 = [goal_node]
for _ in range(max_iterations):
random_point = generate_random_point()
extend_tree(tree1, random_point)
if connect_trees(tree1, tree2):
# 生成路径的逻辑
...
return path
extend_tree(tree2, random_point)
if connect_trees(tree1, tree2):
# 生成路径的逻辑
...
return path
return None
# 使用示例
start_point = np.array([0, 0])
goal_point = np.array([10, 10])
max_iterations = 1000
path = rrt(start_point, goal_point, max_iterations)
if path is not None:
print("找到路径:", path)
else:
print("未找到路径")
```
阅读全文
相关推荐
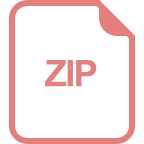
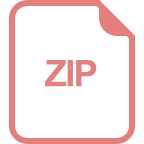
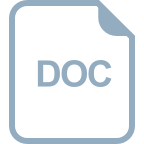
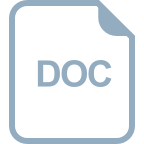
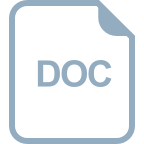


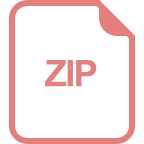
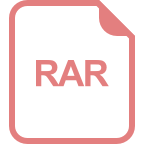
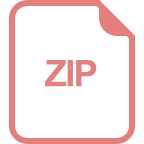

