写一段双向RRT路径规划算法
时间: 2023-06-30 21:26:06 浏览: 117
双向 RRT(Rapidly-exploring Random Tree)是一种基于树搜索的路径规划算法,它能够在高维空间中搜索出一条可行路径。其基本思想是在起点和终点同时构建一颗树,然后从两侧向中间生长,直到两颗树相遇,形成一条路径。下面是一段双向 RRT 路径规划算法的伪代码:
```python
import numpy as np
class RRTNode:
def __init__(self, state):
self.state = state
self.parent = None
class RRT:
def __init__(self, start, goal, step_size, max_iter, obstacle_list):
self.start = RRTNode(start)
self.goal = RRTNode(goal)
self.step_size = step_size
self.max_iter = max_iter
self.obstacle_list = obstacle_list
self.nodes1 = [self.start]
self.nodes2 = [self.goal]
self.path = []
def plan(self):
for i in range(self.max_iter):
q_rand1 = self.sample()
q_near1, idx1 = self.nearest_neighbor(self.nodes1, q_rand1)
q_new1 = self.steer(q_near1, q_rand1, self.step_size)
if self.check_collision(q_near1.state, q_new1.state):
q_new1.parent = idx1
self.nodes1.append(q_new1)
q_rand2 = q_new1
q_near2, idx2 = self.nearest_neighbor(self.nodes2, q_rand2)
q_new2 = self.steer(q_near2, q_rand2, self.step_size)
if self.check_collision(q_near2.state, q_new2.state):
q_new2.parent = idx2
self.nodes2.append(q_new2)
if self.check_goal(q_new1.state, q_new2.state):
self.path = self.generate_path(q_new1, q_new2)
return self.path
return None
def sample(self):
x = np.random.uniform(0, 100)
y = np.random.uniform(0, 100)
return RRTNode((x, y))
def nearest_neighbor(self, nodes, q_rand):
distances = [(node.state[0] - q_rand.state[0])**2 + (node.state[1] - q_rand.state[1])**2 for node in nodes]
idx = np.argmin(distances)
return nodes[idx], idx
def steer(self, q_near, q_rand, step_size):
d = np.sqrt((q_rand.state[0] - q_near.state[0])**2 + (q_rand.state[1] - q_near.state[1])**2)
if d <= step_size:
return q_rand
else:
theta = np.arctan2(q_rand.state[1] - q_near.state[1], q_rand.state[0] - q_near.state[0])
x = q_near.state[0] + step_size * np.cos(theta)
y = q_near.state[1] + step_size * np.sin(theta)
return RRTNode((x, y))
def check_collision(self, q_near, q_new):
for obstacle in self.obstacle_list:
if obstacle.check_collision(q_near, q_new):
return False
return True
def check_goal(self, q_new1, q_new2):
d = np.sqrt((q_new1[0] - q_new2[0])**2 + (q_new1[1] - q_new2[1])**2)
return d < self.step_size
def generate_path(self, q_new1, q_new2):
path1 = [q_new1]
path2 = [q_new2]
node1 = q_new1
node2 = q_new2
while node1.parent is not None:
node1 = self.nodes1[node1.parent]
path1.append(node1)
while node2.parent is not None:
node2 = self.nodes2[node2.parent]
path2.append(node2)
path2.reverse()
return path1 + path2
```
上述代码中,`RRTNode` 类代表树中的一个节点,包含当前状态和父节点信息。`RRT` 类代表整个双向 RRT 算法,包括起点、终点、步长、最大迭代次数、障碍物等信息,并提供了 `plan` 方法用于执行路径规划。在 `plan` 方法中,使用 `sample` 方法随机生成一个状态,然后分别使用 `nearest_neighbor` 方法找到两颗树中距离该状态最近的节点,接着使用 `steer` 方法将树生长到该状态处,并判断该状态是否与障碍物相交。如果两颗树的某个节点相遇,则说明已经找到了一条路径,使用 `generate_path` 方法生成路径信息并返回。在上述代码中,`check_collision` 和 `check_goal` 方法分别用于判断是否与障碍物相交以及是否到达终点。
阅读全文
相关推荐
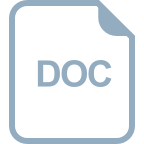
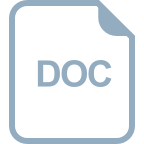
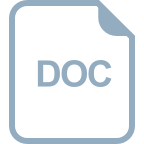

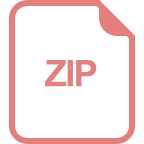



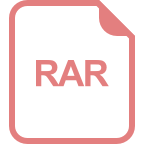
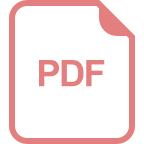
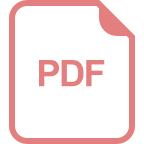
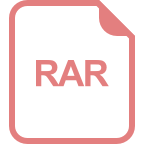
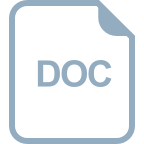
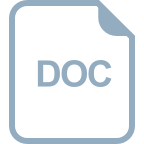
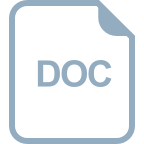
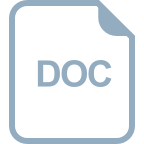

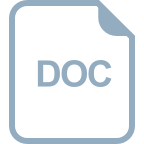
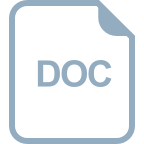